Open Access
ARTICLE
Residential Energy Consumption Forecasting Based on Federated Reinforcement Learning with Data Privacy Protection
1 School of Electronic and Information Engineering, Suzhou University of Science and Technology, Suzhou, 215009, China
2 Jiangsu Province Key Laboratory of Intelligent Building Energy Efficiency, Suzhou University of Science and Technology, Suzhou, 215009, China
3 Chongqing Industrial Big Data Innovation Center Co., Ltd., Chongqing, 400707, China
* Corresponding Authors: You Lu. Email: ; Linqian Cui. Email:
# Both are the first authors due to their equal contributions
(This article belongs to the Special Issue: Advanced Intelligent Decision and Intelligent Control with Applications in Smart City)
Computer Modeling in Engineering & Sciences 2023, 137(1), 717-732. https://doi.org/10.32604/cmes.2023.027032
Received 10 October 2022; Accepted 29 December 2022; Issue published 23 April 2023
Abstract
Most studies have conducted experiments on predicting energy consumption by integrating data for model training. However, the process of centralizing data can cause problems of data leakage. Meanwhile, many laws and regulations on data security and privacy have been enacted, making it difficult to centralize data, which can lead to a data silo problem. Thus, to train the model while maintaining user privacy, we adopt a federated learning framework. However, in all classical federated learning frameworks secure aggregation, the Federated Averaging (FedAvg) method is used to directly weight the model parameters on average, which may have an adverse effect on te model. Therefore, we propose the Federated Reinforcement Learning (FedRL) model, which consists of multiple users collaboratively training the model. Each household trains a local model on local data. These local data never leave the local area, and only the encrypted parameters are uploaded to the central server to participate in the secure aggregation of the global model. We improve FedAvg by incorporating a Q-learning algorithm to assign weights to each locally uploaded local model. And the model has improved predictive performance. We validate the performance of the FedRL model by testing it on a real-world dataset and compare the experimental results with other models. The performance of our proposed method in most of the evaluation metrics is improved compared to both the centralized and distributed models.Keywords
Cite This Article
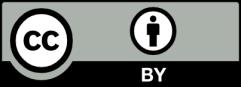