Open Access
ARTICLE
Deep Learning Predicts Stress–Strain Relations of Granular Materials Based on Triaxial Testing Data
1 Zienkiewicz Centre for Computational Engineering, College of Engineering, Swansea University, Swansea, Wales, SA1 8EP, UK
2 College of Shipbuilding Engineering, Harbin Engineering University, Harbin, 150001, China
3 Institute of Applied Mechanics and Biomedical Engineering, Taiyuan University of Technology, Taiyuan, 030024, China
4 Fluid Dynamics and Solid Mechanics Group, Theoretical Division, Los Alamos National Laboratory, Los Alamos, NM 87545, USA
* Corresponding Author: Y. T. Feng. Email:
(This article belongs to the Special Issue: Computational Mechanics of Granular Materials and its Engineering Applications)
Computer Modeling in Engineering & Sciences 2021, 128(1), 129-144. https://doi.org/10.32604/cmes.2021.016172
Received 13 February 2021; Accepted 08 April 2021; Issue published 28 June 2021
Abstract
This study presents an AI-based constitutive modelling framework wherein the prediction model directly learns from triaxial testing data by combining discrete element modelling (DEM) and deep learning. A constitutive learning strategy is proposed based on the generally accepted frame-indifference assumption in constructing material constitutive models. The low-dimensional principal stress-strain sequence pairs, measured from discrete element modelling of triaxial testing, are used to train recurrent neural networks, and then the predicted principal stress sequence is augmented to other high-dimensional or general stress tensor via coordinate transformation. Through detailed hyperparameter investigations, it is found that long short-term memory (LSTM) and gated recurrent unit (GRU) networks have similar prediction performance in constitutive modelling problems, and both satisfactorily predict the stress responses of granular materials subjected to a given unseen strain path. Furthermore, the unique merits and ongoing challenges of data-driven constitutive models for granular materials are discussed.Keywords
Cite This Article
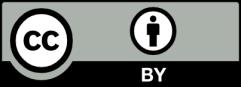