Open Access
ARTICLE
Churn Prediction Model of Telecom Users Based on XGBoost
Beijing University of Posts and Telecommunications, Beijing, 100876, China
* Corresponding Author: Hao Chen. Email:
Journal on Artificial Intelligence 2021, 3(3), 115-121. https://doi.org/10.32604/jai.2021.026851
Received 05 January 2022; Accepted 10 January 2022; Issue published 25 January 2022
Abstract
As the cost of accessing a telecom operator’s network continues to decrease, user churn after arrears occurred repeatedly, which has brought huge economic losses to operators and reminded them that it is significant to identify users who are likely to churn in advance. Machine learning can form a series of judgment rules by summarizing a large amount of data, and telecom user data naturally has the advantage of user scale, which can provide data support for learning algorithms. XGBoost is an improved gradient boosting algorithm, and in this paper, we explore how to use the algorithm to train an efficient model and use this model one month in advance to predict whether users will churn. Our work is mainly divided into two aspects: (1) By completing data exploration, feature engineering and data preprocessing, we obtained a data set that can be used to train a prediction model and features that can effectively predict user churn. And using these features and data sets, two prediction models were trained based on Random Forest and XGBoost. (2) According to the business needs of telecom operators, we continuously evaluated and optimized these models. And by comparing the test results of the two models, we proved that the XGBoost model performs better for the precision and recall of user churn.Keywords
Cite This Article
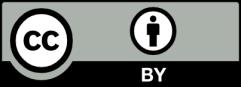