Open Access
REVIEW
A Survey on Machine Learning in COVID-19 Diagnosis
1
School of Educational Science, Nanjing Normal University, Nanjing, 210023, China
2 School of Informatics, University of Leicester, Leicester, LE17RH, UK
#
These authors contributed equally
* Corresponding Author:Zhihai Lu. Email:
Computer Modeling in Engineering & Sciences 2022, 130(1), 23-71. https://doi.org/10.32604/cmes.2021.017679
Received 30 May 2021; Accepted 09 August 2021; Issue published 29 November 2021
Abstract
Since Corona Virus Disease 2019 outbreak, many expert groups worldwide have studied the problem and proposed many diagnostic methods. This paper focuses on the research of Corona Virus Disease 2019 diagnosis. First, the procedure of the diagnosis based on machine learning is introduced in detail, which includes medical data collection, image preprocessing, feature extraction, and image classification. Then, we review seven methods in detail: transfer learning, ensemble learning, unsupervised learning and semi-supervised learning, convolutional neural networks, graph neural networks, explainable deep neural networks, and so on. What’s more, the advantages and limitations of different diagnosis methods are compared. Although the great achievements in medical images classification in recent years, Corona Virus Disease 2019 images classification based on machine learning still encountered many problems. For example, the highly unbalanced dataset, the difficulty of collecting labeled data, and the poor quality of the data. Aiming at these problems, we propose some solutions and provide a comprehensive presentation for future research.Keywords
Cite This Article
Citations
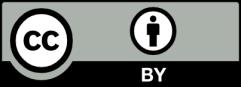