Open Access
REVIEW
Embracing the Future: AI and ML Transforming Urban Environments in Smart Cities
Chitkara Business School, Chitkara University, Rajpura, Punjab, 140401, India
* Corresponding Author: Gagan Deep. Email:
(This article belongs to the Special Issue: Explainable & Responsible Edge-AI for Smart Computing Technologies )
Journal on Artificial Intelligence 2023, 5, 57-73. https://doi.org/10.32604/jai.2023.043329
Received 29 June 2023; Accepted 28 July 2023; Issue published 22 September 2023
Abstract
This research explores the increasing importance of Artificial Intelligence (AI) and Machine Learning (ML) with relation to smart cities. It discusses the AI and ML’s ability to revolutionize various aspects of urban environments, including infrastructure, governance, public safety, and sustainability. The research presents the definition and characteristics of smart cities, highlighting the key components and technologies driving initiatives for smart cities. The methodology employed in this study involved a comprehensive review of relevant literature, research papers, and reports on the subject of AI and ML in smart cities. Various sources were consulted to gather information on the integration of AI and ML technologies in various aspects of smart cities, including infrastructure optimization, public safety enhancement, and citizen services improvement. The findings suggest that AI and ML technologies enable data-driven decision-making, predictive analytics, and optimization in smart city development. They are vital to the development of transport infrastructure, optimizing energy distribution, improving public safety, streamlining governance, and transforming healthcare services. However, ethical and privacy considerations, as well as technical challenges, need to be solved to guarantee the ethical and responsible usage of AI and ML in smart cities. The study concludes by discussing the challenges and future directions of AI and ML in shaping urban environments, highlighting the importance of collaborative efforts and responsible implementation. The findings highlight the transformative potential of AI and ML in optimizing resource utilization, enhancing citizen services, and creating more sustainable and resilient smart cities. Future studies should concentrate on addressing technical limitations, creating robust policy frameworks, and fostering fairness, accountability, and openness in the use of AI and ML technologies in smart cities.Keywords
In recent years, there has been a lot of interest in the creation of smart cities, driven by advancements in technology and the need for sustainable and efficient urban environments. AI and ML have emerged as crucial technologies in relation to smart cities, offering transformative solutions and opportunities for urban evolution. These technologies enable the analysis of large volumes of data, predictive analytics, and optimization, enhancing different characteristics of urban lives, such as managing infrastructure, general population safety, governance, and citizen services [1].
Despite the increasing importance of AI and ML in smart cities, there are obstacles and factors to take into account for their effective execution and responsible use. These challenges include technical limitations, ethical concerns, privacy issues, and the need for robust policy frameworks. Additionally, the scope and potential applications of AI and ML in smart cities need to be explored to understand the extent to which these technologies can revolutionize urban environments.
This research aims to address the opportunities and difficulties of integrating AI and ML in smart cities. The scope of this study encompasses the following areas:
1. Understanding the AI and ML’s function in optimizing urban infrastructure, including transportation systems, energy distribution, and waste management.
2. Exploring the use of ML and AI in enhancing public safety and security, including intelligent surveillance, crime prediction, and cybersecurity.
3. Examining the use of AI and ML in smart governance and citizen services, such as personalized interactions, data-driven making decisions and providing services effectively.
4. Examining the possibility of AI and ML in transforming healthcare services, including remote patient monitoring, disease outbreak detection, and telemedicine.
5. Addressing ethical and privacy considerations associated with application of AI and ML in smart cities, including equity, transparency, and citizen data protection.
1.3 Overview of the Increasing Importance of AI and ML in the Context of Smart Cities
AI and ML are playing an essential part in the development and advancement of smart cities. The integration of AI and ML technologies with relation to smart cities offers transformative solutions and opportunities for urban environments. AI and ML are enabling examination of large volumes of information collected from various sources in smart cities, leading to data-driven decision-making and improved urban management. According to a study by [2], AI and ML techniques are essential for energy management and optimization in smart cities, resulting in significant energy savings and environmental benefits. These technologies allow for the processing of real-time data from transportation networks, optimizing traffic flow, reducing congestion, and enhancing overall transportation efficiency [3]. Predictive analytics and anomaly detection powered by ML algorithms play a crucial role in smart cities. ML models can analyze patterns and historical data to identify trends, make accurate predictions, and optimize resource allocation in areas such as garbage management, energy use, and safety for people [4]. For example, predictive analytics can be used to predict traffic jams and adjust the timing of the traffic signals accordingly [5]. Furthermore, AI and ML technologies facilitate citizen engagement and personalized services in smart cities. Chatbots and virtual assistants powered by AI provide interactive interfaces for citizens to access information, report issues, and receive personalized recommendations, enhancing the overall citizen experience [6]. These technologies enable smart cities to provide efficient and customized services to their residents.
1.4 The Potential of AI and ML to Revolutionize Urban Environments
AI and ML technologies hold tremendous potential to revolutionize urban environments, enabling transformative advancements in various aspects of city life as given below:
1. Enhancing Urban Infrastructure: AI and ML technologies have the capacity to optimize urban infrastructure through enhancing effectiveness, sustainability, and resource management. ML algorithms can analyze data from diverse sources to optimize energy consumption in smart grids, reducing costs and environmental impact [2]. Furthermore, AI techniques can be applied to intelligent transportation systems, optimizing traffic flow, reducing congestion, and enhancing overall mobility [5].
2. Enabling Smart Governance and Services: There is potential for AI and ML to transform governance and public services in urban environments. Intelligent systems powered by AI can improve service delivery, citizen engagement, and decision-making processes. Chatbots and virtual assistants, for example, can provide personalized and efficient services to citizens, addressing their queries and needs [6]. Large data sets may be analysed by AI algorithms to aid in data-driven decision-making in fields like urban planning and policy formulation [7].
3. Advancing Public Safety and Security: AI and ML technologies offer significant potential to improve the security and safety of the public in urban environments as indicated by Table 1. Intelligent methods of monitoring powered by AI algorithms can analyze video feeds and detect potential threats or suspicious activities in real-time [8]. ML models can be trained to predict crime patterns and assist law enforcement agencies in proactive crime prevention [9].
4. Fostering Sustainable and Resilient Cities: AI and ML can contribute to creating sustainable and resilient cities. Sensor data may be analysed by ML algorithms, climatic trends, and historical records to support better environmental management and disaster response [10]. By leveraging AI and ML, cities can optimize waste management systems, reducing environmental impact and improving resource utilization [3].
The research begins with an introduction to smart cities, providing an overview of their defining features and the pivotal role of AI and ML in their development. It then delves into the ways AI and ML can enhance urban infrastructure, optimizing transportation systems, energy grids, waste management, and urban planning. The discussion also encompasses how AI and ML contribute to public safety and security, through intelligent surveillance, predictive analytics, and cybersecurity measures. Furthermore, the research explores the uses for AI and ML in smart governance and citizen services, enabling citizen engagement, virtual assistance, and decision-making based on data. The blending of AI and ML in smart healthcare is also examined, focusing on remote patient monitoring, disease outbreak detection, and improved healthcare access. Ethical and privacy considerations are highlighted, emphasizing the responsible application of ML and AI in smart cities while safeguarding citizen privacy and addressing biases. The research concludes with a discussion on the challenges and future directions of AI and ML in smart cities, including technical limitations, policy frameworks, and emerging trends.
2.1 Definition and Characteristics of Smart Cities
Researchers and organisations have used numerous definitions to describe smart cities. Reference [11] defined smart cities as “innovative cities that use information and communication technologies (ICT) and other means to improve the quality of life, efficiency of urban operations and services, and competitiveness while ensuring that it meets the needs of present and future generations.” This concept emphasizes the use of ICT as a fundamental element in making cities smarter. The following are the characteristics of smart cities as indicated by Fig. 1.
Figure 1: Interconnectedness of the different characteristics of smart cities
Source: Author’s own compilation.
(a) ICT Infrastructure: Smart cities are characterized by robust and interconnected information and communication technology (ICT) infrastructure that enables data gathering, analysis, and sharing to improve urban operations and services [12].
(b) Data-Driven Decision-Making: To enable data-driven decision-making, smart cities rely on the gathering and analysis of enormous volumes of data from numerous sources. This information consists of data from sensors, devices, and systems deployed throughout the city [13].
(c) Sustainability and Efficiency: Smart cities prioritize sustainable practices, aiming to optimize resource utilization, reduce environmental impact, and enhance energy efficiency [14].
(d) Citizen-Centric Approach: Smart cities focus on enhancing the standard of living for residents by offering them with improved facilities, increased citizen engagement, and a better overall urban experience [15].
(e) Collaborative Governance: Smart cities promote collaboration and partnerships between several parties, including government agencies and businesses, academic institutions, and residents, to co-create and implement innovative solutions [16].
2.2 Key Components and Technologies Driving Smart City Initiatives
Smart city initiatives are driven by various key components and technologies that enable the transformation of urban environments as follows:
1. Smart cities are undergoing an IoT revolution by facilitating connectivity and real-time data exchange among various physical devices and sensors [17]. IoT is significantly contributing to several key aspects of smart cities, including transportation systems, energy grids, and waste management. IoT is playing a vital role in optimizing transportation systems in smart cities. By deploying IoT-enabled sensors and devices, cities may collect statistics on traffic movement in real-time, congestion levels, and availability of parking [17]. Such information enables the implementation of data-driven traffic management strategies, resulting in less traffic and more efficient transit. In energy management, IoT is enabling development of smart grids that optimize energy distribution and consumption. IoT sensors deployed in energy grids give current information on energy use trends, granting utilities to identify inefficiencies & allocate energy more efficiently [17]. This results in improved demand response management and reduced energy wastage. Waste management is another area benefiting from IoT in smart cities. IoT sensors in garbage bins track the level of fill and transmit data to waste management systems [17]. This data enables optimized waste collection routes and schedules, leading to enhanced resource utilization and cost reduction. By leveraging IoT technologies, smart cities are adopting a citizen-centric approach, focusing on improving the standard of living for locals. IoT-enabled devices and applications empower citizens to actively engage with urban services and provide real-time feedback [15]. This citizen engagement contributes to the overall success of smart city initiatives. Moreover, IoT fosters collaboration between different stakeholders in smart cities. Government agencies, private sector entities, academic institutions, and citizens come together to co-create innovative solutions [16]. This collaborative governance approach ensures that initiatives related to smart cities meet community needs and ambitions.
2. Big Data and Analytics play a pivotal role in driving the success of smart cities by enabling making decisions based on data and extracting valuable ideas from diverse data sources [18,19]. They contribute to various aspects of smart cities, including resource allocation, service delivery, and urban planning. One significant contribution of Big Data and Analytics is optimizing resource allocation in smart cities. Through analysis of an enormous quantity of data based on sensors and tools, cities may benefit insights into resource utilization patterns, leading to more efficient allocation of resources such as energy, water, and transportation services [11,18]. This optimization enhances sustainability, reduces costs, and improves resource management. Big Data and Analytics also enhance service delivery in smart cities. By analyzing data on citizen behavior, preferences, and feedback, cities can tailor services to meet specific needs. For example, analytics can optimize public transportation routes and schedules based on demand patterns, leading to improved service reliability, reduced congestion, and enhanced mobility [13,18]. In terms of urban planning, Big Data and Analytics provide valuable insights for informed decision-making. Using statistics on population density and transportation flow, environmental factors, & social dynamics, cities are able to data-driven decisions regarding infrastructure development, land use, and zoning regulations [14,18]. This supports more effective urban planning, sustainable growth, and improved quality of life for residents. Furthermore, Big Data and Analytics foster innovation and collaboration in smart cities. By sharing data with researchers, entrepreneurs, and developers, cities create chances for the creation of cutting-edge services and applications. This collaboration drives the creation of solutions that address urban challenges, improve efficiency, and enhance citizen engagement [15,18].
3. Cloud computing offers a significant function in contributing to success of smart cities by providing scalable and cost-efficient infrastructure for data storage, processing, and analysis [20,21]. It offers several key contributions to smart cities, including enhanced data management, improved service delivery, and increased collaboration and innovation. One of the primary contributions of cloud computing to smart cities is in the domain of data management. Numerous sources, including sensors, gadgets, and social media platforms, produce a tonne of data in smart cities. Cloud computing provides the necessary storage capacity and processing capabilities to handle and analyze this data efficiently [20]. By utilizing cloud-based platforms, cities can securely store and manage large volumes of data, ensuring its availability for analysis and decision-making processes [21]. Cloud computing also enables improved service delivery in smart cities. By leveraging cloud-based infrastructure, cities can deploy and scale services rapidly, ensuring reliable and on-demand access to critical applications and resources for both citizens and city administrators [20,21]. This enhances service availability, responsiveness, and agility, ultimately leading to a better overall urban experience. Furthermore, cloud computing fosters collaboration and innovation in smart cities. By providing a shared and flexible computing environment, cloud platforms enable different stakeholders, such as government agencies, businesses, and researchers, to collaborate and develop innovative solutions [20]. This collaboration leads to the development of new applications, business concepts, services that address urban challenges and promote sustainable development. Moreover, cloud computing offers cost-efficiency for smart cities. Cities no longer need to make significant investments in on-premises infrastructure and allows them to leverage computing resources on-demand, paying only for the resources they consume [21]. This scalability and cost-effective approach enable cities with limited budgets to access advanced computing capabilities and leverage cutting-edge technologies without significant upfront investments.
4. AI and ML are playing a vital part in shaping the progression and success of smart cities [22]. These technologies contribute to smart cities by enabling advanced analytics, prediction, and automation, leading to improved decision-making and resource optimization [23]. Numerous data sets are analysed by AI and ML systems generated by smart city infrastructure and systems. They can identify patterns, detect anomalies, and make predictions based on historical data, enabling cities to gain valuable insights for optimizing various aspects of urban life [23]. For instance, in transportation, Real-time traffic data may be analysed by AI and ML systems to improve traffic flow, reduce congestion, and improve transportation efficiency [24]. These technologies also contribute to energy management in smart cities. In order to optimise energy distribution and decrease waste, machine learning algorithms may analyse patterns of energy usage [23]. This leads to significant energy savings and improved sustainability. Furthermore, AI and ML technologies enhance public safety by enabling intelligent surveillance systems. These technologies are able to spot unusual behaviours, assess possible security risks, and enable quick action [23,25]. Such capabilities enhance situational awareness and support proactive measures to ensure the safety and security of citizens.
5. Sensor Networks: Sensor networks are critical components in smart city initiatives as they enable the collection of real-time data from various urban systems and environments. These sensors can measure parameters like air quality, traffic flow, noise and trash production levels, providing valuable data for monitoring and management [26].
6. Connectivity and Communication Infrastructure: Robust connectivity and communication infrastructure are essential for smart city initiatives. High-speed broadband networks, Wi-Fi hotspots, and communication protocols enable the seamless exchange of data between devices, systems, and stakeholders. This facilitates efficient data flow, real-time monitoring, and effective communication [27].
2.3 The Role of AI and ML in Smart City Development
A key role is played by AI and ML in driving smart city development, offering transformative solutions to enhance urban environments as follows:
1. Data Analytics and Decision-Making: AI and ML techniques enable advanced analytics of data and decision-making processes in smart city development. These technologies can analyze vast amounts of data collected from a variety of sources, including sensors and tools, to extract valuable insights and patterns [12]. Algorithms using ML and AI can process real-time data and generate actionable recommendations to optimize resource allocation, improve services, and enhance urban planning [23].
2. Predictive Analytics and Optimization: AI and ML are utilized in smart cities for predictive analytics and optimization. To predict trends and patterns, ML systems may examine both historical and current data, enabling cities to anticipate and address challenges proactively [28]. Predictive analytics can be applied to areas such as traffic management, energy consumption, and waste management to optimize resource allocation and improve operational efficiency [2].
3. Intelligent Transportation Systems: AI and ML technologies are crucial in developing intelligent transportation systems in smart cities. These technologies enable real-time traffic monitoring, congestion prediction, and adaptive traffic signal control, leading to improved traffic flow and reduced congestion [29]. ML models can analyze traffic patterns and historical data to optimize transportation routes, enhance public transportation systems, and enable efficient mobility services [30].
4. Protection of the General Public: In smart cities, AI and ML significantly improve public safety and security. Systems for savvy surveillance powered by AI algorithms can analyze video feeds and detect anomalies or suspicious activities in real-time [31]. ML models can process vast amounts of data from diverse sources to detect patterns related to crime, enabling law enforcement agencies to prevent and respond to incidents effectively [9].
5. Smart Energy Management: AI and ML are instrumental in optimizing energy management and promoting sustainability in smart cities. ML algorithms can examine patterns of energy usage and improve energy allocation, leading to more efficient use of resources and reduced environmental impact [32]. AI-powered systems can adaptively manage energy demand, predict peak loads, and the system should incorporate renewable energy sources [33].
3 Enhancing Urban Infrastructure with AI and ML
3.1 Traffic Control and Smart Transport Systems
In order to improve the effectiveness, safety, and sustainability of transportation systems, intelligent transportation systems (ITS) refers to the integration of cutting-edge technology, such as sensors, communication networks, and data analytics, into the transportation infrastructure [34]. ITS aims to provide intelligent solutions for traffic management, traveler information, vehicle operations, and transportation infrastructure. Intelligent transportation systems utilize various sensors and technologies to monitor traffic conditions and collect real-time data. These sensors can include loop detectors, video cameras, and GPS devices [35]. Data collected from these sensors, such as traffic volume, speed, and congestion patterns, are crucial for effective traffic management and decision-making. In order to forecast traffic patterns and congestion, advanced traffic management approaches use AI and ML algorithms to analyse historical and real-time traffic data. These predictions help in proactive congestion management and enable authorities to implement effective traffic control strategies, such as adaptive signal control systems [36]. ML models can also optimize traffic signal timings to minimize delays and improve traffic flow [37]. AI and optimisation algorithms are used by intelligent traffic signal control systems to dynamically change signal timings based on current traffic circumstances as indicated by Table 1. These programmes enhance travel times, optimise traffic flow, and lessen congestion [38]. ML techniques can be applied to learn traffic patterns and adapt signal control strategies to changing traffic demands [36]. Communication between automobiles, infrastructure, and traffic management systems is made possible through the incorporation of connected vehicle technology. This connectivity allows for cooperative systems that exchange real-time data, such as vehicle speed, location, and traffic conditions [39]. Cooperative systems facilitate enhanced traffic management, collision avoidance, and improved traveler information.
3.2 Optimising the Usage and Distribution of Energy in Smart Networks
To achieve effective and sustainable energy management, it is essential to optimise energy distribution and consumption in smart networks. Bidirectional energy flow and real-time monitoring are made possible by smart grids, which incorporate renewable energy sources, energy storage systems, and demand-side management. The implementation of smart meters as part of the Advanced Metering Infrastructure (AMI) allows for detailed energy consumption monitoring [40]. Demand response initiatives further encourage customers to modify their energy use habits during periods of high demand, which results in load shifting and lessens grid stress [41]. Incorporating DERs, such as solar panels and wind turbines, into the energy grid enables the generation of renewable energy and supports local energy production. AI and ML algorithms contribute a vital funtion in optimizing energy management and distribution, enabling load forecasting, energy scheduling, and power flow optimization [42]. ML models can analyze real-time data, predict energy demand, and dynamically adjust energy distribution and generation to optimize efficiency [43]. By incorporating these technologies, smart grids contribute to reduced energy waste, improved grid stability, and increased utilization of renewable energy sources, ultimately promoting sustainable energy management.
3.3 Smart Waste Management and Environmental Sustainability
Smart waste management systems are instrumental in promoting environmental sustainability by optimizing waste collection, reducing pollution, and maximizing resource utilization as indicated by Table 1. These systems leverage advanced technologies such as Internet of Things (IoT), sensors, and data analytics to enhance waste management processes. Smart bins with sensors that are IoT-enabled can track garbage levels, enabling effective collection routes and reducing needless visits [44]. Data gathering and analysis in real-time enable waste management authorities to identify high-volume areas, optimize collection schedules, and allocate resources more effectively. Furthermore, ML algorithms may examine both historical and current data to predict waste generation designs, facilitating proactive forecasting and resource allocation [45]. Smart waste management systems also promote recycling and waste segregation through the implementation of intelligent sorting techniques. For instance, different sorts of garbage may be recognised using AI-powered picture recognition systems, which can then be categorised [46]. This enables more efficient recycling processes and reduces the environmental impact of waste disposal. By implementing smart waste management systems, cities can achieve significant improvements in environmental sustainability, waste reduction, and resource conservation, leading to a cleaner and greener future.
3.4 The Development of Infrastructure and Better Urban Planning
Improving urban planning and infrastructure development is essential for creating sustainable and livable cities. Technologically sophisticated tools like geographic information systems (GIS), remote sensing, and data analytics, play a crucial role in enhancing urban planning processes. GIS enables the integration and analysis of geospatial data, facilitating informed decision-making for urban development [47]. Remote sensing technologies, such as satellite imagery and aerial photography, provide valuable information for monitoring land use, vegetation, and changes in urban environments [30]. These technologies contribute to effective urban planning by identifying areas for development, assessing environmental impacts, and supporting infrastructure design. Additionally, data analytics and ML algorithms enable the analysis and processing of large-scale urban data, supporting evidence-based decision-making [23]. For instance, ML models can predict population growth patterns, traffic flows, and energy demands, aiding in infrastructure planning and resource allocation [25]. By leveraging these technologies, cities can optimize land use, transportation networks, and environmental management, resulting in urban landscapes that are more resilient and sustainable.
4 AI and ML for Public Safety and Security
4.1 Intelligent Surveillance and Monitoring Systems
AI and ML algorithms enable the development of intelligent surveillance and monitoring systems. These systems can analyze video feeds, detect anomalies, and identify potential security threats in real-time [31]. For example, AI-based video analytics can detect abnormal behavior, unauthorized access, or suspicious activities, enhancing situational awareness and enabling proactive security measures [31].
4.2 Predictive Analytics for Crime Prevention and Emergency Response
AI and ML techniques are utilized in predictive analytics for crime prevention and emergency response. These technologies analyze large volumes of historical crime data, social media feeds, and other relevant sources to identify patterns and predict potential crime hotspots [9]. ML models can assist law enforcement agencies in allocating resources, optimizing patrol routes, and developing proactive crime prevention strategies [9].
4.3 Enhancing Cybersecurity in Smart City Environments
AI and ML play a significant role in enhancing cybersecurity in smart city environments. ML algorithms can analyze network traffic, detect anomalies, and identify potential cyber threats [48]. These technologies enable early detection and mitigation of cyberattacks, protecting critical infrastructure and sensitive data in smart city systems [48]. AI-powered systems can also adaptively learn and evolve to counter emerging cyber threats, improving the resilience and robustness of cybersecurity defenses [49].
5 Smart Governance and Citizen Services
5.1 AI-powered Public Involvement and Engagement
AI technologies make it easier for citizens to participate and engage by enabling personalized interactions and gathering valuable insights. For instance, AI-powered chatbots and virtual assistants can provide citizens with instant access to information, services, and support [50]. These intelligent systems can handle citizen queries, provide recommendations, and guide users through various government processes. AI algorithms can also analyze citizen sentiment from social media and other sources, providing policymakers with real-time feedback and understanding of public opinion [51]. This enhances citizen-government interaction and promotes inclusive governance.
5.2 Streamlining Government Services through Chatbots and Virtual Assistants
Chatbots and virtual assistants powered by AI offer efficient and streamlined government services. These intelligent systems automate routine processes, such as filling forms, making appointments, and accessing public records, reducing administrative burden [52]. Chatbots can guide citizens through service applications, provide personalized recommendations, and offer 24/7 assistance [52]. By automating these tasks, government agencies can improve service delivery, enhance accessibility, and save time and resources.
5.3 Data-Driven Decision-Making for Urban Policymakers
AI and data analytics allow urban officials to make data-driven decisions. Using extensive data analysis from a variety of sources, including user feedback, IoT devices, and social media, AI algorithms can extract valuable insights and patterns [23]. These insights inform evidence-based policy formulation, urban planning, and service improvements [15]. AI technologies can assist in predicting future scenarios, simulating policy impacts, and optimizing resource allocation for improved governance outcomes [23]. Data-driven decision-making enhances the effectiveness and efficiency of government services, ensuring that policies and initiatives align with citizen needs.
6 AI and ML in Smart Healthcare
6.1 Remote Patient Monitoring and Telemedicine
In order for healthcare practitioners to monitor patients’ health problems remotely and give care at a distance, AI and ML are essential to remote patient monitoring and telemedicine. ML algorithms can analyze patient data collected from wearables, sensors, and electronic health records, detecting anomalies and providing early warning signs for deteriorating health [53]. Telemedicine platforms powered by AI facilitate virtual consultations, remote diagnosis, and personalized treatment recommendations, improving accessibility and convenience for patients [54].
6.2 Using Predictive Analytics to Detect and Respond to Disease Outbreaks
Predictive analytics is used to identify disease outbreaks and take appropriate action. ML models can spot patterns and forecast disease outbreaks by examining a variety of data sources, including social media, electronic health records, and environmental data [55]. AI algorithms can support real-time monitoring of disease spread, identify high-risk areas, and assist in resource allocation and response planning [56]. Early detection and prompt response are crucial for effective disease control and prevention.
6.3 Improving Healthcare Access and Service Delivery in Smart Cities
Healthcare access and service delivery are improved by AI and ML technologies in smart cities. In order to improve clinical decision-making, these technologies can analyse vast amounts of healthcare data, including patient records, medical literature, and therapy recommendations [57]. ML algorithms can assist in diagnosing diseases, predicting treatment outcomes, and recommending personalized treatment plans [58]. AI-powered chatbots and virtual assistants provide timely and accurate information to patients, reducing wait times and improving healthcare access [59]. Additionally, AI and ML can increase operational efficiency in healthcare institutions by streamlining operations and allocating resources more efficiently [60].
7 Ethical and Privacy Considerations
7.1 Ensuring Ethical and Responsible AI and ML Use in Smart Cities
To prevent possible harm and advance social well-being, smart cities must employ AI and ML technologies responsibly and ethically. To regulate the use of AI systems, policies and frameworks that prioritise openness, responsibility, and justice should be created [61]. Design and implementation procedures should incorporate ethical issues, ensuring that AI systems align with societal values and respect human rights [62]. Responsible use of AI also requires ongoing monitoring, auditing, and evaluation of AI algorithms and systems to address potential biases and unintended consequences [61].
7.2 Safeguarding Citizen Privacy and Data Protection
As smart cities rely on extensive data collection and analysis, safeguarding citizen privacy and data protection becomes paramount. To specify how data is gathered, rules for data governance must be developed that are visible and clear, stored, accessed, and shared [63]. To safeguard personal information, strong data anonymization methods, encryption, and secure data storage should be used [64]. Citizens’ consent and control over their data should be respected, and mechanisms for data access, correction, and deletion should be provided [63].
7.3 Mitigating Biases and Promoting Fairness in AI Algorithms
AI algorithms have the potential to perpetuate biases and discrimination if not carefully designed and monitored. Bias mitigation techniques, such as varied and unbiased training data, algorithmic auditing, and continuous evaluation, should be employed to minimize biases in AI algorithms [65]. Fairness metrics and guidelines can help in promoting fairness and equal treatment across different demographic groups [66]. Ensuring transparency in AI decision-making processes can enhance accountability and enable individuals to challenge and understand algorithmic outcomes [62].
8 Challenges, Opportunities, and the Role of AI and ML in Smart City Development
8.1 Challenges and Future Directions
AI and ML in smart cities face several technical issues that need to be resolved. These challenges contain the lack of standardized data formats, interoperability issues among different systems, and the need for robust and scalable algorithms [67]. Further research is required to enhance the accuracy and reliability of AI models, improve the interpretability of ML algorithms, and handle dynamic and complex urban data [68]. Additionally, the deployment of AI and ML systems in resource-constrained environments and the integration of edge computing and technical obstacles must be removed while using cloud technology [69]. The execution of AI and ML in smart cities requires careful policy and regulatory considerations. Privacy, security, and ethical concerns must be addressed to protect citizen rights and ensure responsible use of these technologies [70]. Governments need to establish comprehensive frameworks and rules to control the gathering, using, and sharing of data, and to mitigate potential biases and discriminatory practices [71]. Policymakers working together, technology developers, and citizens are essential to establish trust, transparency, and accountability in the deployment of AI and ML in smart cities. The future of AI and ML in smart cities holds tremendous potential. Integrating AI with Internet of Things (IoT) devices to enable real-time data processing and edge decision-making is one of the emerging trends [69]. Federated learning approaches, which allow multiple entities to collaboratively train ML models without sharing sensitive data, hold promise for privacy-preserving AI in smart cities [72]. Continued advancements in AI algorithms, such as deep learning and reinforcement learning, will enhance the capabilities of smart city systems. Furthermore, the application of AI and ML in sustainability, resilience, and social equity domains of smart cities will contribute to more inclusive and environmentally-friendly urban environments.
The research highlights the significant role of AI and ML in smart city development across various domains. The findings emphasize how these technologies can drive transformative solutions, enhance urban environments, and enhance inhabitants’ quality of life. One key area where AI and ML can make a substantial impact is data analytics and selecting the best alternative. By examining the massive volumes of information gathered from sensors and devices, these technologies can extract valuable insights and patterns, leading to optimized resource allocation, improved services, and enhanced urban planning. This capability has the potential to revolutionize how cities operate and rely on current facts to make wise judgements. Predictive analytics and optimization are another crucial aspect where AI and ML can drive smart city development. By examining both past and present data, ML algorithms can forecast inclinations and configurations, enabling cities to proactively address challenges. This capability can be applied to various areas such as traffic management, energy consumption, and waste management, leading to optimized resource allocation and improved operational efficiency. Intelligent transportation systems stand out as a key domain where AI and ML can substantially enhance smart cities. AI algorithms can offer real-time traffic monitoring by using real-time data, congestion prediction, and adaptive traffic signal control. These technologies contribute to improved traffic flow, reduced congestion, and enhanced mobility services. The integration of connected vehicle technologies further enhances traffic management and traveler information. In order to improve public safety and security in smart cities, AI and ML are also essential. Intelligent surveillance systems powered by AI algorithms can analyze video feeds and detect anomalies, improving situational awareness and enabling proactive security measures. Large volumes of data may be processed by ML models to find patterns associated with crime, supporting law enforcement agencies in preventing and responding to incidents effectively. Smart energy management is another domain where AI and ML can drive sustainability in smart cities. ML algorithms can optimize energy consumption and distribution by analyzing usage of energy patterns and incorporating renewable sources of electricity into the grid. These technologies contribute to more efficient resource utilization and reduced environmental impact. The research also highlights the importance of AI and ML in improving urban planning and infrastructure development. By integrating GIS, remote sensing, and data analytics, these technologies enhance decision-making processes and enable evidence-based urban planning. ML models can predict population growth, traffic flows, and energy demands, aiding in infrastructure planning and resource allocation. However, the implementation of AI and ML in smart cities comes with ethical and privacy considerations. Ensuring responsible and ethical use of these technologies is crucial to avoid potential harm and promote societal well-being. Safeguarding citizen privacy and data protection is also essential in the era of extensive data collection. To guarantee equality across all demographic groups, it is vital to address biases and promote fairness in AI systems. While AI and ML offer immense potential for smart city development, several challenges need to be addressed. Technical challenges include standardizing data formats, addressing interoperability issues, and developing robust and scalable algorithms. Policy and regulatory considerations are crucial to establish frameworks that protect citizen rights and ensure responsible use of AI and ML. Building trust, transparency, and accountability in the use of these technologies requires cooperation amongst stakeholders, including decision-makers, technology developers, and people. Looking ahead, emerging trends such as the integration of AI with IoT devices and federated learning approaches hold promise for further advancements in smart city development. Continued advancements in AI algorithms, such as deep learning and reinforcement learning, will expand the capabilities of smart city systems. Additionally, applying AI and ML in sustainability, resilience, and social equity domains will contribute to more inclusive and environmentally-friendly urban environments.
Smart city integration of AI and ML has the ability to revolutionise urban landscapes and raise citizen quality of life. Throughout this discussion, we have highlighted the various ways in which AI and ML can revolutionize smart cities, including optimizing energy distribution, enhancing transportation systems, improving public safety, streamlining governance, and transforming healthcare services. These technologies can enable more efficient, sustainable, and inclusive urban environments. Successful implementation of AI and ML in smart cities requires collaborative efforts among governments, industry stakeholders, researchers, and citizens. Collaborative approaches facilitate knowledge sharing, resource pooling, and coordinated decision-making, leading to more effective and impactful smart city initiatives [12]. By working together, we can leverage the expertise and resources of different stakeholders, overcome technical and policy challenges, and ensure that AI and ML technologies are deployed responsibly and ethically. Looking ahead, the future of AI and ML in shaping urban environments is promising. Emerging trends such as the integration of AI with Internet of Things (IoT) devices, edge computing, and advanced data analytics will further enhance the capabilities of smart cities [73]. AI and ML will continue to drive innovations in areas such as autonomous vehicles, predictive maintenance, citizen engagement, and urban planning, enabling cities to become more resilient, sustainable, and citizen-centric. However, as we embrace the transformative potential of AI and ML in smart cities, addressing issues with privacy, ethics, prejudice, and algorithmic transparency is crucial [74]. Policymakers and stakeholders must prioritize the development of robust regulations, standards, and governance frameworks to safeguard citizen privacy, promoting accountability and ensuring justice in the application of AI and ML technologies [75].
Acknowledgement: I would like to express my sincere gratitude to all the individuals who have contributed to the completion of this research paper. Their unwavering support, valuable insights, and encouragement have been instrumental in making this endeavor a success.
Funding Statement: The authors received no specific funding for this study.
Author Contributions: The authors confirm contribution to the paper as follows: study conception and design: GAGAN DEEP, JYOTI VERMA; data collection: JYOTI VERMA; analysis and interpretation of results: GAGAN DEEP, JYOTI VERMA, GAGAN DEEP; draft manuscript preparation: JYOTI VERMA, GAGAN DEEP. All authors reviewed the results and approved the final version of the manuscript.
Availability of Data and Materials: The data used in this study are available upon request to interested researchers and parties who meet the criteria for access to confidential information. However, some data used in this research cannot be publicly released due to the presence of sensitive or proprietary information, which is subject to legal restrictions and confidentiality agreements.
Conflicts of Interest: The authors declare that they have no conflicts of interest to report regarding the present study.
References
1. T. Yigitcanlar, K. Desouza, L. Butler and F. Roozkhosh, “Contributions and risks of artificial intelligence (AI) in building smarter cities: Insights from a systematic review of the literature,” Energies, vol. 13, no. 6, pp. 1473, 2020. [Google Scholar]
2. X. Li, M. Chen, L. Hao, D. Li and Q. Yang, “Artificial intelligence and machine learning for energy management in smart cities: A comprehensive review,” IEEE Transactions on Industrial Informatics, vol. 16, no. 6, pp. 4287–4302, 2020. [Google Scholar]
3. E. Borgia, L. Bracciale, A. Feliziani, V. Ghini and A. Vesco, “Artificial intelligence and machine learning techniques for sustainable mobility in smart cities,” Sustainability, vol. 12, no. 5, pp. 1803, 2020. [Google Scholar]
4. S. M. Thampi, A. Mekala and P. K. Rajan, “Machine learning techniques for smart cities: A comprehensive review,” IEEE Access, vol. 8, pp. 123478–123495, 2020. [Google Scholar]
5. W. Kong, X. Jiang, J. Chen, L. Yu, Y. Zhang et al., “Urban traffic prediction from spatio-temporal data using deep meta learning,” IEEE Transactions on Intelligent Transportation Systems, vol. 19, no. 11, pp. 3667–3677, 2018. [Google Scholar]
6. M. Silva, J. J. P. C. Rodrigues and S. Nepal, “Intelligent Chatbots in smart cities: Architecture, challenges, and open research issues,” IEEE Communications Magazine, vol. 59, no. 1, pp. 148–154, 2021. [Google Scholar]
7. D. Arribas-Bel, J. E. Patino and E. Tranos, “AI and machine learning in cities: Opportunities and challenges for addressing urban challenges,” Applied Geography, vol. 120, pp. 102222, 2020. [Google Scholar]
8. Y. Chen, Z. Lin, X. Zhao, J. Wang, H. Lu et al., “Towards real-time anomaly detection for streaming videos in smart cities,” Future Generation Computer Systems, vol. 92, pp. 82–94, 2019. [Google Scholar]
9. G. O. Mohler, M. B. Short, P. J. Brantingham, F. P. Schoenberg and G. E. Tita, “Self-exciting point process modeling of crime,” Journal of the American Statistical Association, vol. 106, no. 493, pp. 100–108, 2011. [Google Scholar]
10. A. Oliveira, D. Morais and J. J. P. C. Rodrigues, “A comprehensive survey on AI techniques for disaster management in smart cities,” Sustainable Cities and Society, vol. 65, pp. 102619, 2021. [Google Scholar]
11. R. Giffinger, C. Fertner, H. Kramar, R. Kalasek, N. Pichler-Milanović et al., Smart Cities: Ranking of European Medium-Sized Cities. Vienna UT: Centre of Regional Science, 2007. [Google Scholar]
12. A. Caragliu, C. Del Bo and P. Nijkamp, “Smart cities in Europe,” Journal of Urban Technology, vol. 18, no. 2, pp. 65–82, 2011. [Google Scholar]
13. T. Nam and T. A. Pardo, “Conceptualizing Smart city with dimensions of technology, people, and institutions,” in Proc. of the 12th Annual Int. Digital Government Research Conf.: Digital Government Innovation in Challenging Times, New York, USA, pp. 282–291, 2011. [Google Scholar]
14. R. G. Hollands, “Will the real smart city please stand up?” City, vol. 12, no. 3, pp. 303–320, 2008. [Google Scholar]
15. L. Anthopoulos and P. Fitsilis, “Understanding the smart city domain: A literature review,” in 12th Int. Conf. on Intelligent Environments (IE), Spain, pp. 244–249, 2016. [Google Scholar]
16. N. Komninos, “Intelligent cities: Towards interactive and global innovation environments,” International Journal of Innovation and Regional Development, vol. 1, no. 4, pp. 337–355, 2008. [Google Scholar]
17. A. Zanella, N. Bui, A. Castellani, L. Vangelista and M. Zorzi, “Internet of Things for smart cities,” IEEE Internet of Things Journal, vol. 1, no. 1, pp. 22–32, 2014. [Google Scholar]
18. M. Batty, K. W. Axhausen, F. Giannotti, A. Pozdnoukhov, A. Bazzani et al., “Smart cities of the future,” European Physical Journal Special Topics, vol. 214, no. 1, pp. 481–518, 2012. [Google Scholar]
19. M. M. Rathore, A. Paul, W. H. Hong, H. Seo, I. Awan et al., “Exploiting IoT and big data analytics: Defining Smart Digital City using real-time urban data,” Sustainable Cities and Society, vol. 40, pp. 600–610, 2018. [Google Scholar]
20. H. Jin, Y. Wen, C. Lin and Q. Yang, “Cloud-based wireless sensor networks for smart city,” IEEE Wireless Communications, vol. 21, no. 6, pp. 128–135, 2014. [Google Scholar]
21. A. Botta, W. de Donato, V. Persico and A. Pescapé, “Integration of cloud computing and internet of things: A survey,” Future Generation Computer Systems, vol. 56, no. 7, pp. 684–700, 2016. [Google Scholar]
22. Z. Ullah, F. Al-Turjman, L. Mostarda and R. Gagliardi, “Applications of artificial intelligence and machine learning in smart cities,” Computer Communications, vol. 154, no. 2, pp. 313–323, 2020. [Google Scholar]
23. S. E. Bibri and J. Krogstie, “Smart sustainable cities of the future: An extensive interdisciplinary literature review,” Sustainable Cities and Society, vol. 31, no. 25, pp. 183–212, 2017. [Google Scholar]
24. Q. Shi, A. V. Vasilakos and W. Zhao, “Transportation cyber-physical systems: Challenges and opportunities,” IEEE Transactions on Intelligent Transportation Systems, vol. 20, no. 11, pp. 4157–4164, 2019. [Google Scholar]
25. M. Chen, S. Mao and Y. Liu, “Big data: A survey,” Mobile Networks and Applications, vol. 19, no. 2, pp. 171–209, 2014. [Google Scholar]
26. G. Sagl, B. Resch, B. Hawelka and E. Beinat, “From Social sensor data to collective human behaviour patterns: Analysing and visualising spatio-temporal dynamics in urban environments,” Journal of Location Based Services, vol. 6, no. 2, pp. 71–88, 2012. [Google Scholar]
27. S. Bawa and M. Sharma, “Smart cities: Concepts, technologies, and applications,” in 2016 Int. Conf. on Electrical, Electronics, and Optimization Techniques (ICEEOT), Chennai, India, pp. 550–554, 2016. [Google Scholar]
28. N. Komninos, M. Pallot and H. Schaffers, “Special issue on smart cities and the future internet in Europe,” Journal of the Knowledge Economy, vol. 5, no. 1, pp. 1–4, 2014. [Google Scholar]
29. S. Albawi, A. V. P. Barreto and C. N. Silva, “Machine learning techniques for intelligent traffic management systems in smart cities: A survey,” IEEE Access, vol. 8, pp. 42911–42928, 2020. [Google Scholar]
30. C. Feng, J. Wu, J. Qi, Y. Zhang and J. Yang, “Remote sensing and urban systems: An editorial,” Remote Sensing, vol. 10, no. 5, pp. 668, 2018. [Google Scholar]
31. A. Hosseini, A. Ghaffari and A. Sengur, “Intelligent video surveillance systems: A comprehensive survey,” IEEE Access, vol. 8, pp. 117727–117760, 2020. [Google Scholar]
32. C. L. T. Borges, P. Correia, T. Sousa, Z. Vale and H. Morais, “A review on the integration of distributed energy resources and power systems operation,” Renewable and Sustainable Energy Reviews, vol. 75, pp. 1221–1233, 2017. [Google Scholar]
33. P. Siano, “Demand response and smart grids—A survey,” Renewable and Sustainable Energy Reviews, vol. 30, no. 3, pp. 461–478, 2014. [Google Scholar]
34. D. Yang, Y. Zhang, M. Guo, C. Liu and C. Chen, “A review of intelligent transportation systems with a case study on traffic signal control,” IEEE Access, vol. 4, pp. 4736–4748, 2016. [Google Scholar]
35. E. Safoury and M. Eleiwa, “Smart city applications in transportation systems: State-of-the-art and future directions,” Journal of Sensor and Actuator Networks, vol. 7, no. 4, pp. 55, 2018. [Google Scholar]
36. Y. Feng, Q. Li, Z. Xu, C. Chen and X. Liu, “A survey on urban traffic prediction: From traditional methods to deep learning techniques,” IEEE Access, vol. 7, pp. 101827–101848, 2019. [Google Scholar]
37. H. Wei, W. Ma, Y. Wang, X. Zhang and H. Wang, “Adaptive traffic signal control: Deep reinforcement learning algorithm with experience replay and target network,” Transportation Research Part C: Emerging Technologies, vol. 79, pp. 298–315, 2017. [Google Scholar]
38. Z. Qiu, X. Hu, H. Xu and H. Huang, “Optimal operation of microgrids considering customer satisfaction and economic benefits,” IEEE Transactions on Smart Grid, vol. 9, no. 5, pp. 4600–4612, 2018. [Google Scholar]
39. A. Elbery, X. Wang, A. W. Sadek and B. Abdulhai, “A survey of connected vehicles: Data-driven traffic management and control,” IEEE Transactions on Intelligent Transportation Systems, vol. 22, no. 6, pp. 3604–3622, 2021. [Google Scholar]
40. V. C. Gungor, D. Sahin, T. Kocak, S. Ergut, C. Buccella et al., “Smart grid technologies: Communication technologies and standards,” IEEE Transactions on Industrial Informatics, vol. 7, no. 4, pp. 529–539, 2011. [Google Scholar]
41. H. Farhangi, “The path of the smart grid,” IEEE Power and Energy Magazine, vol. 8, no. 1, pp. 18–28, 2010. [Google Scholar]
42. A. H. Mohsenian-Rad, V. W. S. Wong, J. Jatskevich, R. Schober and A. Leon-Garcia, “Autonomous demand-side management based on game-theoretic energy consumption scheduling for the future smart grid,” IEEE Transactions on Smart Grid, vol. 1, no. 3, pp. 320–331, 2010. [Google Scholar]
43. N. Zhang, X. Chen, M. Jin and Y. Cheng, “Optimal operation of microgrids considering customer satisfaction and economic benefits,” IEEE Transactions on Smart Grid, vol. 9, no. 5, pp. 4600–4612, 2018. [Google Scholar]
44. X. Li, D. Liu, L. Li and Z. Li, “An intelligent waste bin system based on IoT and cloud computing,” Future Generation Computer Systems, vol. 86, pp. 1301–1308, 2018. [Google Scholar]
45. M. S. Hossain, G. Muhammad and D. I. Kim, “Intelligent waste management system using machine learning for smart city,” IEEE Access, vol. 7, pp. 23596–23608, 2019. [Google Scholar]
46. F. Rasouli, J. M.Gómez, F. P. G. Márquez and R. Casas, “Intelligent waste classification system based on deep learning,” Sensors, vol. 18, no. 10, pp. 3229, 2018. [Google Scholar]
47. E. J. Rykiel Jr, “GIS-based urban planning: Lessons from practice,” Journal of the American Planning Association, vol. 78, no. 2, pp. 147–163, 2012. [Google Scholar]
48. A. Bilal, M. Z. Shafiq, S. A. A. Shah and D. S. Kim, “Cybersecurity in smart cities: Challenges, opportunities, and solutions,” Sustainable Cities and Society, vol. 51, pp. 101744, 2019. [Google Scholar]
49. A. Alrawais, A. Alhothaily, C. Hu and X. Cheng, “Machine learning-based intrusion detection techniques for cloud and edge computing: A review,” Journal of Network and Computer Applications, vol. 110, pp. 1–15, 2018. [Google Scholar]
50. M. Joshi, V. Suthar and P. Patel, “Chatbots for government service delivery: Exploring the effectiveness and user experience,” Government Information Quarterly, vol. 38, no. 3, pp. 101628, 2021. [Google Scholar]
51. O. Choudhury, J. Alvarez-Rosario and F. Choudhury, “Using AI to extract citizen sentiment from social media for public policy decision-making,” Government Information Quarterly, vol. 36, no. 4, pp. 101408, 2019. [Google Scholar]
52. A. Espinosa, D. Benslimane and Q. Z. Sheng, “AI chatbots for government-to-citizen services: Issues and challenges,” IEEE Internet Computing, vol. 22, no. 1, pp. 42–49, 2018. [Google Scholar]
53. X. Liu, L. Faes, A. U. Kale, S. K. Wagner, D. J. Fu et al., “A comparison of deep learning performance against health-care professionals in detecting diseases from medical imaging: A systematic review and meta-analysis,” The Lancet Digital Health, vol. 2, no. 6, pp. e271–e297, 2020. [Google Scholar]
54. S. Garg, R. S. Negi and A. Joshi, “Review on telemedicine using machine learning,” in 2019 IEEE 9th Int. Advance Computing Conf. (IACC), India, pp. 738–743, 2019. [Google Scholar]
55. A. F. Dugas, M. Jalalpour, Y. Gel, S. Levin, F. Torcaso et al., “Influenza forecasting with Google Flu trends,” PLoS One, vol. 8, no. 2, pp. e56176, 2013. [Google Scholar] [PubMed]
56. S. Cho, K. H. Sohn, S. Kim and M. G. Kim, “Prediction models for infectious disease outbreaks using artificial intelligence: A systematic review,” Healthcare Informatics Research, vol. 25, no. 1, pp. 2–10, 2019. [Google Scholar]
57. A. Rajkomar, E. Oren, K. Chen, A. M. Dai, N. Hajaj et al., “Scalable and accurate deep learning with electronic health records,” NPJ Digital Medicine, vol. 2, no. 1, pp. 18, 2019. [Google Scholar]
58. A. Esteva, B. Kuprel, R. A. Novoa, J. Ko, S. M. Swetter et al., “Dermatologist-level classification of skin cancer with deep neural networks,” Nature, vol. 542, no. 7639, pp. 115–118, 2017. [Google Scholar] [PubMed]
59. E. J. Topol, “High-performance medicine: The convergence of human and artificial intelligence,” Nature Medicine, vol. 25, no. 1, pp. 44–56, 2019. [Google Scholar] [PubMed]
60. A. B. Kocaballi, S. Berkovsky, J. C.Quiroz, L. Laranjo, H. LTong et al., “The personalization of artificial intelligence in biomedical sciences: Challenges and opportunities,” Journal of Medical Internet Research, vol. 20, no. 5, pp. e10158, 2018. [Google Scholar]
61. A. Jobin, M. Ienca and E. Vayena, “The ethics of AI in health care: A mapping review,” Artificial Intelligence in Medicine, vol. 101, pp. 101–110, 2019. [Google Scholar]
62. L. Floridi, J. Cowls, M. Beltrametti, R. Chatila, P. Chazerand et al., “AI4People—An ethical framework for a good AI society: Opportunities, risks, principles, and recommendations,” Minds and Machines, vol. 28, no. 4, pp. 689–707, 2018. [Google Scholar] [PubMed]
63. T. Yigitcanlar, M. Kamruzzaman and L. Buys, “Big data in smart cities: A review of literature,” Journal of Urban Technology, vol. 26, no. 4, pp. 53–80, 2019. [Google Scholar]
64. S. Al-Fuqaha, A. Gani, F. Al-Obeidat and Q. Al-Kasasbeh, “Data security model for cloud computing,” Journal of Network and Computer Applications, vol. 51, pp. 111–120, 2015. [Google Scholar]
65. N. Mehrabi, F. Morstatter, N. Saxena, K. Lerman and A. Galstyan, “A survey on bias and fairness in machine learning,” ACM Computing Surveys, vol. 52, no. 6, pp. 1–35, 2019. [Google Scholar]
66. S. Barocas, M. Hardt and A. Narayanan, “Fairness and machine learning,” International Journal of Foundations of Computer Science, vol. 30, no. 1, pp. 3–18, 2019. [Google Scholar]
67. N. Abdullah, N. Dey, M. Alazab and R. M. Parizi, “Challenges and opportunities of machine learning in smart cities: A survey,” IEEE Transactions on Emerging Topics in Computing, vol. 7, no. 1, pp. 22–45, 2018. [Google Scholar]
68. Q. Yuan, Y. L. Tang, Y. Zhuang and Z. Li, “Urban big data analytics: From big data to big knowledge,” IEEE Access, vol. 8, pp. 130716–130736, 2020. [Google Scholar]
69. G. Tavares, J. Gama, B. Damas, S. Rousa and P. Sousa, “A survey on smart cities: From concept to future directions,” IEEE Access, vol. 8, pp. 34978–35004, 2020. [Google Scholar]
70. T. Yigitcanlar, M. Kamruzzaman and L. Shi, “Smart cities: Concepts, technologies, and governance frameworks,” Journal of Urban Technology, vol. 28, no. 1, pp. 1–7, 2021. [Google Scholar]
71. P. Budzianowski, T. Janowski, M. Janssen and T. Yigitcanlar, “The role of policy and governance in smart city development,” Journal of Urban Technology, vol. 26, no. 3, pp. 3–6, 2019. [Google Scholar]
72. Q. Yang, Y. Liu, T. Chen and Y. Tong, “Federated machine learning: Concept and applications,” ACM Transactions on Intelligent Systems and Technology, vol. 13, no. 3, pp. 1–20, 2021. [Google Scholar]
73. H. Gupta, S. Katiyar and M. Muzammil, “Big data analytics for smart cities: Challenges, opportunities, and future prospects,” Sustainable Cities and Society, vol. 60, pp. 102221, 2020. [Google Scholar]
74. R. Kitchin, “Thinking critically about and researching smart cities,” GeoJournal, vol. 82, no. 3, pp. 407–420, 2017. [Google Scholar]
75. V. Dignum, B. Berkers, F. Dignum and B. Konat, “Responsible artificial intelligence: How to develop and use AI in a responsible way,” AI and Society, vol. 35, no. 4, pp. 863–977, 2020. [Google Scholar]
Cite This Article
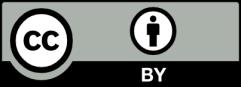