Open Access
ARTICLE
An Efficient Differential Evolution for Truss Sizing Optimization Using AdaBoost Classifier
Hanoi University of Civil Engineering, Hanoi, 100000, Vietnam
* Corresponding Author: Tran-Hieu Nguyen. Email:
(This article belongs to the Special Issue: New Trends in Structural Optimization)
Computer Modeling in Engineering & Sciences 2023, 134(1), 429-458. https://doi.org/10.32604/cmes.2022.020819
Received 14 December 2021; Accepted 25 February 2022; Issue published 24 August 2022
Abstract
Design constraints verification is the most computationally expensive task in evolutionary structural optimization due to a large number of structural analyses that must be conducted. Building a surrogate model to approximate the behavior of structures instead of the exact structural analyses is a possible solution to tackle this problem. However, most existing surrogate models have been designed based on regression techniques. This paper proposes a novel method, called CaDE, which adopts a machine learning classification technique for enhancing the performance of the Differential Evolution (DE) optimization. The proposed method is separated into two stages. During the first optimization stage, the original DE is implemented as usual, but all individuals produced in this phase are stored as inputs of the training data. Based on design constraints verification, these individuals are labeled as “safe” or “unsafe” and their labels are saved as outputs of the training data. When collecting enough data, an AdaBoost model is trained to evaluate the safety state of structures. This model is then used in the second stage to preliminarily assess new individuals, and unpromising ones are rejected without checking design constraints. This method reduces unnecessary structural analyses, thereby shortens the optimization process. Five benchmark truss sizing optimization problems are solved using the proposed method to demonstrate its effectiveness. The obtained results show that the CaDE finds good optimal designs with less structural analyses in comparison with the original DE and four other DE variants. The reduction rate of five examples ranges from 18 to over 50%. Moreover, the proposed method is applied to a real-size transmission tower design problem to exhibit its applicability in practice.Keywords
Cite This Article
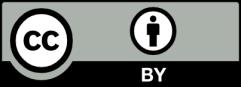