Open Access
ARTICLE
Dynamic Security SFC Branching Path Selection Using Deep Reinforcement Learning
School of Electronic and Information Engineering, Beijing Jiaotong University, Beijing, 100044, China
* Corresponding Author: Man Li. Email:
(This article belongs to the Special Issue: Advanced Achievements of Intelligent and Secure Systems for the Next Generation Computing)
Intelligent Automation & Soft Computing 2023, 37(3), 2919-2939. https://doi.org/10.32604/iasc.2023.039985
Received 27 February 2023; Accepted 12 June 2023; Issue published 11 September 2023
Abstract
Security service function chaining (SFC) based on software-defined networking (SDN) and network function virtualization (NFV) technology allows traffic to be forwarded sequentially among different security service functions to achieve a combination of security functions. Security SFC can be deployed according to requirements, but the current SFC is not flexible enough and lacks an effective feedback mechanism. The SFC is not traffic aware and the changes of traffic may cause the previously deployed security SFC to be invalid. How to establish a closed-loop mechanism to enhance the adaptive capability of the security SFC to malicious traffic has become an important issue. Our contribution is threefold. First, we propose a secure SFC path selection framework. The framework can accept the feedback results of traffic and security service functions in SFC, and dynamically select the optimal path for SFC based on the feedback results. It also realizes the automatic deployment of paths, forming a complete closed loop. Second, we expand the protocol of SFC to realize the security SFC with branching path, which improve flexibility of security SFC. Third, we propose a deep reinforcement learning-based dynamic path selection method for security SFC. It infers the optimal branching path by analyzing feedback from the security SFC. We have experimented with Distributed Denial of Service (DDoS) attack detection modules as security service functions. Experimental results show that our proposed method can dynamically select the optimal branching path for a security SFC based on traffic features and the state of the SFC. And it improves the accuracy of the overall malicious traffic detection of the security SFC and significantly reduces the latency and overall load of the SFC.Keywords
Cite This Article
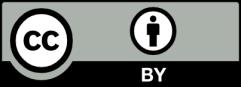