Open Access
ARTICLE
Physics-Informed AI Surrogates for Day-Ahead Wind Power Probabilistic Forecasting with Incomplete Data for Smart Grid in Smart Cities
1 School of Reliability and Systems Engineering, Beihang University, Beijing, China
2 Institute of Reliability Engineering, Beihang University, Beijing, China
* Corresponding Author: Qiang Feng. Email:
(This article belongs to the Special Issue: AI and Machine Learning Modeling in Civil and Building Engineering)
Computer Modeling in Engineering & Sciences 2023, 137(1), 527-554. https://doi.org/10.32604/cmes.2023.027124
Received 14 October 2022; Accepted 05 January 2023; Issue published 23 April 2023
Abstract
Due to the high inherent uncertainty of renewable energy, probabilistic day-ahead wind power forecasting is crucial for modeling and controlling the uncertainty of renewable energy smart grids in smart cities. However, the accuracy and reliability of high-resolution day-ahead wind power forecasting are constrained by unreliable local weather prediction and incomplete power generation data. This article proposes a physics-informed artificial intelligence (AI) surrogates method to augment the incomplete dataset and quantify its uncertainty to improve wind power forecasting performance. The incomplete dataset, built with numerical weather prediction data, historical wind power generation, and weather factors data, is augmented based on generative adversarial networks. After augmentation, the enriched data is then fed into a multiple AI surrogates model constructed by two extreme learning machine networks to train the forecasting model for wind power. Therefore, the forecasting models’ accuracy and generalization ability are improved by mining the implicit physics information from the incomplete dataset. An incomplete dataset gathered from a wind farm in North China, containing only 15 days of weather and wind power generation data with missing points caused by occasional shutdowns, is utilized to verify the proposed method’s performance. Compared with other probabilistic forecasting methods, the proposed method shows better accuracy and probabilistic performance on the same incomplete dataset, which highlights its potential for more flexible and sensitive maintenance of smart grids in smart cities.Graphic Abstract
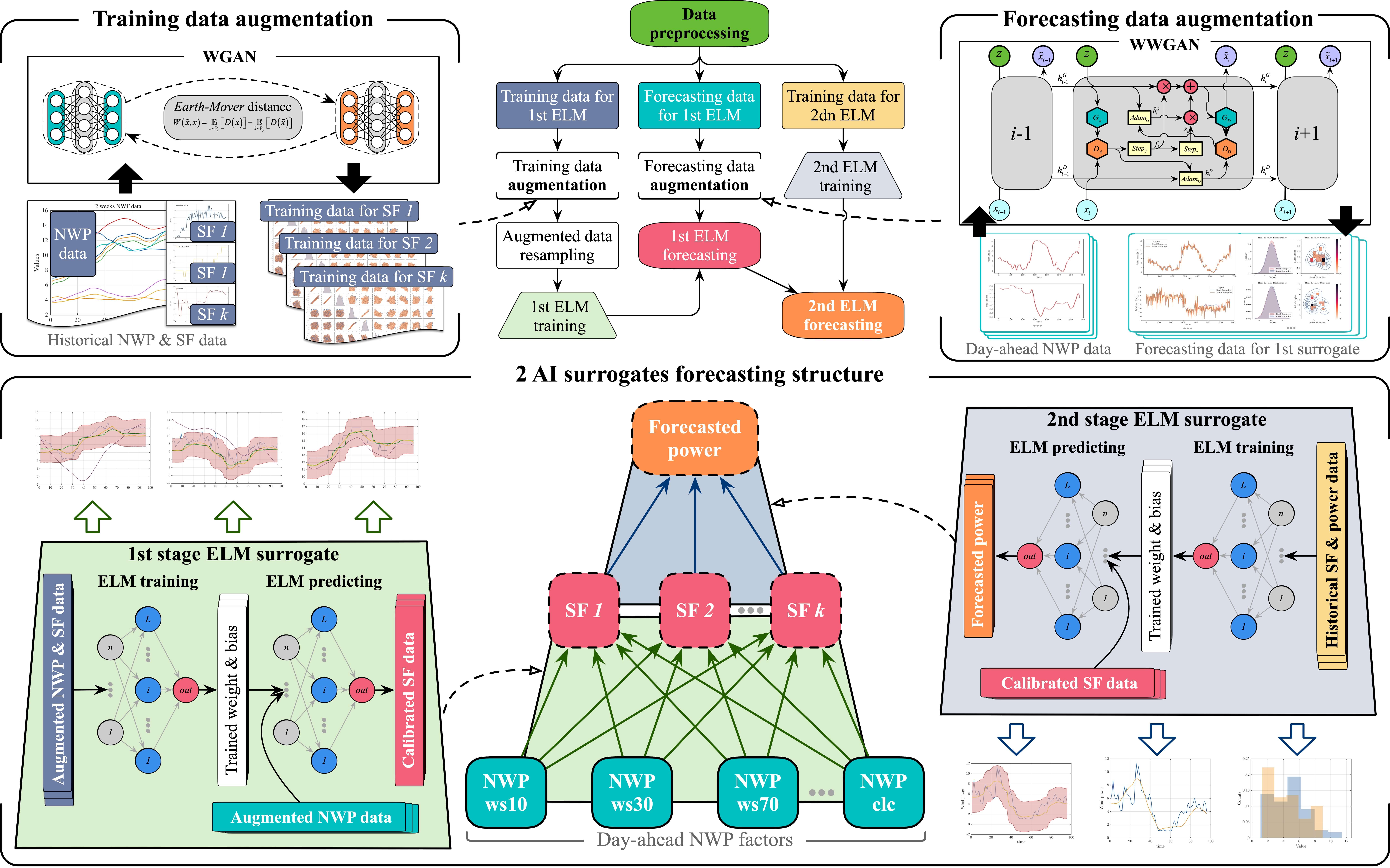
Keywords
Cite This Article
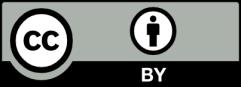