Open Access
ARTICLE
Extracting Sub-Networks from Brain Functional Network Using Graph Regularized Nonnegative Matrix Factorization
1 School of Information Science & Engineering, Changzhou University, Changzhou, 213164, China.
2 School of Architecture Building & Civil engineering, Loughborough University, Loughborough, LE11 3TU, UK.
* Corresponding Authors: Zhuqing Jiao. Email: ; Shuihua Wang. Email:
.
(This article belongs to the Special Issue: Recent Advances on Deep Learning for Medical Signal Analysis (RADLMSA))
Computer Modeling in Engineering & Sciences 2020, 123(2), 845-871. https://doi.org/10.32604/cmes.2020.08999
Received 01 November 2019; Accepted 20 November 2019; Issue published 01 May 2020
Abstract
Currently, functional connectomes constructed from neuroimaging data have emerged as a powerful tool in identifying brain disorders. If one brain disease just manifests as some cognitive dysfunction, it means that the disease may affect some local connectivity in the brain functional network. That is, there are functional abnormalities in the sub-network. Therefore, it is crucial to accurately identify them in pathological diagnosis. To solve these problems, we proposed a sub-network extraction method based on graph regularization nonnegative matrix factorization (GNMF). The dynamic functional networks of normal subjects and early mild cognitive impairment (eMCI) subjects were vectorized and the functional connection vectors (FCV) were assembled to aggregation matrices. Then GNMF was applied to factorize the aggregation matrix to get the base matrix, in which the column vectors were restored to a common sub-network and a distinctive sub-network, and visualization and statistical analysis were conducted on the two sub-networks, respectively. Experimental results demonstrated that, compared with other matrix factorization methods, the proposed method can more obviously reflect the similarity between the common subnetwork of eMCI subjects and normal subjects, as well as the difference between the distinctive sub-network of eMCI subjects and normal subjects, Therefore, the high-dimensional features in brain functional networks can be best represented locally in the lowdimensional space, which provides a new idea for studying brain functional connectomes.Keywords
Cite This Article
Citations
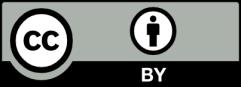