Open Access
ARTICLE
Prediction of Photosynthetic Carbon Assimilation Rate of Individual Rice Leaves under Changes in Light Environment Using BLSTM-Augmented LSTM
1 Graduate School of Bio-Applications and Systems Engineering, Tokyo University of Agriculture and Technology, Koganei City,
184-8588, Japan
2
Institute of Agriculture, Tokyo University of Agriculture and Technology, Fuchu City, 183-0054, Japan
3
Institute of Engineering, Tokyo University of Agriculture and Technology, Koganei City, 184-8588, Japan
* Corresponding Author: Kenichi Tatsumi. Email:
Computer Modeling in Engineering & Sciences 2022, 133(3), 557-577. https://doi.org/10.32604/cmes.2022.020623
Received 03 December 2021; Accepted 22 February 2022; Issue published 03 August 2022
Abstract
A model to predict photosynthetic carbon assimilation rate (A) with high accuracy is important for forecasting crop yield and productivity. Long short-term memory (LSTM), a neural network suitable for time-series data, enables prediction with high accuracy but requires mesophyll variables. In addition, for practical use, it is desirable to have a technique that can predict A from easily available information. In this study, we propose a BLSTMaugmented LSTM (BALSTM) model, which utilizes bi-directional LSTM (BLSTM) to indirectly reproduce the mesophyll variables required for LSTM. The most significant feature of the proposed model is that its hybrid architecture uses only three relatively easy-to-collect external environmental variables—photosynthetic photon flux density (Qin), ambient CO2 concentration (Ca), and temperature (Tair)—to generate mesophyll CO2 concentration (Ci) and stomatal conductance to water vapor (gsw) as intermediate outputs. Then, A is predicted by applying the obtained intermediate outputs to the learning model. Accordingly, in this study, 1) BALSTM (Qin, Ca, Tair) had a significantly higher A prediction accuracy than LSTM (Qin, Ca, Tair) in case of using only Qin, Ca, and Tair; 2) BALSTMCi,gsw, which had Ci and gsw as intermediate products, had the highest A prediction accuracy compared with other candidates; and 3) for samples where LSTM (Qin, Ca, Tair)) had poor prediction accuracy, BALSTMCi,gsw (Qin, Ca, Tair)) clearly improved the results. However, it was found that incorrect predictions may be formed when certain factors are not reflected in the data (e.g., timing, cultivar, and growth stage) or when the training data distribution that accounts for these factors differs from the predicted data distribution. Therefore, a robust model should be constructed in the future to improve the prediction accuracy of A by conducting gasexchange measurements (incl uding a wide range of external environmental values) and by increasing the number of training data samples.Keywords
Cite This Article
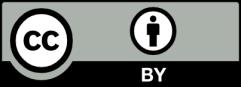