Open Access
ARTICLE
Dart Games Optimizer with Deep Learning-Based Computational Linguistics Named Entity Recognition
1 Department of Computer Science, College of Sciences and Humanities-Aflaj, Prince Sattam bin Abdulaziz University, Al Aflaj, 16828, Saudi Arabia
2 Department of Applied Linguistics, College of Languages, Princess Nourah Bint Abdulrahman University, P. O. Box 84428, Riyadh, 11671, Saudi Arabia
3 Department of Computer Sciences, College of Computing and Information System, Umm Al-Qura University, Makkah, 24211, Saudi Arabia
4 Department of Information Technology, College of Computer and Information Sciences, King Saud University, P. O. Box 145111, Riyadh, 4545, Saudi Arabia
5 Research Centre, Future University in Egypt, New Cairo, 11845, Egypt
6 Department of Computer and Self Development, Preparatory Year Deanship, Prince Sattam bin Abdulaziz University, AlKharj, Saudi Arabia
7 Department of Information System, College of Computer Engineering and Sciences, Prince Sattam bin Abdulaziz University, AlKharj, Saudi Arabia
* Corresponding Author: Mesfer Al Duhayyim. Email:
Intelligent Automation & Soft Computing 2023, 37(3), 2549-2566. https://doi.org/10.32604/iasc.2023.034827
Received 28 July 2022; Accepted 26 October 2022; Issue published 11 September 2023
Abstract
Computational linguistics is an engineering-based scientific discipline. It deals with understanding written and spoken language from a computational viewpoint. Further, the domain also helps construct the artefacts that are useful in processing and producing a language either in bulk or in a dialogue setting. Named Entity Recognition (NER) is a fundamental task in the data extraction process. It concentrates on identifying and labelling the atomic components from several texts grouped under different entities, such as organizations, people, places, and times. Further, the NER mechanism identifies and removes more types of entities as per the requirements. The significance of the NER mechanism has been well-established in Natural Language Processing (NLP) tasks, and various research investigations have been conducted to develop novel NER methods. The conventional ways of managing the tasks range from rule-related and hand-crafted feature-related Machine Learning (ML) techniques to Deep Learning (DL) techniques. In this aspect, the current study introduces a novel Dart Games Optimizer with Hybrid Deep Learning-Driven Computational Linguistics (DGOHDL-CL) model for NER. The presented DGOHDL-CL technique aims to determine and label the atomic components from several texts as a collection of the named entities. In the presented DGOHDL-CL technique, the word embedding process is executed at the initial stage with the help of the word2vec model. For the NER mechanism, the Convolutional Gated Recurrent Unit (CGRU) model is employed in this work. At last, the DGO technique is used as a hyperparameter tuning strategy for the CGRU algorithm to boost the NER’s outcomes. No earlier studies integrated the DGO mechanism with the CGRU model for NER. To exhibit the superiority of the proposed DGOHDL-CL technique, a widespread simulation analysis was executed on two datasets, CoNLL-2003 and OntoNotes 5.0. The experimental outcomes establish the promising performance of the DGOHDL-CL technique over other models.Keywords
Cite This Article
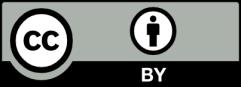