Open Access
ARTICLE
Reconstruction and Optimization of Complex Network Community Structure under Deep Learning and Quantum Ant Colony Optimization Algorithm
1 Digital Performance and Simulation Technology, School of Computer Science & Technology, Beijing Institute of Technology, Beijing, 100081, China
2 Fujian Provincial Key Laboratory of Information Processing and Intelligent Control, Minjiang University, Fuzhou, 350117, China
3 Department of Computer Science, University of California, Davis, CA 95616, USA
* Corresponding Author: Fuquan Zhang. Email:
Intelligent Automation & Soft Computing 2021, 27(1), 159-171. https://doi.org/10.32604/iasc.2021.012813
Received 13 July 2020; Accepted 16 August 2020; Issue published 07 January 2021
Abstract
Community structure is a key component in complex network systems. This paper aims to improve the effectiveness of community detection and community discovery in complex network systems by providing directions for the reconstruction and optimization of community structures to expand the application of intelligent optimization algorithms in community structures. First, deep learning algorithms and ant colony algorithms are used to elaborate the community detection and community discovery in complex networks. Next, we introduce the technology of transfer learning and propose an algorithm of deep self-encoder modeling based on transfer learning (DSEM-TL). The DSEM-TL algorithm’s indicators include normalized mutual information and modularity. Finally, an algorithm that combines the ant colony optimization (ACO) algorithm and the quantum update strategy, called QACO, is proposed. The proposed community structure reconstruction scheme is compared with other methods using the accuracy rate as the indicator. The results show that the DSEM-TL algorithm exhibits the optimal detection rate, better applicability, and higher effectiveness in real networks. Under the given the condition that the number of edges between communities Zout is >6, DSEM-TL shows better performance on the Girvan–Newman benchmark network than several other community discovery algorithms. Furthermore, under the given condition that the mixed parameter μ is >0.65, the DSEM-TL algorithm outperforms several other algorithms on the Lancichinetti–Fortunato–Radicchi benchmark network. When given μ < 0.4, the QACO algorithm can determine the proper division of the corresponding network. When the case is μ > 0.45, the division result corresponding to the QACO algorithm is closer to the real community division, which has a faster convergence speed and better convergence performances. Consequently, the proposed community structure reconstruction scheme has higher accuracy. The proposed two intelligent optimization algorithms have potential application in the reconstruction and optimization of community structure.Keywords
Cite This Article
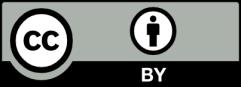