Open Access
PROCEEDINGS
Efficient and Robust Temperature Field Simulation of Long-Distance Crude Oil Pipeline Based on Bayesian Neural Network and PDE
1 Faculty of Environment and Life, Beijing University of Technology, Beijing, 100124, China
2 School of Chemical Engineering and Technology, Xi’an Jiaotong University, Xi’an, 710049, China
3 State Key Laboratory of Multiphase Flow in Power Engineering, Xi’an Jiaotong University, Xi’an, 710049, China
4 School of Mechanical Engineering, Beijing Institute of Petrochemical Technology4., Beijing, 102617, China
* Corresponding Author: Weixin Jiang. Email:
The International Conference on Computational & Experimental Engineering and Sciences 2023, 25(4), 1-2. https://doi.org/10.32604/icces.2023.08861
Abstract
The hydraulic and thermal simulation of crude oil pipeline transportation is greatly significant for the safe transportation and accurate regulation of pipelines. With reasonable basic parameters, the solution of the traditional partial differential equation (PDE) for the axial soil temperature field on the pipeline can obtain accurate simulation results, yet it brings about a low calculation efficiency problem. In order to overcome the low-efficiency problem, an efficient and robust hybrid solution model for soil temperature field coupling with Bayesian neural network and PDE is proposed, which considers the dynamic changes of boundary conditions. Four models, including the proposed hybrid model, PDE, and two kinds of neural networks (NNs), are adopted to predict the single soil temperature field and transient oil temperature at the outlet of the pipeline. It is found that the prediction results of the hybrid model are closer to those of PDE than those of NNs, and the hybrid model can obtain more stable and reliable prediction results of transient oil temperature than NNs on the basic training data sets with different quantities and boundary conditions. On the other hand, the calculation time of the hybrid method is far less than that of PDE, and the hybrid model is applicable for the situation of small training data to improve the simulation efficiency. This study can provide a scientific reference for the fast real-time simulation of crude oil pipelines.Keywords
Cite This Article
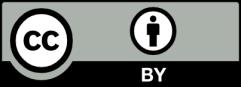