Open Access
ARTICLE
An Improved Lung Cancer Segmentation Based on Nature-Inspired Optimization Approaches
1 Chitkara University Institute of Engineering and Technology, Chitkara University, Rajpura, Punjab, 140401, India
2 Higher Polytechnic School, Universidad Europea del Atlántico, C/Isabel Torres 21, Santander, 39011, Spain
3 Department of Engineering, Universidad Internacional Iberoamericana, Arecibo, PR, 00613, USA
4 Uttaranchal Institute of Technology, Uttaranchal University, Dehradun, Uttarakhand, 248007, India
5 Computer Science Department, King Saud University, Riyadh, Saudi Arabia
6 Faculty of Medicine, Jordan University of Science and Technology, Irbid, Jordan
* Corresponding Authors: Surya Narayan Panda. Email: ; Ishu Sharma. Email:
Computer Modeling in Engineering & Sciences 2024, 138(2), 1051-1075. https://doi.org/10.32604/cmes.2023.030712
Received 19 April 2023; Accepted 06 June 2023; Issue published 17 November 2023
Abstract
The distinction and precise identification of tumor nodules are crucial for timely lung cancer diagnosis and planning intervention. This research work addresses the major issues pertaining to the field of medical image processing while focusing on lung cancer Computed Tomography (CT) images. In this context, the paper proposes an improved lung cancer segmentation technique based on the strengths of nature-inspired approaches. The better resolution of CT is exploited to distinguish healthy subjects from those who have lung cancer. In this process, the visual challenges of the K-means are addressed with the integration of four nature-inspired swarm intelligent techniques. The techniques experimented in this paper are K-means with Artificial Bee Colony (ABC), K-means with Cuckoo Search Algorithm (CSA), K-means with Particle Swarm Optimization (PSO), and K-means with Firefly Algorithm (FFA). The testing and evaluation are performed on Early Lung Cancer Action Program (ELCAP) database. The simulation analysis is performed using lung cancer images set against metrics: precision, sensitivity, specificity, f-measure, accuracy, Matthews Correlation Coefficient (MCC), Jaccard, and Dice. The detailed evaluation shows that the K-means with Cuckoo Search Algorithm (CSA) significantly improved the quality of lung cancer segmentation in comparison to the other optimization approaches utilized for lung cancer images. The results exhibit that the proposed approach (K-means with CSA) achieves precision, sensitivity, and F-measure of 0.942, 0.964, and 0.953, respectively, and an average accuracy of 93%. The experimental results prove that K-means with ABC, K-means with PSO, K-means with FFA, and K-means with CSA have achieved an improvement of 10.8%, 13.38%, 13.93%, and 15.7%, respectively, for accuracy measure in comparison to K-means segmentation for lung cancer images. Further, it is highlighted that the proposed K-means with CSA have achieved a significant improvement in accuracy, hence can be utilized by researchers for improved segmentation processes of medical image datasets for identifying the targeted region of interest.Keywords
Cite This Article
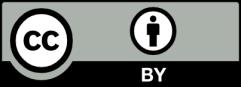