Open Access
ARTICLE
Real-Time Spammers Detection Based on Metadata Features with Machine Learning
1 School of Computer Science and Technology, University of Science and Technology of China, Hefei, 230026, China
2 School of Information and Communication Engineering, Hainan University, Haikou, 570228, China
3 Metaverse Research Institute, School of Computer Science and Cyber Engineering, Guangzhou University, Guangzhou, 510006, China
* Corresponding Author: Asad Khan. Email:
(This article belongs to the Special Issue: Deep Learning for Multimedia Processing)
Intelligent Automation & Soft Computing 2023, 38(3), 241-258. https://doi.org/10.32604/iasc.2023.041645
Received 30 April 2023; Accepted 10 July 2023; Issue published 27 February 2024
Abstract
Spammer detection is to identify and block malicious activities performing users. Such users should be identified and terminated from social media to keep the social media process organic and to maintain the integrity of online social spaces. Previous research aimed to find spammers based on hybrid approaches of graph mining, posted content, and metadata, using small and manually labeled datasets. However, such hybrid approaches are unscalable, not robust, particular dataset dependent, and require numerous parameters, complex graphs, and natural language processing (NLP) resources to make decisions, which makes spammer detection impractical for real-time detection. For example, graph mining requires neighbors’ information, posted content-based approaches require multiple tweets from user profiles, then NLP resources to make decisions that are not applicable in a real-time environment. To fill the gap, firstly, we propose a REal-time Metadata based Spammer detection (REMS) model based on only metadata features to identify spammers, which takes the least number of parameters and provides adequate results. REMS is a scalable and robust model that uses only 19 metadata features of Twitter users to induce 73.81% F1-Score classification accuracy using a balanced training dataset (50% spam and 50% genuine users). The 19 features are 8 original and 11 derived features from the original features of Twitter users, identified with extensive experiments and analysis. Secondly, we present the largest and most diverse dataset of published research, comprising 211 K spam users and 1 million genuine users. The diversity of the dataset can be measured as it comprises users who posted 2.1 million Tweets on seven topics (100 hashtags) from 6 different geographical locations. The REMS’s superior classification performance with multiple machine and deep learning methods indicates that only metadata features have the potential to identify spammers rather than focusing on volatile posted content and complex graph structures. Dataset and REMS’s codes are available on GitHub ().Keywords
Cite This Article
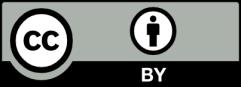