Open Access
REVIEW
Phishing Attacks in Social Engineering: A Review
Department of Computer Science, University of Ghana, Legon Accra, Ghana
* Corresponding Author: Kofi Sarpong Adu-Manu. Email:
Journal of Cyber Security 2022, 4(4), 239-267. https://doi.org/10.32604/jcs.2023.041095
Received 11 April 2023; Accepted 01 June 2023; Issue published 10 August 2023
Abstract
Organisations closed their offices and began working from home online to prevent the spread of the COVID-19 virus. This shift in work culture coincided with increased online use during the same period. As a result, the rate of cybercrime has skyrocketed. This study examines the approaches, techniques, and countermeasures of Social Engineering and phishing in this context. The study discusses recent trends in the existing approaches for identifying phishing assaults. We explore social engineering attacks, categorise them into types, and offer both technical and social solutions for countering phishing attacks which makes this paper different from similar works that mainly focused on the types of attacks. We also show essential human characteristics that make users vulnerable to phishing attacks, their mitigating strategies, challenges, and future directions.Keywords
In the previous two decades, the information technology area has grown considerably, resulting in cutting-edge solutions applicable to different endeavours and industries such as finance, commerce and trading, medical services, energy, and most crucially, education through the use of information systems [1]. Security, data protection, and privacy become increasingly important as organizations grow and expand. Knowing the importance of security concerns, the majority of these organizations focus on and invest in information security tools (software) and equipment (hardware) to secure the organisation’s information and other hardware assets from any kind of threat or danger [2]. Meanwhile, the greatest threat to an organisation’s security is not a computer virus, an unpatched exploitable bug in a critical computer program, or a gravely implemented firewall, but rather the user of that particular information system due to flaws in human behaviour [3,4]. In most organizations, manipulating (controlling) the human agent (that is the personnel) via an information system is more straightforward than influencing the information system (that is the system agent). Organizations frequently overlook the human agent (the user in charge of the information system) who acts as a vital component in the information systems and the potential for a security breach through their activities [4]. As a result, organizations must focus on techniques to secure their information system’s users (the human agent), software, and physical resources (hardware and networks).
The act of social engineering is defined as a threat or attack on an organisation by a human agent (user). Social engineering attacks (or human hacking) can occur in various ways but are most commonly carried out via mobile devices as electronic messages (voice, text, pictures and videos) called phishing [5–7]. The success of social engineering attacks heavily depends on the prospective victim’s willingness to trust the intruder and give the intruder access to crucial information that could be utilized to attack the organisation [8].
Phishing is one of the most common social engineering techniques intruders use to exploit organizations worldwide [9,10]. Applications specifically developed for phishing-based assaults do not exploit system weaknesses or information systems. Such phishing-based programs do not employ regular system features; instead of relying on the user’s inability to distinguish between legal and illegitimate applications within the information system [11]. Because the attack is not focused on the information system but on the user, the solutions must include methods to safeguard the user and the technological system. Organisational regulations that prevent users from performing necessary actions without authorization must be used to limit users.
Researchers have proposed various strategies for identifying phishing attacks in social engineering during the last decade [12,13]. Because phishing attacks are a socio-technical method of social engineering attacks [14], researchers’ suggested preventive strategies to resist phishing attacks are viewed from the perspectives of the user (social interventions) and the computer system (technical intervention). The user interventions provide techniques for the user to shield himself from the invader [15]. In contrast, the system interventions provide ways and constraints to help safeguard the user even more through technical means [15,16]. Recent methods presented by researchers to combat phishing attacks have centred on technology rather than the user (i.e., providing social-oriented solutions). However, most phishing attacks are designed for compromising the user rather than the technological system [12,17,18].
There have been few scholarly reviews on social engineering and phishing [19]. A recent survey was conducted on phishing attack strategies and mitigating techniques where the authors discussed phishing attacks, why intruders use the attack, attack strategies, and countermeasures [19]. A significant limitation of their work was focusing solely on the technical component (device-level) of countermeasures overlooking user-level (directed) attacks which is the primary concern of phishing attacks.
In [13], the authors surveyed online phishing detection methodologies and trends presented automated online phishing detection systems and evaluated their performance in their work. They focused on phishing detection from a technical perspective (device level) without regard to the issues at the user level. In [20], the authors identified and detected phishing activities on the internet using Random Forest (RF) and Artificial Neural Network (ANN) techniques. Their approach recorded 97.4% accuracy in phish detection [20].
A recent study surveyed by [21] reported on phishing website detecting techniques. The authors presented a systematic review of the latest advancements in phishing detection strategies to improve readers’ understanding of those techniques and algorithms. Despite the advances in phishing detection systems, the interventions to identify phishing activities on the Internet are still dependent on the user’s decision to disregard the warnings and notifications. For example, if MacAfee anti-phishing software detects phishing activity on your computer, you can opt to disregard its warnings and proceed. However, if the user is thoroughly trained and educated about the legal repercussions, they will be well informed and make the right decisions.
In this paper, we present social engineering attacks and discuss the mode of attack propagation and life cycle. Out of the types of social engineering attacks, we present an in-depth study on phishing the most common among the social engineering attacks. We classify the attacks into types and discuss the trends and viable countermeasures from two perspectives (the user-level and device-level referred to as socio-technical perspectives). Finally, we discuss phishing activities that took place due to COVID-19.
The rest of the paper is organized as follows. Section 2 discusses the motivation for the paper. Section 3 describes the methodology. The act of human hacking is discussed in Section 4. The section describes many social engineering attacks, best practices for preventing social engineering, and the most recent attack paradigm. Section 5 thoroughly discusses phishing, and Section 6 delves into interventions, focusing on user and system intervention. Section 7 discusses the challenges and future directions. The conclusion of the paper is provided in Section 8.
Falling victim to a phishing attack can result in significant financial loss for an organization or an individual. It may cause crucial organizational information to get into the wrong hands; some intruders even go so far as to seize complete control and refuse the organization’s services (Denial of Service). Intruders only seek pleasure in a few circumstances [14,22].
With the introduction of the Internet and online trading, phishing activities have increased. The COVID-19 pandemic recently resulted in a massive increase in phishing activity, notably since corporate manual tasks were shifted to digital and online platforms. Employees and clients transacted business from various locations through internet platforms, exposing them to multiple threats and attacks. Due to physical movement restrictions, employees had to stay at home, connect over the internet to communicate with others, and undertake all work-related tasks, including meetings. The manual to digital migrations of organisations increased the number of internet users (business and social) and the time users spent on the internet [23].
The significant increase of users online resulted in a surge in cybercrime [24]. Internet users must be educated about cyber security, the most common types of attack propagation, the dangers of the effects if victimised, and their ways of prevention and mitigation [25]. Given the increase in phishing attacks, the frequency with which they occurred, and the changing nature of the attacks during the COVID-19 pandemic, surveying phishing attacks in social engineering is relevant. We are also motivated since the research will provide domain knowledge that will help with information security. The study will go a long way toward providing researchers with a full grasp of phishing, especially with the surge in online users, most of whom are naïve and unaware of the digital world.
It is crucial to explain explicitly that phishing is a strategy of human manipulation rather than a mechanism of machine manipulation. We address the broad context (social engineering) to a more particular dimension in this study (phishing). We examine trends in social engineering, phishing attacks, forms of phishing attack dissemination, examples of attacks and their consequences, and the life cycle of all social engineering attacks.
In this paper, we adopted the Multivocal Literature Review (MLR) as the appropriate literature survey approach to provide state-of-the-art progress in phishing attacks in social engineering. The MLR approach synthesises the publications from a Systematic Literature Review perspective (that is Journals, Published Literature, conferences and workshops) and from a professional perspective (Grey Literature Review). The relevant publications were retrieved based on specific keywords and a combination of Boolean operators such as “AND” and “OR”. These keywords and Boolean operators formed the search strings for our data collection. The researchers gathered data from different sources such as Google Scholar, Web of Science, IEEE Explore, Springer, Elsevier and Scopus. The publication year of these papers spans from 2013 to 2023 (over the past decade). Also, the professional perspective data was collected with the search string on Microsoft Bing and Google search which generated data from well-recognized organisational websites, published books and reports. The researchers intend to draw ideas from diverse areas due to the pervading nature of the issue. For the inclusion criteria, we focused on the following: research that concentrates on social engineering and, or phishing, research on cyber threats and attacks since COVID-19 and research-based empirical analysis. For the exclusion criteria, we focused on repeated and duplicate studies, research papers that were not authored in the English Language and primary research that is not pertinent to the study’s objectives.
4 The Act of Human Hacking (Social Engineering)
This section discusses a broader look at the act of human hacking. Social engineering attacks have become the most common method of committing a crime on the internet. Perpetrators deceive people, obtain access to their information, steal user data, disrupt users’ computer systems and target their information technology infrastructure [1]. Social engineering attacks are sophisticated cyber-security attacks that use innate human nature to penetrate security systems and consequently have some of the most significant success rates [24]. In Fig. 1, social engineering attacks, their purpose, transmission mechanisms, attack life cycle, and mode of attack are illustrated. To succeed, all social engineering attack strategies must go through the same life cycle.
Figure 1: Social engineering goals, processes, modes and lifecycle paradigm
The purpose of the attack is decided by what the attacker desires from the victim or the victim’s organization, and the mode of attack distinguishes social engineering attacks. Some intruding techniques are physical, while others are technical, socio-technical, or sociological. According to the human vulnerability-assessment approach, not all threats and attacks in social engineering affect every user of an information system; humans are often victims of various attack strategies and unique occurrences. This makes detecting and anticipating attacks challenging [11]. The adverse effects of a successful social engineering attack on individuals and organizations are significant [26]. Users may, for example, experience data loss, financial losses, decreased staff morale in the organization, and diminished trust between customers and enterprises [27]. Social engineering attack techniques are classified and labelled based on how the attack is disseminated. Fig. 1 depicts social engineering concerning the many types of social engineering.
According to the Cyber Edge Report 2021, social engineering attacks were the most prevalent since the COVID-19 pandemic. According to them, “the number of organizations hit by at least one effective social engineering attack per year is approximately 79%”. Similarly, a social engineering strategy detected 99% of cyber threats [14]. During the pandemic, phishing attacks were the most prevalent, accounting for around 30% of all cybersecurity attacks, followed by malware attacks before the distributed denial of service attacks [28].
4.1 Types of Social Engineering Attacks
This section explores the seven most common but dangerous social engineering attacks adapted by perpetrators on their victims. These social engineering attacks describe the techniques employed, the tools used, the most common platform used, and some existing tools designed to overcome them.
Phishing is a social engineering attack attempting to scam users of information systems to access personal and cooperate information such as name, address, bank details, and credit card details [29]. It is made of the socio-technical mode of social engineering attacks [14]. Scammers using phishing attacks may embed links to redirect users to suspicious websites that appear legitimate.
These scams create a sense of urgency to manipulate users to act in a manner that challenges good judgment [30]. Helmi et al. defined phishing as a computer security attack. The attacker tends to trick users by using fake websites that are masqueraded to appear and look just like the authentic website—for example, prompting the potential victims to share their data or install it into their computer hidden harmful software [31].
This social engineering attack technique uses tailgating and piggybacking to access restricted areas [32]. The attack is a physical model of social engineering attack [14]. The intruder follows the susceptive victim (an authorised person) into a restricted area in tailgating. It is pretty straightforward as hanging tight for somebody who has approved admittance to enter a place and afterwards acting like somebody who failed to remember an identification or is there for an exact reason, like support [33]. This attack exposes those who have the power to access information systems or restricted areas or grant access to users of those systems by the attacker who may impersonate delivery personnel or others who may require temporal access to the system or premise [30]. For example, an intruder trying to have physical access to an authorised place may ask an authorised person with an RFID to hold the door open to have access because he forgot his RFID Card [32,34].
Pretexting is an attack driven by a fabricated scenario designed by the attacker, attempting to confirm and steal personal or organisational information from the target [30,35]. Before phishing became the most used social engineering technique, pretexting was the pervading [36]. In terms of an advanced intrusion attempt to exploit a weakness of a company or an individual, this method requires the intruder to build a credible story outline that leaves no or little room for questions or doubt by the targeted users. The trick uses fear and urgency while creating a sense of trust in the user while the attacker convinces the victim to confirm or obtain sought information [30].
The pretexting methods require valid information to avoid getting caught [37,38]. This could disadvantage the attacker in getting credible information to convince the susceptive victim. This attack can be presented through a cell phone, electronic mail, or a physical mean [32]. Example “One of the candidates from Shandong province, China, applied for a scholarship. The criminal network got the information about the candidate, and the social engineers contacted the candidate asking him about the scholarship and asking him to pay a remittance, which will be returned with the scholarship amount. The student trusted and transferred the amount, which then is withdrawn by the criminals, thus, making SE attackers successful in their attack” [7]. Another example is the act of reverse social engineering [39].
Baiting is almost the same as a phishing attack, but the difference is that in baiting, the intruder lures a victim through enticement strategies [30,40]. The intruder uses the lure of a promised good result if a user surrenders login credentials to a specific site or performs the intended act designed by the intruder [10,41]. Baiting schemes are not limited only to digital or online systems; they can also be launched successfully through physical media. Baiting was described as a significant controller area network (CANDY) attack that can be established as a trojan house targeted to an information system [30,42]. For example, an email tells users to click on a link to get a gift [32]. The fact that bait is an idea exposed to the user to fall for could be a disadvantage to the attack [43].
This social engineering attack technique is almost the same as baiting and phishing. Still, their uniqueness is that in this type of threat, the intruder presents the attack as a technical support service in exchange for information [30,32]. A common thread is for the attacker to impersonate an information technology representative and offer assistance to a victim who may be experiencing technical challenges. The attacker aims to lunch malware on the user’s system [30]. For example, an attacker pretending to be information IT opts to help a user who needs IT support. By accessing the system the users use to help, the attacker can plant any device or software into the system [30,37].
Shoulder surfing is another social engineering attack technique used widely nowadays [32]. Shoulder surfing can be defined as an act that occurs when attackers try to observe the victim's activities over the victim’s shoulder to get the password, PIN or any other sensitive information [37,44]. This mode of social engineering attack is a physical one. The intruder must be with the victim to launch the attack successfully [14]. Most social engineering attack modes prevent face-to-face meetings with the victim, but in shoulder surfing, the intruder and the victim must be close [39].
This form of social engineering attack primarily works effectively in crowded places. At the same time, the attacker with a targeted victim can sit behind the victim and observe most of their activity even as they enter their PIN in an ATM, enter the password for a system, or even fill out forms that need sensitive information to provide. Since the increase in smart devices with touch screens such as Samsung Galaxy, iPhone and iPad, the risk of shoulder surfing has increased [45]. Most people use these devices to enter their online banking account, business, and personal e-mail while travelling on trains, trams, and buses. Federico, Simone and others claim that the shoulder suffering attack works 97.07% of the time with different touchscreen keyboard devices, with 1.15% of errors [44,46].
Staff impersonation is when an attacker using social engineering calls a user of an information system by phone, manipulates the user into regarding them as an authorised user of the system and asks for a change in authentication detail of the system username or password [32]. The social engineering attack can be executed physically, technically or socially depending on how intruders use it [14]. In some cases, the user in charge asks for the caller’s (who they think is an authorised person) basic information example, their full name and date of birth. The attacker provides a quick response if having the correct data collected about the staff they are impersonating [32]. In another scenario, the intruder can enter the organisation’s premises, presenting themselves as a legitimate employee and asking about a sensitive data storage area such as the server room [46]. Most of the employees in the company will help the intruder think they are new employees who need help knowing the company’s places.
4.2 Recommended Best Practices to Prevent Social Engineering
It is clear that, despite all technology safeguards, there is still a human aspect of vulnerability. The strategies listed below, when correctly implemented, can help to mitigate the social engineering attack techniques depicted in Fig. 2 [14,24,30,47]. Develop a well-thought-out security policy that incorporates both technological and user-level procedures. The information technology support team in preventing and responding to social engineering attacks will aid users by:
• Educating and training users on cyber security qualities and vulnerabilities during and after recruitment.
• Putting in place technical processes to prevent users from performing actions that make them subject to social engineering attacks.
• Employing strong network security, including a blacklist of suspected websites and a whitelist of all domains that users can access.
• Securing the environment and organizational or individual information on physical and virtual locations and multiple levels.
• Conducting regular cyber security audits in the organisation and upgrading software and information. This procedure will close most loopholes intruders may have found in the system.
Figure 2: Recommended best practices to avert social engineering attacks
Phishing is frequently referenced in the media and by organizations such as banks [48] and law enforcement agencies [49]. Phishing attacks have evolved with new methods and media [12]. Phishing is a scalable act of deception in which an attacker disguised as another person attempts to get relevant information from an information system user [49,50]. In their study on the most common social engineering approach used to hack information, the authors discovered that phishing is the most common social engineering attack [51]. All phishing attack strategies are most effective on Android and iOS users [52]. This section provides details on the impact of COVID-19 on phishing activities, trends in phishing attack approach, classification of phishing attack propagation, types of phishing attacks, and phishing and behaviour factors that influence phishing activities.
5.1 Impact of COVID-19 on Phishing
The outbreak of the COVID-19 pandemic has caused a massive increase in online activities, from e-commerce, payment, finance, SaaS, and social media to sending emails [23,24,28]. The growth of the internet caused an increase in cybersecurity attacks and frauds.
Table 1 shows that phishing activity in the fourth quarter of 2020 will quadruple from the fourth quarter of 2019. During this time, the impacts of the COVID-19 pandemic were severe, and all outside activities were curtailed, with some countries experiencing total lockdowns. According to the Anti-Phishing Working Group (APWG), phishing attempts, targeted users, and organizations fluctuate with the seasons. By 2020, over 80% of suspicious websites will have enabled SSL certificates and data encryption [13,53].
5.2 Trends in Phishing Attacks Detection Methodologies
Many works have been done in literature reviews, and software has also been developed in information security concerning phishing attacks (protecting humans against manipulations). Still, not much has been done concerning phishing attacks [54]. It is rather unfortunate that all the software and advice provided by the writers and developers are not effective enough to stop the attacks from happening altogether. They only control and minimise their effects. According to the literature, nine different ways mitigation mechanisms have been developed to combat these phishing attacks. These are Web Content Similarity, Web Structure Similarity, Web Access Log, Domain Blacklist, Domain Blocklist, Domain Whitelist, URL Similarity, Phishtank and Machine Learning. Despite their accuracy, some of these recommended mitigation approaches require complicated calculations and skills, making them difficult for users to use [55].
In work done by [56], the authors argued that the WhatsApp mobile application’s traditional two-factor authentication scheme to authenticate user login is still unsafe. Because the trusted platform module program on the chip, which is a chip on which the user information is stored, can be reprogrammed for malicious activities. Hence, the authors proposed a new user authentication approach that required user name, age, sex, home address, phone number, and email address to be a composite key to identifying a user. Their approach uses the user data, and the user takes the time to input the necessary data during user registration three consecutive times. It will be stored and used to compare the time users take each time they log into the WhatsApp platform. The user is denied access if there is no match with existing records.
The authors proposed authentication scheme is better than the WhatsApp application's current authentication scheme. Still, the system recognises that they fail to make provisions for users if they are not healthy or have the right energy to type at their usual speed. Even though the search was about WhatsApp hacking, the researcher concentrates more on the application itself and not the individuals. In this search, the focus will be on the human aspects that lead to the success of these phishing attacks blended with the system-side interventions [56]. Table 2 presents recent works, their recommended solutions, approaches to combating phishing attacks and their limitations.
5.3 Classification of the Attack Propagation
In this section, the phishing attacks have been classified into propagation mode. The form and phase in which the intruders present the attacks are shown in Table 3.
The medium by which the attack has been propagated defines the name given to the attack. Phishing attack propagation includes; (1) email phishing, (2) vishing, and (3) smishing. A brief description of each type of phishing is provided in the following sections.
Phishing using electronic mail is the medium that comprises the broadest range of attacks among others and is also the first to emerge [31,93]. With this attack technique, specifically crafted emails are distributed to targeted users enticing them to act in a specific way according to the instructions described in the mail, which leads to the disclosure of the user’s data to the attacker. An email phishing attack is straightforward for attackers to propagate because of the ease of sending an email to many users [40,94]. This is different from spear-phishing because email phishing does not target any victim; the email is usually spread over the internet with no direction.
Vision is a phishing attack propagation technique that involves the use of voice. The fact that the use of cell phones to attempt to scam a user is no news. The introduction of VoIP technology brought about an alarming increase in the practice of the attack technique. The technique can spoof a cell phone number in other the call’s IM appears to have come from an authentic source [70]. According to [40], introducing intelligent and automated systems improves the success probability of Vishing attacks by making them difficult to differentiate from an authentic call.
5.4.3 Smishing (SMS/MMS Phishing)
It is the use of short messaging services to implement phishing attacks. Sort and multimedia messaging services are the media responsible for smishing attacks. The SMS method involves sending an SMS pretending to be someone else (such as a bank, school or government agency) containing a vital message [40,70]. The victim of the attack technique is then redirected to a fraudulent website or phone number, which requires the victim of the attack to log in or provide some identifying information. When this occurs, the attackers can use the details gathered. Smishing is not the same as Spear Phishing; Smishing is through a global mobile service, not the internet like spear phishing.
Spear phishing is an attack propagation technique targeted at victims in contrast with the generic mass production of spam mail [95]. More accurately, it is an attack propagation technique against an individual or organisation that utilises specially crafted material to improve the chances that the user who opens the email will be manipulated into doing whatever the sender wants [51,96]. The emails are prepared to have come from a person known to the victim. On the other hand, the attacker’s email content must also relate to something of more importance to the victim so that the email instructions do not look so suspicious to the intended [15,97]. The email may refer to the targeted victim by name and other information that the target has no knowledge an unknown person could have about them. Spear phishing is almost the same as email phishing, but it is more specific considering its tarted victims than email phishing [96].
Whaling is a phishing attack propagation technique whose targeted approach is similar to spear phishing. The highest difference is that a whaling attack is directed at senior-level management in an organisation whose authority provides the attack with the right to gain access to data within their company, not just a user [1,51]. Because this phishing attack is highly targeted, attackers take the best time to prepare and ensure their scams are not easy to detect, making them look like authentic mail to the victims [1]. As in the case of spear phishing, the phisher’s main aim is to manipulate the victim into installing malware on the victim’s device to provide access for the attacker to the targeted information.
This attack propagation technique uses advertisement-hosting services to host adverts that contain malware designed to activate when the victim clicks on the advertisement [70,98]. The main advantage of this phishing attack approach is that advertising is hard to detect and prevent simply because the malware is hosted on an authorised advertisement website [98]. Advertising is challenging to avoid. The advertisement agencies do not require customers (advertisers) to provide accurate details about the advertisement [99]. This approach is different from adware. Adware specifically uses online adverts to distribute malware to victims, and it is less focused and has a wide range of effects on the victims.
A quick response code is a layout matrix containing a black-and-white pixel used to store and communicate compressed information. This two-dimensional QR code is being introduced to replace the previous single-dimensional Barcode because it is easier to read and contains more compressed information [70,100]. A specially designed optical lens is made to scan and read the contents of the QR code then a QR code reader decodes the information contained within the QR code and processes it [100,101]. In this form of attack propagation, the phisher prepares and spreads a QR code around pretending to be an authentic advertisement of a legitimate product. Then the QR code directs the victim of the attack to a malicious website where the phisher has the chance to manipulate the victim and gain access to relevant information [70].
5.5 Phishing and User Behavioural Factors
This section explains the human behavioural factors that expose users to a phishing attack. Intruders using phishing techniques take advantage of the emotional features of humans.
5.5.1 Factors That Make Users Fall Victim to Phishing Attacks
Social engineering using phishing attacks works well by the intruder manipulating the user's emotions [1,102]. Despite the organisations’ and individuals’ efforts to continually update and buy the current security tools and applications, it only takes one naive user to circumvent security controls. They classify these factors into psychological ploys, as presented in Table 4.
5.5.2 User Personality Traits (OCEAN)
The five personality traits model used to classify human behaviour about users being vulnerable to cyber-attacks was discussed [105]. A well-structured personality dimension has five personality traits: openness, conscientiousness, extroversion, agreeableness, and neuroticism (OCEAN) are presented in Table 5 with a detailed explanation of how they relate to using the vulnerability to phishing attacks on the internet.
The interventions for resolving phishing attacks are twofold: user-level interventions (ULIs) and device-level interventions (DLIs). Phishing attacks are best prevented and detected when these two forms of interventions are used simultaneously. The following are the descriptions of both forms of phishing attack interventions.
6.1 User-Level Interventions (ULIs)
Hassandoust conducted a search in 2020 on the “role of contextualisation in user’s vulnerability to phishing attempts”, the intended acts of users against phishing and their actual responses. It was found that users mostly fall victim to phishing attacks because the bait for the attack is in their context and interest [108].
Phishing attacks cannot be adequately prevented and controlled only by Purely Technical Solutions [90]. Defending an information system against phishing attacks is highly difficult because, primarily, attacks are social engineering attacks meant for hacking the user, not the machine [46].
Regular training of the organisation’s employees allows some organisations to limit or sometimes prevent and identify attempts with social engineering technologies [46]. Because the difficulty training users on phishing techniques tends to be much easier than educating them about the organisation’s firewall systems, users in the organisation will no longer be as vulnerable as they were to the threats of the security chain [73,109]. It will be a pervasively lousy habit in an organisation to organise a technical training workshop without security aspects being learnt [106]. Research has shown that despite all the advice and caution, the organisation emphasises the importance of everyday security awareness only after security is breached or when colossal money is lost [67]. All security training organised by the organisation must reinforce both the social engineering attacks and the antisocial engineering attack defences for users to get used to the awareness of confidentiality and increase their sense of responsibility regarding information security [104]. Employees should understand that any simple social engineering attack intrusion with their fault may lead to losses, the organisation’s information landing on the wrong hand, or even the loss of profits [46].
An organisation could possess all advanced anti-virus software, firewall and intrusion detection systems appropriately installed and still be unable to avoid hacking from social engineering attacks [3,110]. Social engineering itself is primarily complicated. The most vigilant and careful user could still fall victim to the master social engineering methods, making the attacks a big issue [25,46]. These attributes of social engineering call for the organisation to set up strong policies to protect itself and its users. As some users take instructions for granted, it is more appropriate to put measures to deal with all employees who might not abide by the instructions [111]. An organisation with solid education and training system against social engineering and its attack techniques may still fall victim to the attack if there are equally not strong policies working hand in hand with the education and training system. There can be severe punishment and implementation groups for employees who might not abide by the organisation’s guidelines [112,113].
6.1.3 Emphasising Protection of Personal Privacy
The first step of a social engineering attack that includes intruding on an organisation is to gain a few seemingly valueless pieces of information, mainly personal information and documents about any of its users [46]. This information and documents may seem familiar in the world and unimportant to the individual or the organisation, but they are very relevant to a social engineer. Most users do not know why certain information and their organisation should be protected and limited [112,113]. But contrarily, social engineering attackers mostly have use for apparently disregarding information because that information is the critical element to judging whether they will be able to pretend successfully to begin the intrusion [25,46]. The organisation is responsible for informing its staff about the dangerous results their faulty administration and mismanagement of non-public information can bring to them and the organisation. Deliberate information security policies and appropriate staff training programs will significantly improve users’ awareness of legal ways of dealing with the internal information of the corporation [46].
There must be legal laws against the act for phishing activities to be entirely eradicated. When people are made to understand that you cannot do wrong and go scot-free, they might turn to put up good behaviour. Many countries release section after section of the law to fight the act [59]. In Ghana, the Cybersecurity Act 2020 (The Yellow Book) has been passed by parliament in the fight against the act. An institution (The Cybersecurity Authority) has also been set aside for the mandate of researching cybercrime. This instrument was developed after Ghana experienced a massive increase in cybercrime and cyberbullying. This institution was established to protect the country’s “critical infrastructure, regulates cybersecurity activities, provides for the protection of children on the internet, and develops Ghana’s cybersecurity ecosystem”. Ghana hopes to reduce cybercrime after setting up this institution [114]. Even though these laws and regulations will be a barrier to more casual intruders, they might not be a threat to more serious intruders [70]. For example, professional hackers will still develop new approaches to getting what they want because the act constantly evolves. Because of these, Lawmakers will have to be on their toes to make new Laws once the attackers change their approach.
6.2 Device-Level Interventions (DLIs)
There are several ways by which phishing attacks can be prevented or detected on the internet through technical means. These approaches are implemented in online applications, developed as web browser plugins or web servers. They are sometimes combined to create anti-phishing software. The following outline the technical ways or DLIs by which phishing attacks are detected and prevented on the internet.
Web content assessment methodology for detecting phishing uses approximate string-matching algorithms to determine the relationship between the content feature set of a website [10]. There are certain features that a standard website must contain [63,80]. As discussed in [115] is presented in Table 6.
Black listing is the most used of all phishing detection techniques. A blacklist is a collection of harmful websites or domains. In other words, the black listing is an approach to phish detection whereby an extensive list of collected domain names or URLs of recorded questionable or dangerous websites is maintained [74]. Users can check from the blacklist to see if the website they are being directed to is likely a phishing or harmful website. Adding a phishing website to a blacklist can cause a serious financial threat to the owner since it can drastically reduce the site’s traffic load to lower than 5% of all traffic load [75].
The prime feature that defines the effectiveness and efficiency of a blacklist can be described as how long the blacklist web application takes to update the list and the accuracy of the phishing detection mechanism used by the blacklist. To detect harmful or suspicious websites, the ways to blacklist a website are now incorporated into browser-based security tools (such as an anti-phishing toolbar or browser plug-ins). The rationale is to help prevent users from being manipulated into entering their data into unauthorised sites [70].
Websites provided on the blacklist must be added by a user or another detection mechanism which is a significant limitation. What if the user could not add or forgot to add the technique—it means that the next user could still fall victim to the attack even if they use the black list to check before taking action. Another prime limitation of the black listing is that not all phishing websites or domains are likely to be recorded in the list. The blacklist only records what it has captured. This limitation can be combated by adding other detection techniques to become a hybrid.
Webpages are identified by their uniform resource locator (URL), representing the web page’s IP address. A website URL has four essential parts. It begins with the protocol used to access the page example, HTTP and HTTPS. The registered domain name contains mainly the name of the organisation that owns the website [82]. After the registered domain name, the last part of the URL is the free URL. The free URL contains all other pages and subdomains the domain can have. The fully qualified domain name identifies the server hosting the webpage. It consists of a registered domain name and prefix, which we refer to as subdomains example: www.google.com.gh [79]. A phisher has complete control over the subdomains portion and can set it to any value. The Registered Domain Name (RDN) portion is constrained since it must be registered with a domain name registrar. The registered domain name consists of two parts: a public suffix (gh) preceded by the main level domain (com). The URL may also have path and query components, which the phisher can change. We use the term FreeURL to refer to those parts of the URL that are fully controllable by the phisher. Consider an example URL: https://www.ug.edu.gh/students/src/home. We can identify the following components and presents the examples of Protocol, Free Qualified Domain Name, Registered Domain Name, Main Level Domain and FreeURL [82]:
Protocol = https
Free Qualified Domain Name = www.ug.edu.gh
Registered Domain Name = ug.edu.gh
Main Level Domain = ug
FreeURL = {www,/gh/students/src/home}
6.2.4 Web Structure/Content Similarity
Web structure defines how the contents on a web page are put. It specifies the role, alignment, indent, paragraphs and the number of contents a particular webpage contains. It also defines how the frames, rows, and columns are positioned [79]. Intruders mostly clone authentic web pages to create their versions for their purpose. They make these clones look similar to the original, if not the same as the original. They do this so that users can find it very difficult to find the difference. The approach extracts the structure and features of the web page, decomposes them, and compares them with legitimate web pages. The method detects the web page’s document object model (DOM) tree [79].
For a user to access any web resource, a request must be placed with the URL representing the IP address of the particular web resource. A web resource could be a text, image, executable file, moving picture or sound. A logbook is created to keep track of all web requests. Therefore, a web-access log lists all web requests placed by users. The web access log provides pieces of information about the web resource. Pieces of information like IP address, authorised user’s name, date and time, status, byte, reference, user agent, and conquering port are used by Phish detecting applications. These applications use the list of these web addresses collected by the web access server and compare them with the URL in question [83]. This is to check if the URL in question has ever been requested before and how often the requests are placed [79,83,84] and others collect the weblog and apply computer vision techniques to it to detect URL phishing on the internet [20,85].
PhishTank is a data repository that collects information on phishing activities on the internet from users and stores them in a data repository. This information is the domain name if the domain is a phishing domain or a legitimate domain [76]. Phishtank allows users to report domains for being phishing domains. This is done by providing the URL of the domain detected. The platform also provides access for users to search and authenticate suspected URLs and check if they contain anything phishing or not before being used [77]. Users can copy and paste the suspected URL in a search box on PhishTank and get their authentication results. They can also reach the website an Application Programming Interface (API) provided by phish thank for developers [77,78].
Machine learning is the modelling and simulating of a real-world problem to find a solution. Many machine learning methods (convolutional neural networks, long short-term memory) have been adopted in modelling, visualising and predicting to find solutions to phishing problems [59]. Machine learning is the most popular approach to detecting phishing [61]. According to [61,70] since discovering some machine-learning algorithms, solving an email phishing problem is a matter of classification. The machine learning approach to detecting phishing activities on the internet is more flexible. Nevertheless, it is challenging to select the appropriate classification algorithm for phishing detection [10,12]. Also, social engineering is the most used cyber security threat, and phishing is its most pervasive technique [12]. They use machine learning algorithms to propose a phishing-detecting algorithm to mitigate the attacks. Reference [85] provided a new algorithm for phishing website detection using machine learning. They propose an associative algorithm called the fast association algorithm using the four well-known algorithms: Classification based on association (CBA), Classification Based on Multiple Association (CMAR), Multi Classification Rule Based on Association (MCAR), and ECAR.
This approach combines more than one of the above-listed approaches to make a new approach. This could be using two or more classification algorithms (machine learning) or more than one of the nine approaches and user interventions [86,87]. Because phishing activities are human-based attacks and constantly evolve, the existing ones are combined and made stronger against the attack instead of developing new approaches. This is the reason why the combination of the techniques is deemed necessary. Nevertheless, there seem to be few works done in this area, especially the combination involving the user side interventions and combined the convolutional neural network (CNN) and site long short-term memory (LSTM), which are machine learning classification algorithms. They used the model to visualise the act to predict and detect the attack [55,57,63].
7 Challenges and Future Directions
Because phishing attacks are human-targeted, they are challenging to detect. An organization or individual establishes all the goals and procedures for detecting and preventing phishing attack strategies. It is still up to the user to determine whether or not to comply at each stage. It is also difficult to assess the impact of user interventions. Also, how can the effectiveness, accuracy, and latency of user-level interventions be quantified? The majority of phishing detection and prevention solutions proposed by researchers are insufficient. Instead, they are either social or technical. Solutions must be derived from social (user-level interventions) and technological (device-level interventions), as both work together to make the defence mechanism effective.
Education and training are two of the most frequently recommended user-level interventions. It will be highly beneficial for organizations and individuals to understand how successful education and training are and how they contribute to phishing attack prevention and detection success. We recommend the under-listed areas that require future research directions and further study. It is expected that researchers and security experts will:
• Combine machine learning algorithm(s) with human or user-level interventions to improve phish detection.
• Focus on emerging approaches at the device and user level to provide interventions designed to detect phishing activities on the Internet.
• Adapt Machine Learning techniques on smartphones to aid device and user levels interventions to enhance phishing detection rates.
• Create phishing detectors with alert capabilities to significantly enhance phishing detection rates and prevent users from being scammed when performing transactions on the Internet.
Attackers have used phishing as a social engineering mechanism to gain vital information from users. Phishing compromises computer security, cybersecurity, and user security, whether online or offline. Several solutions have been recommended to address phishing attacks at the technological device level, but little has been done at the user level. User-level intervention remains challenging. As a result, in this technologically advanced world, appropriate educational models and relevant tools are required to provide deeper insight for the user to recognize phishing scams and similar activities readily. This article has presented a detailed study of the current state-of-the-art social engineering techniques, emphasizing phishing activities. We began by discussing human hacking as a significant issue in which people are deceived to gain their information. We discussed the many forms of social engineering attacks and offered some best practices for dealing with them.
Furthermore, we discussed phishing, indicating the impact of COVID-19 on phishing activities, trends in phishing attacks, classification of the attacks propagating, types of phishing attacks, and user behaviour characteristics. Finally, we discussed strategies for phishing attacks at both the user and device levels. Given the extensive work in social engineering, there are still numerous challenges to address before user-level phishing becomes ubiquitous.
Acknowledgement: We acknowledge contributions from colleagues who took time to go through the manuscript.
Funding Statement: The authors received no specific funding for this study.
Conflicts of Interest: The authors declare that they have no conflicts of interest to report regarding the present study.
References
1. S. Abraham and I. S. Chengalur-Smith, “An overview of social engineering malware: Trends, tactics, and implications,” Technology in Society, vol. 32, no. 3, pp. 183–196, 2010. [Google Scholar]
2. K. E. H. A. Alhosani, S. K. A. Khalid, N. A. Samsudin, S. Jamel and K. M. Bin Mohamad, “A policy-driven, human oriented information security model: A case study in UAE banking sector,” in 2019 IEEE Conf. on Application, Information and Network Security, AINS 2019, Pulau Pinang, Malaysia, 2019. [Google Scholar]
3. K. D. Mitnick and W. L. Simon, The Art of Deception: Controlling the Human Element of Security, 1st ed., Indianapolis Indiana, USA: John Wiley & Sons, pp. 13–133, 2003. [Google Scholar]
4. F. Mouton, M. M. Malan, L. Leenen and H. S. Venter, “Social engineering attack framework,” in 2014 Information Security for South Africa—Proc. of the ISSA 2014 Conf., Nanjing, China, 2014. [Google Scholar]
5. M. Edwards, R. Larson, B. Green, A. Rashid and A. Baron, “Panning for gold: Automatically analysing online social engineering attack surfaces,” Computers & Security, vol. 69, no. 1, pp. 18–34, 2017. [Google Scholar]
6. K. Krombholz, H. Hobel, M. Huber and E. Weippl, “Advanced social engineering attacks,” Journal of Information Security and Applications, vol. 22, no. 1, pp. 113–122, 2015. [Google Scholar]
7. A. Yasin, R. Fatima, L. Liu, A. Yasin and J. Wang, “Contemplating social engineering studies and attack scenarios: A review study,” Security and Privacy, vol. 2, no. 4, pp. 113, 2019. [Google Scholar]
8. M. Junger, L. Montoya and F. J. Overink, “Priming and warnings are not effective to prevent social engineering attacks,” Computers in Human Behavior, vol. 66, no. 3, pp. 75–87, 2017. [Google Scholar]
9. H. Faris and S. Yazid, “Phishing web page detection methods: URL and HTML features detection,” in IoTaIS 2020—Proc. 2020 IEEE Int. Conf. Internet Things Intelligent System, Bangalore, India, pp. 167–171, 2021. [Google Scholar]
10. K. Nagaraj, B. Bhattacharjee, A. Sridhar and G. S. Sharvani, “Detection of phishing websites using a novel twofold ensemble model,” Journal of Systems and Information Technology, vol. 20, no. 3, pp. 321–357, 2018. [Google Scholar]
11. A. Cullen and L. Armitage, “A human vulnerability assessment methodology,” in 2018 Int. Conf. Cyber Situational Awareness, Data Analytics Assessment, CyberSA 2018, Glasgow, UK, pp. 2–3, 2018. [Google Scholar]
12. A. A. Alsufyani, “Social engineering attack detection using machine learning: Text phishing attack,” Indian Journal of Computer Science and Engineering (IJCSE), vol. 12, no. 3, pp. 743–751, 2021. [Google Scholar]
13. M. Vijayalakshmi, S. M. Shalinie, M. H. Yang and U. R.M., “Web phishing detection techniques: A survey on the state-of-the-art,” Taxonomy and Future Directions, vol. 9, pp. 235–246, 2020. [Google Scholar]
14. M. Hijji and G. Alam, “A multivocal literature review on growing social engineering based cyber-attacks/threats during the COVID-19 pandemic: Challenges and prospective solutions,” IEEE Access, vol. 9, pp. 7152–7169, 2021. [Google Scholar] [PubMed]
15. Y. Kwak, S. Lee, A. Damiano and A. Vishwanath, “Why do users not report spear phishing emails?,” Telematics and Informatics, vol. 48, pp. 101343, 2020. [Google Scholar]
16. A. M. Kennedy and A. Parsons, “Macro-social marketing and social engineering: A systems approach,” Journal of Social Marketing, vol. 2, no. 1, pp. 37–51, 2012. [Google Scholar]
17. A. Suleimanov, M. Abramov and A. Tulupyev, “Modelling of the social engineering attacks based on social graph of employees communications analysis,” in 2018 IEEE Industrial Cyber-Physical Systems, ICPS 2018, St. Petersburg, Russia, 2018. [Google Scholar]
18. S. Sabouni, A. Cullen and L. Armitage, “A preliminary radicalisation framework based on social engineering techniques,” in 2017 Int. Conf. on Cyber Situational Awareness, Data Analytics and Assessment, Cyber SA 2017, London, UK, 2017. [Google Scholar]
19. A. K. Jain and B. B. Gupta, “A survey of phishing attack techniques, defence mechanisms and open research challenges,” Enterprise Information Systems, vol. 16, no. 4, pp. 527–565, 2021. [Google Scholar]
20. A. Zamir, H. U. Khan, T. Iqbal, N. Yousaf, F. Aslam et al., “Phishing web site detection using diverse machine learning algorithms,” The Electronic Library, vol. 38, no. 1, pp. 65–80, 2020. [Google Scholar]
21. A. Safi and S. Singh, “A systematic literature review on phishing website detection,” Journal of King Saud University—Computer and Information Sciences, vol. 35, no. 2, pp. 590–611, 2023. [Google Scholar]
22. A. S. Martino and X. Perramon, “Phishing secrets: History, effects, and countermeasures,” International Journal of Network Security, vol. 11, no. 3, pp. 163–171, 2010. [Google Scholar]
23. N. Sarginson, “Securing your remote workforce against new phishing attacks,” Computer Fraud & Security, vol. 2020, no. 9, pp. 9–12. [Google Scholar]
24. S. Venkatesha, K. R. Reddy and B. R. Chandavarkar, “Social engineering attacks during the COVID-19 pandemic,” SN Computer Science, vol. 2, no. 2, pp. 1–9, 2021. [Google Scholar]
25. N. Klimburg-Witjes and A. Wentland, “Hacking humans? Social engineering and the construction of the ‘deficient user’ in cybersecurity discourses,” Science Technology and Human Values, vol. 46, no. 6, pp. 1316–1339, 2021. [Google Scholar]
26. N. Saxena, E. Hayes, E. Bertino, P. Ojo, K. K. R. Choo et al., “Impact and key challenges of insider threats on organizations and critical businesses,” Electronics, vol. 9, no. 9, pp. 1–29, 2020. [Google Scholar]
27. S. Gupta, A. Singhal and A. Kapoor, “A literature survey on social engineering attacks: Phishing attack,” in IEEE Int. Conf. on Computing, Communication and Automation, ICCCA 2016, Greater Noida, India, 2017. [Google Scholar]
28. A. J. Gabriel, A. Darwsih and A. E. Hassanien, “Cyber security in the age of COVID-19,” in Digital Transformation and Emerging Technologies for Fighting COVID-19 Pandemic: Innovative Approaches, Cham, Switzerland: Springer, pp. 275–295, 2021. [Google Scholar]
29. A. Bhardwaj, V. Sapra, A. Kumar, N. Kumar and S. Arthi, “Why is phishing still successful?” Computer Fraud & Security Bulletin, vol. 2020, no. 9, pp. 15–19, 2020. [Google Scholar]
30. N. Y. Conteh and P. J. Schmick, “Cybersecurity: Risks, vulnerabilities and countermeasures to prevent social engineering attacks,” International Journal of Advanced Computer Research, vol. 6, no. 23, pp. 31–38, 2016. [Google Scholar]
31. R. A. A. Helmi, C. S. Ren, A. Jamal and M. I. Abdullah, “Email anti-phishing detection application,” in 2019 IEEE 9th Int. Conf. System Engineering and Technology, Shah Alam, Malaysia, vol. 6, pp. 264–267, 2019. [Google Scholar]
32. F. Salahdine and N. Kaabouch, “Social engineering attacks: A survey,” Future Internet, vol. 11, no. 4, pp. 89, 2019. [Google Scholar]
33. R. G. Brody, W. B. Brizzee and L. Cano, “Flying under the radar: Social engineering,” International Journal of Accounting & Information Management, vol. 20, no. 4, pp. 335–347, 2012. [Google Scholar]
34. X. Liu, Q. Li and C. Sonali, “Social engineering and insider threats,” in 2017 Int. Conf. Cyber-Enabled Distributed Computing and Knowledge Discovery, CyberC 2017, Nanjing, China, vol. 2018, pp. 25–34, 2017. [Google Scholar]
35. X. Luo, W. Zhang, S. Burd and A. Seazzu, “Investigating phishing victimization with the Heuristic-Systematic model: A theoretical framework and an exploration,” Computers & Security, vol. 38, no. 1, pp. 28–38, 2013. [Google Scholar]
36. M. P. Steves, K. K. Greene and M. F. Theofanos, “A phish scale: Rating human phishing message detection difficulty,” in Workshop on Usable Security and Privacy (USEC) 2019, San Diego, CA, USA, pp. 1–14, 2019. [Google Scholar]
37. F. Breda, H. Barbosa and T. Morais, “Social engineering and cyber security,” 2016. https://library.iated.org/ [Google Scholar]
38. P. K. Dey, “Prashant’s algorithm for password management system,” International Journal of Engineering Science Computer, vol. 6, no. 8, pp. 2424–2426, 2016. [Google Scholar]
39. D. Airehrour, N. Vasudevan Nair and S. Madanian, “Social engineering attacks and countermeasures in the New Zealand banking system: Advancing a user-reflective mitigation model,” Information, vol. 9, no. 10, pp. 110, 2018. [Google Scholar]
40. E. D. Frauenstein and S. V. Flowerday, “Social network phishing: Becoming habituated to clicks and ignorant to threats?,” in 2016 Information Security for South Africa—Proc. 2016 ISSA Conf., Johannesburg, South Africa, pp. 98–105, 2016. [Google Scholar]
41. H. Aldawood, T. Alashoor and G. Skinner, “Does awareness of social engineering make employees more secure?,” International Journal of Computer Applications, vol. 177, no. 38, pp. 45–49, 2020. [Google Scholar]
42. G. Costantino, A. La Marra, F. Martinelli and I. Matteucci, “CANDY: A social engineering attack to leak information from infotainment system,” IEEE Vehicular Technology Conference, vol. 2018, pp. 1–5, 2018. [Google Scholar]
43. S. Parekh, D. Parikh, S. Kotak and S. Sankhe, “A new method for detection of phishing websites: URL detection,” in Proc. Int. Conf. Inventive Communication and Computational Technologies, ICICCT 2018, Coimbatore, India, pp. 949–952, 2018. [Google Scholar]
44. F. Maggi, A. Volpatto, S. Gasparini, G. Boracchi and S. Zanero, “POSTER: Fast, automatic iPhone shoulder surfing,” in Proc. of ACM Conf. Computing and Communication Security, Chicago, Illinois, USA, pp. 805–807, 2011. [Google Scholar]
45. F. Twum, K. Nti and M.Asante, “Improving security levels in Automatic Teller Machines (ATM) using multifactor authentication,” International Journal of Science and Engineering Applications, vol. 5, no. 3, pp. 126–134, 2016. [Google Scholar]
46. A. S. Alazri, “The awareness of social engineering in information,” in Proc. of the 18th ACM Conf. on Computer and Communications Security (CCS'11), New York, NY, USA, Association for Computing Machinery, pp. 805–808, 2015. [Google Scholar]
47. A. Jamil, K. Asif, Z. Ghulam, M. K. Nazir, S. Mudassar Alam et al., “MPMPA: A mitigation and prevention model for social engineering based phishing attacks on Facebook,” in Proc. of 2018 IEEE Int. Conf. Big Data 2018, Seattle, WA, USA, pp. 5040–5048, 2019. [Google Scholar]
48. S. Aonzo, A. Merlo and G. Tavella, “Phishing attacks on modern android,” in Proc. of the 2018 ACM SIGSAC Conf. on Computer and Communications Security (CCS'18), New York, NY, USA, Association for Computing Machinery, pp. 1788–1801, 2018. [Google Scholar]
49. E. E. H. Lastdrager, “Achieving a consensual definition of phishing based on a systematic review of the literature,” Crime Science, vol. 3, no. 1, pp. 1–10, 2014. [Google Scholar]
50. C. Iuga, J. R. C. Nurse and A. Erola, “Baiting the hook: Factors impacting susceptibility to phishing attacks,” Human-Centric Computing and Information Sciences, vol. 6, no. 1, 2016. [Google Scholar]
51. P. Y. Leonov, A. V. Vorobyev and A. A. Ezhova, “The main social engineering techniques aimed at hacking information systems,” in Ural Symp. on Biomedical Engineering, Radioelectronics and Information Technology (USBEREIT), Yekaterinburg, Russia, pp. 471–473, 2021. [Google Scholar]
52. H. Samrat, “Library as an instrument for social engineering: The Bangladesh experience,” Frontiers of Library, Information and Computer Sciences, vol. 2, no. 2, pp. 181–185, 2016. [Google Scholar]
53. P. Activity and T. Report, “Phishing activity trends report 2 quarter,” 2021. https://apwg.org/trendsreports/ [Google Scholar]
54. D. Goel and A. K. Jain, “Mobile phishing attacks and defence mechanisms: State of art and open research challenges,” Computers & Security, vol. 73, no. 4, pp. 519–544, 2018. [Google Scholar]
55. W. Wei, Q. Ke, J. Nowak, M. Korytkowski and M. Wo, “Accurate and fast URL phishing detector: A convolutional neural network approach,” Computer Networks, vol. 178, pp. 107275, 2020. [Google Scholar]
56. J. Soyemi and M. Hammed, “An enhanced authentication scheme for preventing phishing attacks on Whatsapp accounts,” in Proc. of 2nd Int. Conf., Ogun State, Nigeria, pp. 102–108, 2020. [Google Scholar]
57. Y. Fang, C. Zhang, C. Huang, L. Liu and Y. Yang, “Phishing email detection using improved RCNN model with multilevel vectors and attention mechanism,” IEEE Access, vol. 7, pp. 56329–56340, 2019. [Google Scholar]
58. M. Rajab, “Visualisation model based on phishing features,” Journal of Information and Knowledge Management, vol. 18, no. 1, pp. 1–17, 2019. [Google Scholar]
59. F. Feng, Q. Zhou, Z. Shen, X. Yang, L. Han et al., “The application of a novel neural network in the detection of phishing websites,” Journal of Ambient Intelligence and Humanized Computing, pp. 1–15, 2018. https://doi.org/10.1007/s12652-018-0786-3 [Google Scholar] [CrossRef]
60. S. C. Jeeva and E. B. Rajsingh, “Intelligent phishing URL detection using association rule mining,” Human-Centric Computing and Information Sciences, vol. 6, no. 1, 2016. https://doi.org/10.1186/s13673-016-0064-3 [Google Scholar] [CrossRef]
61. O. K. Sahingoz, E. Buber, O. Demir and B. Diri, “Machine learning based phishing detection from URLs,” Expert Systems with Applications, vol. 117, no. 4, pp. 345–357, 2019. [Google Scholar]
62. G. Sonowal and K. S. Kuppusamy, “MMSPhiD: A phoneme based phishing verification model for persons with visual impairments,” Information & Computer Security, vol. 26, no. 5, pp. 613–636, 2018. [Google Scholar]
63. M. A. Adebowale, K. T. Lwin and M. A. Hossain, “Intelligent phishing detection scheme using deep learning algorithms,” Journal of Enterprise Information Management, vol. 36, no. 3, pp. 747–766, 2020. [Google Scholar]
64. E. D. Frauenstein and S. Flowerday, “Susceptibility to phishing on social network sites: A personality information processing model,” Computers & Security, vol. 94, no. 1, pp. 101862, 2020. [Google Scholar]
65. J. Eeffects, E. Appeals and P. Susceptiblity, “Effects of emotional appeals on phishing susceptibility,” pp. 1–15, 2020. https://aisel.aisnet.org/wisp2019/16/ [Google Scholar]
66. K. K. Adu and E. Adjei, “The phenomenon of data loss and cyber security issues in Ghana,” Foresight, vol. 20, no. 2, pp. 150–161, 2018. [Google Scholar]
67. R. Sabillon, J. Serra-Ruiz, V. Cavaller and J. Cano, “A comprehensive cybersecurity audit model to improve cybersecurity assurance: The cybersecurity audit model (CSAM),” in Proc. of 2017 Int. Conf. on Information Systems and Computer Science, vol. 2017, pp. 253–259, 2018. [Google Scholar]
68. D. B. Resnik and P. R. Finn, “Ethics and phishing experiments,” Science Engineering Ethics, vol. 24, pp. 1241–1252, 2017. [Google Scholar] [PubMed]
69. H. Shahbaznezhad, F. Kolini, M. Rashidirad and H. Shahbaznezhad, “Employees’ behavior in phishing attacks: What individual, organizational, and technological factors matter?,” Journal of Computer Information Systems, vol. 61, no. 6, pp. 539–550, 2020. https://doi.org/10.1080/08874417.2020.1812134 [Google Scholar] [CrossRef]
70. R. Alabdan, “Phishing attacks survey: Types, vectors, and technical approaches,” Future Internet, vol. 12, no. 10, pp. 168, 2020. https://doi.org/10.3390/fi12100168 [Google Scholar] [CrossRef]
71. M. Evans, L. A. Maglaras, Y. He and H. Janicke, “Human behaviour as an aspect of cybersecurity assurance,” Security and Communication Networks, vol. 9, pp. 4667–4679, 2016. [Google Scholar]
72. J. H. Cho, H. Cam and A. Oltramari, “Effect of personality traits on trust and risk to phishing vulnerability: Modeling and analysis,” in 2016 IEEE Int. Multi-Disciplinary Conf. on Cognitive Methods in Situation Awareness and Decision Support, CogSIMA 2016, San Diego, CA, pp. 7–13, 2016. [Google Scholar]
73. C. C. Campbell, “Solutions for counteracting human deception in social engineering attacks,” Information Technology & People, vol. 32, no. 5, pp. 1130–1152, 2019. [Google Scholar]
74. R. M. Mohammad, F. Thabtah and L. Mccluskey, “ScienceDirect tutorial and critical analysis of phishing websites methods,” Computer Science Review, vol. 17, no. 12, pp. 1–24, 2015. [Google Scholar]
75. I. Qabajeh, F. Thabtah and F. Chiclana, “A recent review of conventional vs. automated cybersecurity anti-phishing techniques,” Computer Science Review, vol. 29, no. 3, pp. 44–55, 2018. [Google Scholar]
76. S. Bell and P. Komisarczuk, “An analysis of phishing blacklists: Google safe browsing, OpenPhish, and PhishTank,” Pervasive Health Pervasive Computing Technology Healthcare, pp. 1–11, 2020. [Google Scholar]
77. M. H. Alkawaz, S. J. Steven and A. I. Hajamydeen, “Detecting phishing website using machine learning,” in 2020 16th IEEE Int. Colloquium on Signal Processing & its Application, CSPA 2020, Langkawi, Malaysia, pp. 111–114, 2020. [Google Scholar]
78. B. B. G. Aakanksha, T. Ankit, K. Jain and D. P. Agrawal, “Fighting against phishing attacks: State of the art and future challenges,” Neural Computing Application, vol. 28, pp. 3629–3654, 2016. [Google Scholar]
79. S. Tanaka, T. Matsunaka, A. Yamada and A. Kubota, “Phishing site detection using similarity of website structure,” in 2021 IEEE Conf. Dependable Secure Computing, DSC 2021, Aizuwakamatsu, Fukushima, Japan, 2021. [Google Scholar]
80. M. Moghimi and A. Y. Varjani, “New rule-based phishing detection method,” Expert Systems with Applications, vol. 53, pp. 231–242, 2016. [Google Scholar]
81. R. S. Rao and S. T. Ali, “A computer vision technique to detect phishing attacks,” in 2015 Fifth Int. Conf. on Communication Systems and Network Technologies, Gwalior, India, pp. 596–601, 2015. https://doi.org/10.1109/CSNT.2015.68 [Google Scholar] [CrossRef]
82. S. Marchal, K. Saari, N. Singh and N. Asokan, “Know your phish: Novel techniques for detecting phishing sites and their targets,” in 2016 IEEE 36th Int. Conf. on Distributed Computing Systems (ICDCS), Nara, Japan, 2016. [Google Scholar]
83. D. Tripathi, B. Nigam and D. R. Edla, “A novel web fraud detection technique using association rule mining,” Procedia Computer Science, vol. 115, no. 2, pp. 274–281, 2017. [Google Scholar]
84. R. Kumar, K. Garg and V. Kumar, “Extraction of frequent patterns from web logs using web log mining techniques,” International Journal of Computer Applications, vol. 59, no. 10, pp. 19–25, 2012. [Google Scholar]
85. W. Hadi, F. Aburub and S. Alhawari, “A new fast associative classification algorithm for detecting phishing websites,” Applied Soft Computing Journal, vol. 48, no. 1, pp. 729–734, 2016. [Google Scholar]
86. M. Dadkhah, S. Shamshirband and A. W. A. Wahab, “A hybrid approach for phishing web site detection,” The Electronic Library, vol. 34, no. 6, pp. 927–944, 2016. [Google Scholar]
87. T. Peng, I. Harris and Y. Sawa, “Detecting phishing attacks using natural language processing and machine learning,” in 2018 IEEE 12th Int. Conf. on Semantic Computing (ICSC), Laguna Hills, CA, USA, pp. 300–301, 2018. https://doi.org/10.1109/ICSC.2018.00056 [Google Scholar] [CrossRef]
88. X. D. Hoang and T. H. Nguyen, “Detecting common web attacks based on supervised machine learning using web logs,” Journal of Theoretical and Applied Information Technology, vol. 99, no. 6, pp. 1339–1350, 2021. [Google Scholar]
89. A. Odeh, A. Alarbi, I. Keshta and E. Abdelfattah, “Efficient prediction of phishing websites using multilayer perceptron (MLP),” Journal of Theoretical and Applied Information Technology, vol. 98, no. 16, pp. 3353–3363, 2020. [Google Scholar]
90. R. Wash, “How experts detect phishing scam emails,” in Proc. of the ACM on Human-Computer Interaction, vol. 4, no. CSCW2, pp. 1–28, 2020. [Google Scholar]
91. H. Siadati, T. Nguyen, P. Gupta, M. Jakobsson and N. Memon, “Mind your SMSes: Mitigating social engineering in second factor authentication,” Computer Security, vol. 65, pp. 14–28, 2016. [Google Scholar]
92. C. Marforio, R. J. Masti, C. Soriente, K. Kostiainen and S. Capkun, “Personalized security indicators to detect application phishing attacks in mobile platforms,” arXiv:1502.06824, 2015. https://doi.org/10.48550/arXiv.1502.06824 [Google Scholar] [CrossRef]
93. K. Parsons, M. Butavicius, P. Delfabbro and M. Lillie, “Predicting susceptibility to social influence in phishing emails,” International Journal of Human-Computer Studies, vol. 128, pp. 17–26, 2019. [Google Scholar]
94. D. Oliveira, H. Rocha, H. Yang, D. Ellis, S. Dommaraju et al., “Dissecting spear phishing emails for older vs young adults: On the interplay of weapons of influence and life domains in predicting susceptibility to phishing,” in Proc. of the 2017 CHI Conf. on Human Factors in Computing Systems, 2017. https://doi.org/10.1145/3025453.3025831 [Google Scholar] [CrossRef]
95. T. Halevi, J. Lewis and N. Memon, “Phishing, personality traits and facebook,” arXiv:1301.7643, 2013. https://doi.org/10.48550/arXiv.1301.7643 [Google Scholar] [CrossRef]
96. J. Bullee, L. Montoya, M. Junger and P. Hartel, “Spear phishing in organisations explained,” Information and Computer Security, vol. 25, no. 5, pp. 593–613, 2017. [Google Scholar]
97. Z. Benenson, F. Gassmann and R. Landwirth, “Unpacking spear phishing susceptibility,” Financial Cryptography and Data Security, vol. 1, pp. 610–627, 2017. [Google Scholar]
98. A. K. Sood and R. J. Enbody, “Malvertising—exploiting web advertising,” Computer Fraud & Security, vol. 2011, no. 4, pp. 11–16, 2011. [Google Scholar]
99. J. Reichel, F. Peck, M. Inaba, B. Moges, B. S. Chawla et al., “I have too much respect for my elders: Understanding South African mobile users’ perceptions of privacy and current behaviors on Facebook and WhatsApp,” in Proc. 29th USENIX Security Symp., Pittsburgh, PA, USA, pp. 1949–1966, 2020. [Google Scholar]
100. T. Vidas, E. Owusu, S. Wang, C. Zeng, L. F. Cranor et al., “QRishing: The susceptibility of smartphone users to QR code phishing attacks,” in Financial Cryptography and Data Security, pp. 52–69, 2013. [Google Scholar]
101. A. Das, S. Baki, A. El Aassal, R. Verma and A. Dunbar, “SoK: A comprehensive reexamination of phishing research from the security perspective,” IEEE Communications Surveys and Tutorials, vol. 22, no. 1, pp. 671–708, 2019. [Google Scholar]
102. R. Chelliah, S. Wei, E. B. M. Daliri, M. Rubab, F. Elahi et al., “Development of nanosensors based intelligent packaging systems: Food quality and medicine,” Nanomaterials, vol. 11, no. 6, pp. 1515, 2021. [Google Scholar] [PubMed]
103. M. A. Rader, S. Shawon and M. Rahman, “Exploring historical and emerging phishing techniques and mitigating the associated security risks,” International Journal of Network Security & its Applications, vol. 5, no. 4, pp. 23–41, 2013. [Google Scholar]
104. R. Wash and M. M. Cooper, “Who provides phishing training? Facts, stories, and people like me,” in Proc. of the 2018 CHI Conf. on Human Factors in Computing Systems (CHI'18), New York, NY, USA, Association for Computing Machinery, pp. 1–12, 2018. https://doi.org/10.1145/3173574.3174066 [Google Scholar] [CrossRef]
105. E. C. Tupes and R. E. Christal, “Recurrent personality factors based on trait ratings,” Journal of Personality, vol. 60, no. 2, pp. 225–251, 1992. [Google Scholar] [PubMed]
106. T. Halevi, J. Lewis and N. Memon, “A pilot study of cyber security and privacy related behavior and personality traits,” in WWW' 2013 Companion—Proc. of the 22nd Int. Conf. World Wide Web, New York, NY, USA, pp. 737–744, 2013. [Google Scholar]
107. S. Adalı and J. Golbeck, “Predicting personality with social behavior: A comparative study,” Social Network Analysis and Mining, vol. 4, no. 1, pp. 1–20, 2014. [Google Scholar]
108. F. Hassandoust, H. Singh and J. Williams, “The role of contextualization in users’ vulnerability to phishing attempts,” Australasian Journal of Information, vol. 24, pp. 1–32, 2020. [Google Scholar]
109. I. Gulenko, “Social against social engineering: Concept and development of a Facebook application to raise security and risk awareness,” Information Management & Computer Security, vol. 21, no. 2, pp. 91–101, 2013. [Google Scholar]
110. A. Jain, H. Tailang, H. Goswami, S. Dutta, M. S. Sankhla et al., “Social engineering: Hacking a human being through technology,” IOSR Journal of Computer Engineering, vol. 18, no. 5, pp. 94–100, 2016. [Google Scholar]
111. M. Volkamer, K. Renaud and P. Gerber, “Spot the phish by checking the pruned URL,” Information & Computer Security, vol. 24, no. 4, pp. 372–385, 2016. [Google Scholar]
112. M. Workman, “A test of interventions for security threats from social engineering,” Information Management & Computer Security, vol. 16, no. 5, pp. 463–483, 2008. [Google Scholar]
113. M. Junger, L. Montoya and F. Overink, “Computers in human behavior priming and warnings are not effective to prevent social engineering attacks,” Computers in Human Behavior, vol. 66, no. 3, pp. 75–87, 2017. [Google Scholar]
114. Ghana, “Cybersecurity act passed to promote & regulate cybersecurity activities | Ministry of communications,” 2020. http://csa.gov.gh/ [Google Scholar]
115. R. S. Rao and S. T. Ali, “PhishShield: A desktop application to detect phishing webpages through heuristic approach,” Procedia Computer Science, vol. 54, no. 4, pp. 147–156, 2015. [Google Scholar]
Cite This Article
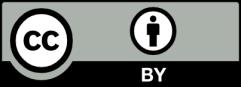