Open Access
ARTICLE
Traffic Flow Statistics Method Based on Deep Learning and Multi-Feature Fusion
College of Mechanical and Electrical Engineering, Qingdao University, Qingdao, 266071, China
* Corresponding Author: Hong Zhao. Email:
(This article belongs to the Special Issue: Modeling and Analysis of Autonomous Intelligence)
Computer Modeling in Engineering & Sciences 2021, 129(2), 465-483. https://doi.org/10.32604/cmes.2021.017276
Received 28 April 2021; Accepted 11 August 2021; Issue published 08 October 2021
Abstract
Traffic flow statistics have become a particularly important part of intelligent transportation. To solve the problems of low real-time robustness and accuracy in traffic flow statistics. In the DeepSort tracking algorithm, the Kalman filter (KF), which is only suitable for linear problems, is replaced by the extended Kalman filter (EKF), which can effectively solve nonlinear problems and integrate the Histogram of Oriented Gradient (HOG) of the target. The multi-target tracking framework was constructed with YOLO V5 target detection algorithm. An efficient and long-running Traffic Flow Statistical framework (TFSF) is established based on the tracking framework. Virtual lines are set up to record the movement direction of vehicles to more accurate and detailed statistics of traffic flow. In order to verify the robustness and accuracy of the traffic flow statistical framework, the traffic flow in different scenes of actual road conditions was collected for verification. The experimental validation shows that the accuracy of the traffic statistics framework reaches more than 93%, and the running speed under the detection data set in this paper is 32.7FPS, which can meet the real-time requirements and has a particular significance for the development of intelligent transportation.Keywords
Cite This Article
Citations
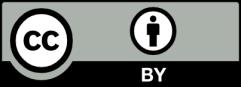