Open Access
ARTICLE
Investigating of Classification Algorithms for Heart Disease Risk Prediction
1 City University of Science and Information Technology, Peshawar, 25000, Pakistan
2 Radiology Science Department College of Applied Medical Science, Najran University, Kingdom of Saudi Arabia
3 Department of Electrical Engineering, University of Engineering and Technology, Mardan, 23200, Pakistan
4 Computer Science Department, College of Computer Science and Information System, Najran University, Saudi Arabia
5 Anatomy Department, Medicine College, Najran University, Saudi Arabia
* Corresponding Author: Bilal Khan. Email:
Journal of Intelligent Medicine and Healthcare 2022, 1(1), 11-31. https://doi.org/10.32604/jimh.2022.030161
Received 19 March 2022; Accepted 01 May 2022; Issue published 14 June 2022
Abstract
Prognosis of HD is a complex task that requires experience and expertise to predict in the early stage. Nowadays, heart failure is rising due to the inherent lifestyle. The healthcare industry generates dense records of patients, which cannot be managed manually. Such an amount of data is very significant in the field of data mining and machine learning when gathering valuable knowledge. During the last few decades, researchers have used different approaches for the prediction of HD, but still, the major problem is the uncertainty factor in the output data and also there is a need to reduce the error rate and increase the accuracy of evaluation metrics for HDP. However, this study largess the comparative analysis of diverse classification algorithms going on two different heart disease datasets taken from the Kaggle repository and University of California, Irvine (UCI) machine learning repository to find the best solution for HDP. Going through comparative analysis, ten classifiers; LR, J48, NB, ANN, SC, Bagging, DS, AdaBoost, REPT, and SVM are evaluated using MAE, RAE, precision, recall, f-measure, and accuracy. The overall finding indicates that for the dataset taken from UCI, the SVM classifier performs well as compared to other classifiers in terms of increasing accuracy and reducing error rate that is 33.2631 for RAE, and 0.165 for MAE, 0.841 for precision, 0.835 for recall, 0.833 for f-measure and 83.49% for accuracy. Whereas for dataset taken from Kaggle, the SC performs well in terms of increasing accuracy and reducing error rate that is 3.30% for RAE, 0.016 for MAE, 0.984 for precision, 0.984 for recall, 0.984 for f-measure, and 98.44% for accuracy.Keywords
Cite This Article
N. Mary, B. Khan, A. A Asiri, F. Muhammad, S. Alqhtani et al., "Investigating of classification algorithms for heart disease risk prediction," Journal of Intelligent Medicine and Healthcare, vol. 1, no.1, pp. 11–31, 2022. https://doi.org/10.32604/jimh.2022.030161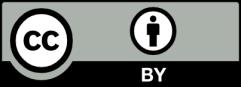