Open Access
ARTICLE
Image Reconstruction Based on Compressed Sensing Measurement Matrix Optimization Method
1 School of Electronics and Information, Yangtze University, Jingzhou, 434023, China
2 College of Engineering and Technology, Yangtze University, Jingzhou, 434020, China
3 School of Computer Science, Yangtze University, Jingzhou, 434023, China
* Corresponding Author: Deshu Lin. Email:
Journal on Internet of Things 2020, 2(1), 47-54. https://doi.org/10.32604/jiot.2020.09117
Received 01 January 2020; Accepted 05 May 2020; Issue published 06 August 2020
Abstract
In this paper, the observation matrix and reconstruction algorithm of compressed sensing sampling theorem are studied. The advantages and disadvantages of greedy reconstruction algorithm are analyzed. The disadvantages of signal sparsely are preset in this algorithm. The sparsely adaptive estimation algorithm is proposed. The compressed sampling matching tracking algorithm supports the set selection and culling atomic standards to improve. The sparse step size adaptive compressed sampling matching tracking algorithm is proposed. The improved algorithm selects the sparsely as the step size to select the support set atom, and the maximum correlation value. Half of the threshold culling algorithm supports the concentration of excess atoms. The experimental results show that the improved algorithm has better power and lower image reconstruction error under the same sparsely criterion, and has higher image reconstruction quality and visual effects.Keywords
Cite This Article
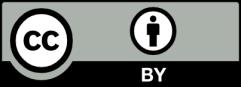