Open Access
ARTICLE
NFHP-RN: A Method of Few-Shot Network Attack Detection Based on the Network Flow Holographic Picture-ResNet
1 School of Cyber Science and Engineering, Sichuan University, Chengdu, 610065, China
2 CyberScience Research Institute, Sichuan University, Chengdu, 610065, China
3 Chengdu Fengwei Technology Co., Ltd., Chengdu, 610041, China
* Corresponding Author: Xingshu Chen. Email:
(This article belongs to the Special Issue: Machine Learning Empowered Distributed Computing: Advance in Architecture, Theory and Practice)
Computer Modeling in Engineering & Sciences 2024, 140(1), 929-955. https://doi.org/10.32604/cmes.2024.048793
Received 18 December 2023; Accepted 22 February 2024; Issue published 16 April 2024
Abstract
Due to the rapid evolution of Advanced Persistent Threats (APTs) attacks, the emergence of new and rare attack samples, and even those never seen before, make it challenging for traditional rule-based detection methods to extract universal rules for effective detection. With the progress in techniques such as transfer learning and meta-learning, few-shot network attack detection has progressed. However, challenges in few-shot network attack detection arise from the inability of time sequence flow features to adapt to the fixed length input requirement of deep learning, difficulties in capturing rich information from original flow in the case of insufficient samples, and the challenge of high-level abstract representation. To address these challenges, a few-shot network attack detection based on NFHP (Network Flow Holographic Picture)-RN (ResNet) is proposed. Specifically, leveraging inherent properties of images such as translation invariance, rotation invariance, scale invariance, and illumination invariance, network attack traffic features and contextual relationships are intuitively represented in NFHP. In addition, an improved RN network model is employed for high-level abstract feature extraction, ensuring that the extracted high-level abstract features maintain the detailed characteristics of the original traffic behavior, regardless of changes in background traffic. Finally, a meta-learning model based on the self-attention mechanism is constructed, achieving the detection of novel APT few-shot network attacks through the empirical generalization of high-level abstract feature representations of known-class network attack behaviors. Experimental results demonstrate that the proposed method can learn high-level abstract features of network attacks across different traffic detail granularities. Compared with state-of-the-art methods, it achieves favorable accuracy, precision, recall, and F1 scores for the identification of unknown-class network attacks through cross-validation on multiple datasets.Keywords
Cite This Article
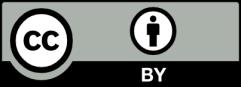