Open Access
ARTICLE
State Accurate Representation and Performance Prediction Algorithm Optimization for Industrial Equipment Based on Digital Twin
1 School of Artificial Intelligence and Big Data, Hefei University, Hefei, 230601, China
2 Anhui Imagine Industrial Technology Co., Ltd., Hefei, 230088, China
* Corresponding Author: Ying Bai. Email:
(This article belongs to the Special Issue: Innovation in Mechatronics Approaches for Physical Human-Robot Interaction and Co-Manipulation)
Intelligent Automation & Soft Computing 2023, 37(3), 2999-3018. https://doi.org/10.32604/iasc.2023.040124
Received 06 March 2023; Accepted 12 June 2023; Issue published 11 September 2023
Abstract
The combination of the Industrial Internet of Things (IIoT) and digital twin (DT) technology makes it possible for the DT model to realize the dynamic perception of equipment status and performance. However, conventional digital modeling is weak in the fusion and adjustment ability between virtual and real information. The performance prediction based on experience greatly reduces the inclusiveness and accuracy of the model. In this paper, a DT-IIoT optimization model is proposed to improve the real-time representation and prediction ability of the key equipment state. Firstly, a global real-time feedback and the dynamic adjustment mechanism is established by combining DT-IIoT with algorithm optimization. Secondly, a strong screening dual-model optimization (SSDO) prediction method based on Stacking integration and fusion is proposed in the dynamic regulation mechanism. Lightweight screening and multi-round optimization are used to improve the prediction accuracy of the evolution model. Finally, taking the boiler performance of a power plant in Shanxi as an example, the accurate representation and evolution prediction of boiler steam quantity is realized. The results show that the real-time state representation and life cycle performance prediction of large key equipment is optimized through these methods. The self-lifting ability of the Stacking integration and fusion-based SSDO prediction method is 15.85% on average, and the optimal self-lifting ability is 18.16%. The optimization model reduces the MSE loss from the initial 0.318 to the optimal 0.1074, and increases from the initial 0.731 to the optimal 0.9092. The adaptability and reliability of the model are comprehensively improved, and better prediction and analysis results are achieved. This ensures the stable operation of core equipment, and is of great significance to comprehensively understanding the equipment status and performance.Keywords
Cite This Article
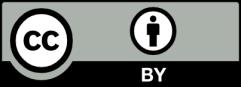