Open Access
ARTICLE
Clustering Algorithms: Taxonomy, Comparison, and Empirical Analysis in 2D Datasets
1 Computer Science-Mathematics Department, Faculty of Science, South Valley University, Qena, 83523, Egypt
2 Fellow of the Academy of Scientific Research and Technology (ASRT), Egypt
* Corresponding Author: Samih M. Mostafa. Email:
Journal on Artificial Intelligence 2020, 2(4), 189-215. https://doi.org/10.32604/jai.2020.014944
Received 29 October 2020; Accepted 03 December 2020; Issue published 31 December 2020
Abstract
Because of the abundance of clustering methods, comparing between methods and determining which method is proper for a given dataset is crucial. Especially, the availability of huge experimental datasets and transactional and the emerging requirements for data mining and the like needs badly for clustering algorithms that can be applied in various domains. This paper presents essential notions of clustering and offers an overview of the significant features of the most common representative clustering algorithms of clustering categories presented in a comparative way. More specifically the study is based on the numerical type of the data that the algorithm supports, the shape of the clusters, and complexity. The experiments were done using nine clustering algorithms representing the common clustering categories on eight 2D clustered datasets differ in the clusters’ shapes and density of the data points. Furthermore, the comparison was done from the point of view seven performance measures.Keywords
Cite This Article
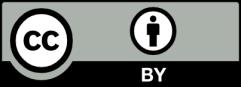