Open Access
ARTICLE
Adaptive Multi-Feature Fusion for Vehicle Micro-Motor Noise Recognition Considering Auditory Perception
1 School of Mechanical Engineering, Southwest Jiaotong University, Chengdu, 610031, China
2 National Laboratory for Rail Transportation, Southwest Jiaotong University, Chengdu, 610031, China
* Corresponding Author: Yudong Wu. Email:
(This article belongs to the Special Issue: Passive and Active Noise Control for Vehicle)
Sound & Vibration 2023, 57, 133-153. https://doi.org/10.32604/sv.2023.044203
Received 24 July 2023; Accepted 12 September 2023; Issue published 21 December 2023
Abstract
The deployment of vehicle micro-motors has witnessed an expansion owing to the progression in electrification and intelligent technologies. However, some micro-motors may exhibit design deficiencies, component wear, assembly errors, and other imperfections that may arise during the design or manufacturing phases. Consequently, these micro-motors might generate anomalous noises during their operation, consequently exerting a substantial adverse influence on the overall comfort of drivers and passengers. Automobile micro-motors exhibit a diverse array of structural variations, consequently leading to the manifestation of a multitude of distinctive auditory irregularities. To address the identification of diverse forms of abnormal noise, this research presents a novel approach rooted in the utilization of vibro-acoustic fusion-convolutional neural network (VAF-CNN). This method entails the deployment of distinct network branches, each serving to capture disparate features from the multi-sensor data, all the while considering the auditory perception traits inherent in the human auditory system. The intermediary layer integrates the concept of adaptive weighting of multi-sensor features, thus affording a calibration mechanism for the features hailing from multiple sensors, thereby enabling a further refinement of features within the branch network. For optimal model efficacy, a feature fusion mechanism is implemented in the concluding layer. To substantiate the efficacy of the proposed approach, this paper initially employs an augmented data methodology inspired by modified SpecAugment, applied to the dataset of abnormal noise samples, encompassing scenarios both with and without in-vehicle interior noise. This serves to mitigate the issue of limited sample availability. Subsequent comparative evaluations are executed, contrasting the performance of the model founded upon single-sensor data against other feature fusion models reliant on multi-sensor data. The experimental results substantiate that the suggested methodology yields heightened recognition accuracy and greater resilience against interference. Moreover, it holds notable practical significance in the engineering domain, as it furnishes valuable support for the targeted management of noise emanating from vehicle micro-motors.Graphical Abstract
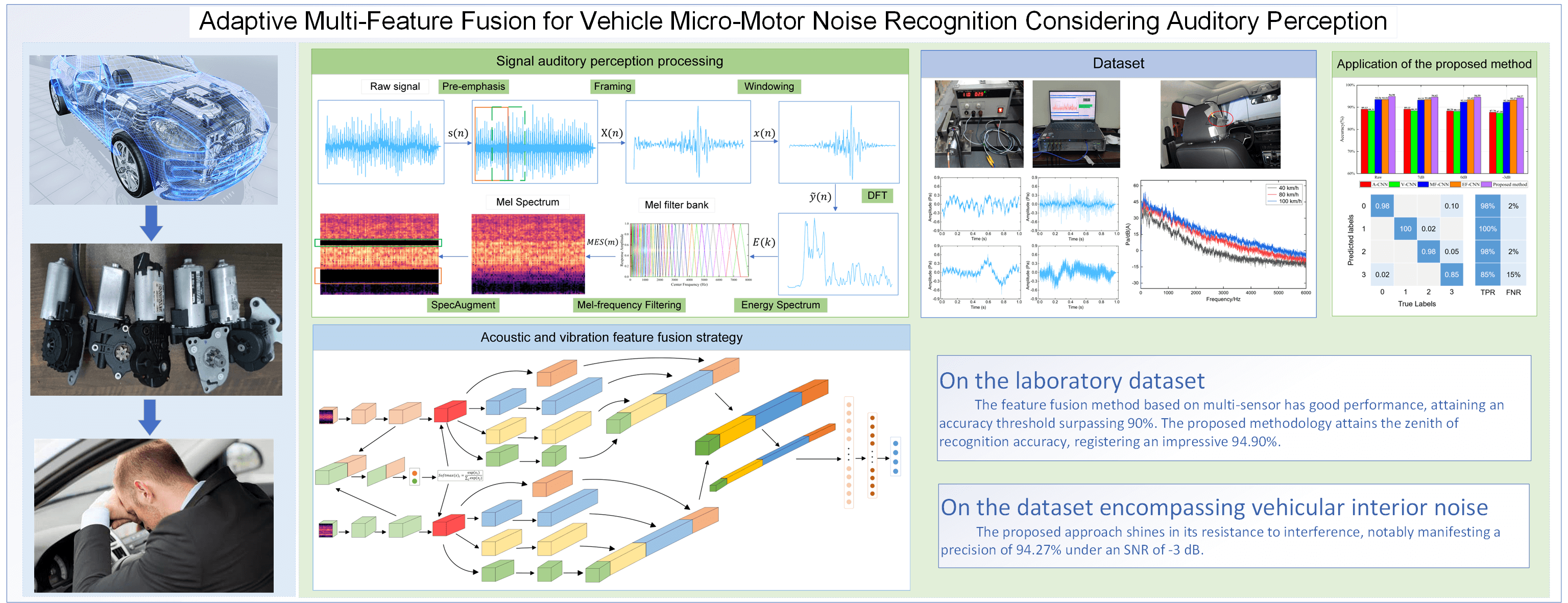
Keywords
Cite This Article
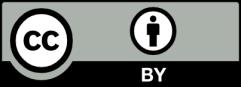