Open Access
ARTICLE
Sparse Adversarial Learning for FDIA Attack Sample Generation in Distributed Smart Grids
1 College of Computer Science and Technology, Shanghai University of Electric Power, Shanghai, 201306, China
2 College of Electrical Engineering, Shanghai University of Electric Power, Shanghai, 201306, China
* Corresponding Author: Fengyong Li. Email:
(This article belongs to the Special Issue: Machine Learning Empowered Distributed Computing: Advance in Architecture, Theory and Practice)
Computer Modeling in Engineering & Sciences 2024, 139(2), 2095-2115. https://doi.org/10.32604/cmes.2023.044431
Received 30 July 2023; Accepted 10 November 2023; Issue published 29 January 2024
Abstract
False data injection attack (FDIA) is an attack that affects the stability of grid cyber-physical system (GCPS) by evading the detecting mechanism of bad data. Existing FDIA detection methods usually employ complex neural network models to detect FDIA attacks. However, they overlook the fact that FDIA attack samples at public-private network edges are extremely sparse, making it difficult for neural network models to obtain sufficient samples to construct a robust detection model. To address this problem, this paper designs an efficient sample generative adversarial model of FDIA attack in public-private network edge, which can effectively bypass the detection model to threaten the power grid system. A generative adversarial network (GAN) framework is first constructed by combining residual networks (ResNet) with fully connected networks (FCN). Then, a sparse adversarial learning model is built by integrating the time-aligned data and normal data, which is used to learn the distribution characteristics between normal data and attack data through iterative confrontation. Furthermore, we introduce a Gaussian hybrid distribution matrix by aggregating the network structure of attack data characteristics and normal data characteristics, which can connect and calculate FDIA data with normal characteristics. Finally, efficient FDIA attack samples can be sequentially generated through interactive adversarial learning. Extensive simulation experiments are conducted with IEEE 14-bus and IEEE 118-bus system data, and the results demonstrate that the generated attack samples of the proposed model can present superior performance compared to state-of-the-art models in terms of attack strength, robustness, and covert capability.Keywords
Cite This Article
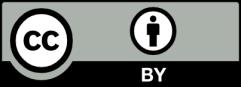