Open Access
ARTICLE
Attentive Neighborhood Feature Augmentation for Semi-supervised Learning
1 School of Computer Science and Cyber Engineering, Guangzhou University, Guangzhou, 510002, China
2 Fujian Provincial Key Laboratory of Information Processing and Intelligent Control, Minjiang University, Fuzhou, 350121, China
* Corresponding Authors: Jing Li. Email: ; Xianmin Wang. Email:
(This article belongs to the Special Issue: AI Powered Human-centric Computing with Cloud/Fog/Edge)
Intelligent Automation & Soft Computing 2023, 37(2), 1753-1771. https://doi.org/10.32604/iasc.2023.039600
Received 07 February 2023; Accepted 14 April 2023; Issue published 21 June 2023
Abstract
Recent state-of-the-art semi-supervised learning (SSL) methods usually use data augmentations as core components. Such methods, however, are limited to simple transformations such as the augmentations under the instance’s naive representations or the augmentations under the instance’s semantic representations. To tackle this problem, we offer a unique insight into data augmentations and propose a novel data-augmentation-based semi-supervised learning method, called Attentive Neighborhood Feature Augmentation (ANFA). The motivation of our method lies in the observation that the relationship between the given feature and its neighborhood may contribute to constructing more reliable transformations for the data, and further facilitating the classifier to distinguish the ambiguous features from the low-dense regions. Specially, we first project the labeled and unlabeled data points into an embedding space and then construct a neighbor graph that serves as a similarity measure based on the similar representations in the embedding space. Then, we employ an attention mechanism to transform the target features into augmented ones based on the neighbor graph. Finally, we formulate a novel semi-supervised loss by encouraging the predictions of the interpolations of augmented features to be consistent with the corresponding interpolations of the predictions of the target features. We carried out experiments on SVHN and CIFAR-10 benchmark datasets and the experimental results demonstrate that our method outperforms the state-of-the-art methods when the number of labeled examples is limited.Keywords
Cite This Article
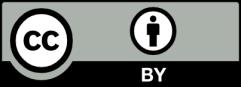