Open Access
ARTICLE
Pancreatic Cancer Data Classification with Quantum Machine Learning
1 Centre for Development of Advanced Computing (C-DAC), Pune, 411008, India
2 Bioinformatics Centre, Savitribai Phule Pune University, Pune, 411007, India
* Corresponding Author: Smita Saxena. Email:
Journal of Quantum Computing 2023, 5, 1-13. https://doi.org/10.32604/jqc.2023.044555
Received 02 August 2023; Accepted 09 October 2023; Issue published 09 November 2023
Abstract
Quantum computing is a promising new approach to tackle the complex real-world computational problems by harnessing the power of quantum mechanics principles. The inherent parallelism and exponential computational power of quantum systems hold the potential to outpace classical counterparts in solving complex optimization problems, which are pervasive in machine learning. Quantum Support Vector Machine (QSVM) is a quantum machine learning algorithm inspired by classical Support Vector Machine (SVM) that exploits quantum parallelism to efficiently classify data points in high-dimensional feature spaces. We provide a comprehensive overview of the underlying principles of QSVM, elucidating how different quantum feature maps and quantum kernels enable the manipulation of quantum states to perform classification tasks. Through a comparative analysis, we reveal the quantum advantage achieved by these algorithms in terms of speedup and solution quality. As a case study, we explored the potential of quantum paradigms in the context of a real-world problem: classifying pancreatic cancer biomarker data. The Support Vector Classifier (SVC) algorithm was employed for the classical approach while the QSVM algorithm was executed on a quantum simulator provided by the Qiskit quantum computing framework. The classical approach as well as the quantum-based techniques reported similar accuracy. This uniformity suggests that these methods effectively captured similar underlying patterns in the dataset. Remarkably, quantum implementations exhibited substantially reduced execution times demonstrating the potential of quantum approaches in enhancing classification efficiency. This affirms the growing significance of quantum computing as a transformative tool for augmenting machine learning paradigms and also underscores the potency of quantum execution for computational acceleration.Keywords
Cite This Article
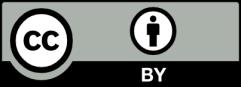