Open Access
ARTICLE
Qualia Role-Based Quantity Relation Extraction for Solving Algebra Story Problems
Faculty of Artificial Intelligence in Education, Central China Normal University, Wuhan, 430079, China
* Corresponding Author: Ting Zhang. Email:
(This article belongs to the Special Issue: Humanized Computing and Reasoning in Teaching and Learning)
Computer Modeling in Engineering & Sciences 2023, 136(1), 403-419. https://doi.org/10.32604/cmes.2023.023242
Received 15 April 2022; Accepted 05 September 2022; Issue published 05 January 2023
Abstract
A qualia role-based entity-dependency graph (EDG) is proposed to represent and extract quantity relations for solving algebra story problems stated in Chinese. Traditional neural solvers use end-to-end models to translate problem texts into math expressions, which lack quantity relation acquisition in sophisticated scenarios. To address the problem, the proposed method leverages EDG to represent quantity relations hidden in qualia roles of math objects. Algorithms were designed for EDG generation and quantity relation extraction for solving algebra story problems. Experimental result shows that the proposed method achieved an average accuracy of 82.2% on quantity relation extraction compared to 74.5% of baseline method. Another prompt learning result shows a 5% increase obtained in problem solving by injecting the extracted quantity relations into the baseline neural solvers.Keywords
Cite This Article
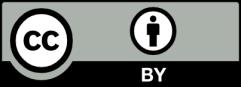