Open Access
ARTICLE
A Framework of Lightweight Deep Cross-Connected Convolution Kernel Mapping Support Vector Machines
1 Beijing University of Technology, Beijing, 100124, China
2 Guilin University of Electronic Technology, Guilin, 541004, China
3 School of Engineering, Edith Cowan University, Perth, WA 6027, Australia
* Corresponding Author: Ting Zhang. Email:
Journal on Artificial Intelligence 2022, 4(1), 37-48. https://doi.org/10.32604/jai.2022.027875
Received 28 January 2022; Accepted 15 March 2022; Issue published 16 May 2022
Abstract
Deep kernel mapping support vector machines have achieved good results in numerous tasks by mapping features from a low-dimensional space to a high-dimensional space and then using support vector machines for classification. However, the depth kernel mapping support vector machine does not take into account the connection of different dimensional spaces and increases the model parameters. To further improve the recognition capability of deep kernel mapping support vector machines while reducing the number of model parameters, this paper proposes a framework of Lightweight Deep Convolutional Cross-Connected Kernel Mapping Support Vector Machines (LC-CKMSVM). The framework consists of a feature extraction module and a classification module. The feature extraction module first maps the data from low-dimensional to high-dimensional space by fusing the representations of different dimensional spaces through cross-connections; then, it uses depthwise separable convolution to replace part of the original convolution to reduce the number of parameters in the module; The classification module uses a soft margin support vector machine for classification. The results on 6 different visual datasets show that LC-CKMSVM obtains better classification accuracies on most cases than the other five models.Keywords
Cite This Article
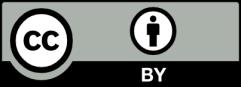
This work is licensed under a Creative Commons Attribution 4.0 International License , which permits unrestricted use, distribution, and reproduction in any medium, provided the original work is properly cited.