Open Access
REVIEW
A broad overview of genotype imputation: Standard guidelines, approaches, and future investigations in genomic association studies
GMLab, Department of Neurosciences, Biomedicine and Movement Sciences, University of Verona, Verona, 37134, Italy
* Corresponding Authors: MIRKO TRECCANI. Email: ; GIOVANNI MALERBA. Email:
(This article belongs to the Special Issue: Bioinformatics Study of Diseases)
BIOCELL 2023, 47(6), 1225-1241. https://doi.org/10.32604/biocell.2023.027884
Received 19 November 2022; Accepted 16 February 2023; Issue published 19 May 2023
Abstract
The advent of genomic big data and the statistical need for reaching significant results have led genome-wide association studies to be ravenous of a huge number of genetic markers scattered along the whole genome. Since its very beginning, the so-called genotype imputation served this purpose; this statistical and inferential procedure based on a known reference panel opened the theoretical possibility to extend association analyses to a greater number of polymorphic sites which have not been previously assayed by the used technology. In this review, we present a broad overview of the genotype imputation process, showing the most known methods and presenting the main areas of interest, with a closer look to the most up-to-date approaches and a deeper understanding of its usage in the present-day genomic landscape, shedding a light on its future developments and investigation areas.Keywords
Genotype imputation is a process to statistically infer missing genotypes in target samples using local linkage disequilibrium patterns from a reference panel of phased haplotypes. It is used in modern genome-wide association studies (GWAS) to extend the number of genetic variants from a set of genotyping microarrays.
Imputation was first used on genetically isolated human populations to identify ancestral haplotypes shared among samples (Pilia et al., 2006; Scuteri et al., 2007). Following the improvement of sequencing technologies, genotype imputation was then applied to several research studies, focused on human genetic diseases and complex traits (Everest et al., 2022; Kember et al., 2022).
Genotype imputation consists of the application of the homonym statistical technique for statistically measuring the genotype in terms of allele dosage or genotype likelihood probabilities. It reconstructs missing genotypes from a sample of genotyped individuals at many markers, leveraging on a large supportive dataset of fully characterized haplotypes, known as a reference panel. Imputation algorithms have been greatly improved over time and now they can handle reference panels containing millions of individuals who underwent whole genome sequencing.
Regarding the human genome, it is noteworthy that more than 715′081′156 short sequence variants and 7′097′115 structural variants (https://www.ensembl.org/Homo_sapiens/Info/Annotation) (Cunningham et al., 2022) have been reported so far. These represent most of the so-called genetic variability of human populations, and a part of them is likely to be associated with the variability of rare and common traits. Therefore, it would be very helpful to have tools able to predict the genotype at these polymorphic sites. This task could be accomplished using the proper reference panels and efficient imputation algorithms.
Imputation is routinely applied to samples that underwent genotyping microarray methodologies. Genotyping arrays carry probes for hundreds of thousands (or millions) of genetic loci widespread throughout the human genome; therefore, genotyping array–based studies cannot assay the whole set of polymorphic sites scattered across the whole genome: for this reason, the reconstruction of missing genetic loci turns out to be fundamental to enrich downstream analyses and increase the chance to detect true associations.
Thus, since its first applications, genotype imputation increased the number of significant results on either Mendelian or complex diseases (Li et al., 2006; Scott et al., 2007; Mijatovic et al., 2012). Genotype imputation was not only restricted to empower GWAS, but also to confirm or correct genotyped markers based on their computed probabilities (Marchini and Howie, 2010), to fine-map variants and confirm low evidence of association (Orho-Melander et al., 2008) and combine multiple studies into meta-analyses (Zeggini et al., 2008). With the improvements in genotyping technologies and the advent of the sequencing era, genotype imputation was effective also in the context of the genotyping of highly polymorphic regions, such as the major histocompatibility complex (MHC) region, the human leukocyte antigen (HLA, chromosome 6p) system (Meyer and Nunes, 2017; Naito and Okada, 2022) and the genomic regions coding for immunoglobulin chains (on chromosome 2 for kappa light chain, chromosome 22 for lambda light chain and chromosome 14 for the heavy chain) (McBride et al., 1982a, 1982b), to study low-quality sources, such as ancient DNA (Razali et al., 2021), and to investigate extremely rare variants (Sazonovs and Barrett, 2018).
In this review, we present an overview of genotype imputation, focusing on its application in human genomics, illustrating the major players of this inferential process, showing the main methods and approaches to obtain good-quality and reliable imputed data, and deeply exploring the main areas of investigation and research. Finally, we preview the future of genotype imputation, pointing out its major limitations and the challenges that have been overcome and that imputation is facing in the genomic big data and next-generation sequencing era.
At present, imputation is becoming a common habit in several research designs, representing a standard procedure in different pipelines for genomic analyses. However, imputation has significantly got far from its original conception, broadening its scope and applications.
The possibility to predict haplotypes and, therefore, alleles of ungenotyped variant loci arose from the fundamental concept in genetics that individuals of a given population share the haplotype stretches that have descended from common ancestors. This is evident when investigating the transmission of alleles into families (Malerba et al., 2000). Siblings share a higher number of identical stretches of DNA, deriving from parents, than a randomly chosen group of individuals. Therefore, individuals having a common ancestor are expected to share haplotype stretches whose extent depends on the temporal distance (number of recombination events throughout generations) between the individuals and the common ancestor.
The idea that individuals share identical genetic stretches (defined as haplotypes) led researchers to explore and implement imputation in genetic studies: genetic similarities among individuals suggested they could share the same ancestral haplotype and hence this turned out to be effective in genotype reconstruction of missing genotypes in related individuals. Since imputation is based on the linkage disequilibrium structure of a given genomic region, it is important to phase the alleles at different close variant loci which is the process of addressing marker alleles to either maternal or paternal haplotypes. This task can be challenging in unrelated individuals whereas it could be easier when performing family-based studies to infer genotype distribution in pedigrees and test genetic models of inheritance for traits and diseases (George and Elston, 1987; Fulker et al., 1999).
The theoretical imputation process
The general procedure behind imputation, originally developed on pedigrees, was based on three main steps: (1) some samples belonging to the same family were genotyped on several thousands (or hundreds of thousands) of markers distributed across the genome, (2) information on the haplotype to which these markers belonged were arranged and collected and (3) untyped markers of the remaining samples (of the same family) were inferred, recognizing the shared haplotypes with the fully characterized individuals (Fig. 1). The whole process was entitled “in silico genotyping” (Burdick et al., 2006), most likely because of its ability to predict genotypes from missing genetic information (Fig. 1).
Figure 1: Genotype imputation process. On top (A), two distinct phased haplotypes (Phased Haplotype 1 and Phased Haplotype 2) from a single individual are reported with missing genetic data. The middle section (B) shows the reference panel, made up of several haplotypes (colored lines). The phased haplotypes (see A) are compared with each haplotype of the reference panel (see B); genotype imputation infers the missing regions from the matching haplotypes of the reference panel (see B). Results (C) show the inferred haplotypes. The first haplotype has been inferred as the product of recombination of the yellow and blue haplotypes of the reference panel; the second haplotype has been inferred as the product of recombination of the pink, green and red haplotypes of the reference panel. For both haplotypes, the missing information has been fulfilled with different levels of certainty.
Moving towards unrelated individuals
Sooner, research interest in imputation moved to samples of unrelated individuals, which mostly build up the core of case-control studies. Compared to pedigrees, unrelated individuals did not share long haplotypes (as in the case of family members), but shorter regions that, in any case, could still be identical-by-descent, because of the presence of common ancestors, albeit far distant in time. Because a direct, informative comparison was not possible, as in the case of consanguineous individuals, the imputation process needed to be slightly revised from its original design. Specifically, (1) a catalog of detailed haplotypes for the genotyping of many individuals was set (reference panel); (2) then, phasing of close markers and comparison of phased haplotypes with the detailed haplotypes of the reference panel was performed, and (3) finally missing genotypes were predicted based on the haplotypes of the reference panel matching the phased haplotypes of the sample set.
The analysis of unrelated samples started moving the focus of imputation from close little groups of related samples, at most made of tens of individuals (Marchani et al., 2012; Chen et al., 2013), to higher amounts of individuals, not necessarily related, and sharing at a certain point similar genetic information, the so-called reference panel (Browning and Browning, 2016). The modern imputation finally began. Today, in the big data and next-generation sequencing era, genotype imputation relies on enormous reference panels, made of hundreds of thousands of samples and millions of genome-wide markers (McCarthy et al., 2016). Since the reference panels derive from individuals belonging to many ethnic groups, imputation may be conducted using the proper reference panels, even though it is not still clear what is their most effective structure (for example, haplotypes from the world-wide population or from the population having the same ethnicity of the tested samples) (Roshyara et al., 2016; Degenhardt et al., 2019; Kowalski et al., 2019). To handle these data, fast and efficient algorithms, as well as powerful and performant computational resources, are constantly improved, to keep up with the pace of present-day needs.
Genotype imputation can be summarized as a comparative process between a sample set and a known target set aimed at filling missing gaps in the sample set using the information retrieved in the target set. Here, we define as “sample set” the input dataset, made of individuals who have been sparsely genotyped along the genome and as “reference panel” the set of individuals (haplotypes) who have been assayed at the genome level and are used as the template to impute retrieved information in the sample set.
The sample set is commonly made of tens or hundreds of individuals who have been genotyped for some thousands of markers along the whole genome. The reference panel, instead, is a set of several tens or hundreds of thousands of individuals who have been genotyped at the genomic level for several hundreds of thousands or millions of loci. In the reference panel, the genetic information of all the markers is well characterized and, for this reason, it acts as the template for comparisons with the sample set. Indeed, the typed markers in the sample set are matched with the markers stored in the reference panel; the aim of this comparison is to find matches between groups of markers, the haplotypes. Hence, the matching markers are used as seeds to reconstruct in every sample of the sample set the genetic information (or genotypes) of the surrounding markers.
A new perspective: Probabilistic genotypes
Following the proposed workflow, imputation can infer intervals of imputed variants surrounded by stretches of genotyped markers; imputed variants are generated from the comparison between the sample set and the reference panel. However, this inference does not generate results having absolute certainty: imputed markers show a variable level of uncertainty, depending on several factors (see section Factors affecting imputation), and for this reason, cannot be represented as standard genotypes. Indeed, each imputed marker is reported not as a discrete genotype but as a genotype probability (GP), that is to say giving two alleles 0 and 1, the probability of an individual in the sample set of being homozygous for the reference allele (0/0), heterozygous (0/1), and homozygous for the alternative allele (1/1). To summarize these probabilistic values, imputed data are mostly represented in terms of allelic dosage (DS), a value between 0 and 2; allelic dosage, or dosage, reports for every marker the number of alternative (or risk) alleles carried by a single individual in the sample set. Usually, a dosage equal to 0 corresponds to a probability of 1 of being homozygous for the reference allele, a dosage equal to 1 to a probability of 1 of being heterozygous and a dosage of 2 of being homozygous for the alternative alleles. Because the allelic dosage is a continuous value, all the possible values between 0 and 2 represent a probability of being closer to one of the three playing genotypes.
The advent of genotype imputation boosted an immediate development of a great variety of tools and methodologies, to facilitate the management if the increasing computational load. Imputation software was initially clustered into two main categories (Li et al., 2009): computationally intensive, like IMPUTE (Marchini et al., 2007; Howie et al., 2009) and MACH (Scott et al., 2007), which took into account every genotyped marker when imputing each missing genotypes and computationally efficient, like PLINK (Purcell et al., 2007; Chang et al., 2015) and BEAGLE (Browning and Browning, 2007), which focused on neighboring markers when imputing each missing genotypes.
So far, among the many methods which have been developed to achieve good imputation results, the implementation based on Hidden Markov Models (HMMs, Stephens and Donnelly, 2000) outperforms all the other approaches. Most, if not all, of current imputation methods, rely on HMMs: despite the different algorithmic implementations, most imputation software shows similar performances (Marchini and Howie, 2010). The main features which mostly differentiate imputation methods regard the way to handle the reference panels and data storage compression, the input data and the computed output values, and method-specific implementations, like the ability to perform phasing and imputation in one or two steps and the possibility to tweak parameters and set exclusion criteria (Spencer et al., 2009).
Present-day imputation approaches
The first software implementations were extremely intensive and required a huge amount of computational power, memory, and data storage. During the past years, the methods underwent considerable improvements, becoming faster, more efficient, and more effective in every step of the genotype imputation procedure. Today, software implementation allows to impute not only bi-allelic SNPs but also multi-allelic variants (either SNPs or small INDELs); recent studies pointed out that all previously developed tools performed well on bi-allelic variants, but only new generation tools can handle multi-allelic variants, producing reliable results (Hanks et al., 2022). Indeed, current genotype imputation software is mostly categorized into two significantly different methods: population-based imputation (PBI) and family-based imputation (FBI) (Liu et al., 2019). The PBI methods are the most used and well-established approaches for genotype imputation; they usually rely on a reference panel of unrelated samples and make use of linkage disequilibrium and correlation between close SNPs of a specific population to predict ungenotyped markers. On the other hand, FBI methods are used to infer genotypes of related individuals; they rely on familial information, such as pedigrees and identity by descent, to reconstruct allelic phases and infer unobserved genotypes (Saad and Wijsman, 2014a). Different studies investigated the strengths and weaknesses of these methods (Saad and Wijsman, 2014b; Liu et al., 2019; Ullah et al., 2019). PBI showed to be particularly suitable for the investigation of common variants (frequency greater than 5%), having a higher imputation accuracy when the investigated sample set is closely related to the population of the reference panel; moreover, the size of the reference panel showed to increase imputation accuracy for rarer variants (frequency lower than 5%), probably due to their higher frequencies in a larger dataset. On the contrary, FBI methods are widely used for the imputation of rare variants, due to their increased frequencies in small and inbred populations, such as in families, but seemed to perform poorly on common variants; however, the use of study-specific reference panel seems to increase imputation accuracy for common variants, probably due to higher precision in the haplotypes of local reference samples (Liu et al., 2019; Whalen et al., 2019). For all these reasons, hybrid approaches have been explored (Liu et al., 2013; Kreiner-Møller et al., 2015; Lent et al., 2016), to make good use of the advantages of the two strategies, showing a considerable increase in genotype imputation performance (Ullah et al., 2019). Of note, nowadays most used and standard software are Beagle 5.4 (Browning et al., 2018, 2021), Eagle 2.4.1 (Loh et al., 2016, 2016) and SHAPEIT4 (Delaneau et al., 2019) for phasing and Beagle 5.4 (Browning et al., 2018, 2021), IMPUTE5 (Rubinacci et al., 2020) and Minimac4 (Das et al., 2016) for population-based imputation, and GIGI (Cheung et al., 2013), Merlin (Burdick et al., 2006) and cnF2freq (Nettelblad, 2012) for family-based imputation. In the end, the most promising hybrid approaches, taking advantage of both PBI and FBI methods, are Fimpute (Sargolzaei et al., 2014), and the combination of IMPUTE2 with Merlin (Liu et al., 2019) and GIGI with Beagle (Saad and Wijsman, 2014a).
Online platform for imputation
The constant increase of computational load, mostly related to the number of input markers and samples and the size of the reference panels, pointed out the need for powerful infrastructure to perform heavy computational tasks. Two free next-generation genotype imputation servers have been developed, respectively the Michigan Imputation Server and the TOPMed Imputation Server (Das et al., 2016). The platforms consist of a user-friendly interface in which the user, upon registration, can input their sample data (as a gzipped variant call format, one for each chromosome), setting the desired parameters like choosing standard reference panels (such as the 1000 Genomes Project phase 3, the Haplotype Reference Consortium panel and the TOPMed r2 panel; see section Reference panels) and an optional subpopulation, quality filtering to apply on the imputed data (see section Quality filtering for good imputation results), and finally choosing to perform phasing, imputation or both the two procedures sequentially. Both Michigan and the TOPMed Imputation Servers use Eagle 2.4 and Minimac4 to perform phasing and imputation, respectively. Although the combination of Eagle 2.4 and Minimac4 was assayed as one of the slowest approaches when compared to other software (such as Beagle 5), it turned out to be the most efficient in terms of computational resources for every size of the input sample set (Browning et al., 2018), representing the most suitable solution for online queued platforms.
The computational load of imputation
In general, genotype imputation requires high amounts of time and resources. State-of-the-art tools and algorithms have boosted performances, not only in managing big data but also in lowering the running time and computational load. Moreover, computation time and sample size are indirectly related: per-sample running time to perform imputation increases together with the decrease in sample size. This counterintuitive procedure is related to the fixed computational cost of reading the reference panel, which is shared among all the samples (Browning et al., 2018). Thus, the imputation procedure focuses on groups rather than on single individuals; this overview is confirmed not only from a constitutive perspective (that is, the need to have a group of individuals in both the sample and the reference panels, to clarify as much as possible the frequencies of the haplotypes in the working dataset), but also from a computational perspective.
In the last couple of years, with the advent of cloud computing services (such as Amazon Web Services, Microsoft Azure, or Google Cloud), imputation pipelines started to be optimized on pre-defined and suited virtual machines, in order to dramatically reduce the cost of imputation: a recent study pointed out the possibility to perform imputation at a cost lower than 1 U.S. cent per sample (Browning et al., 2018). The fact that imputation has been implemented in the context of a global computation indicates how much it is a fundamental tool in the most current frontier research.
Beyond the imputation software used, another key player is the reference panel. The reference panel was originally defined as a collection of individuals typed at a dense set of SNPs (Li et al., 2009). With the advancements in genotyping and sequencing technologies, it has been possible to type several hundreds of thousands of individuals for hundreds of thousands or millions of genetic loci at the genome-wide level that can be included in the reference panels (Fig. 2).
Figure 2: The most relevant reference panels for the imputation of human populations. The picture shows the relevant features (number of markers, samples, and ethnicity), and the year of release of the most important reference panels for genome-wide imputation of human samples. The blue box reports the worldwide human population samples included into the different panels.
A brief history of reference panels
Historically, the first reference panels for genotype imputation came from international consortia: the International HapMap project and the 1000 Genomes Project. Both projects aimed to characterize the genetic variability of human populations.
The main differences among them were the number of samples and genetic markers available as well as the number of represented human populations and ethnicities. In chronological order, the first two panels were the HapMap phase I (International HapMap Consortium, 2005) and phase II (International HapMap Consortium et al., 2007) which comprised 269 samples across four populations (Yoruba in Ibadan, Japanese in Tokyo, Han Chinese in Beijing and U.S. Utah residence with northern and western Europe ancestry) on 1 and 3 million of SNPs, respectively. With the spreading of first- and second-generation sequencing technologies, the 1000 Genomes Project (1000 Genomes Project Consortium et al., 2010) came to light with its phase I (1000 Genomes Project Consortium et al., 2012) and phase III (1000 Genomes Project Consortium et al., 2015) panels, comprising 2504 individuals from 26 populations for over 88 million variants. In 2016, the 1000 Genomes Project established itself as the standard resource for genomic analysis, superseding the HapMap panels. However, in the last five years, alternative and more powerful resources have been developed: a clear example is the Trans-Omics for Precision Medicine (TOPMed) reference panel (Das et al., 2016), made of around 180 thousand sequenced individuals (60% of non-European ancestry) from more than 85 different studies for a total of 308 million variants (version r2).
Populations in the reference panels
One of the main features and concerns regarding the reference panels is the structure of populations and/or subpopulations included in the reference panel. The presence of a reference panel equipped with a reliable reference population, having a high number of markers and individuals (and hence, a good picture of the most common haplotypes across the population), is a fundamental step for good quality imputation results (see section Factors affecting imputation). During the comparison of samples of both unphased sample set and reference panel, genotype imputation can lead to stronger results when the two sets are highly similar (i.e., having a similar haplotype structure); on the contrary, when the genetic background of the sample and reference sets is quite distant, imputation is committed to a dramatical increasing of false and/or weak signals. As a rule, in order to increase the accuracy of imputation calls, a consensus between the ancestries of the sample and reference sets is fundamental (de Marino et al., 2022), but a suitable reference panel is not always available. Indeed, recent studies, which focused on sample sets of mixed ancestries or low-represented subpopulations, pointed out the limits of present-day reference panels. For this reason, several researchers (Kowalski et al., 2019; O’Connell et al., 2021) tried to manage this issue by setting reference panels including individuals from different populations to ideally mimic the ancestries of the mixed populations. As a result, careful study designs together with a combination of reference populations from different ancestries seemed to be one of the most feasible approaches to overcome the bias of low-represented populations.
The probabilistic nature of genotype imputation does not permit to have absolute certainty of imputed calls. Studies on genotype imputation all agreed on several factors affecting imputation quality and results (Johnson et al., 2013; Khankhanian et al., 2015; Shi et al., 2018; Geibel et al., 2021a; Zhang et al., 2021). The most known causes are due to the technology used for sample genotyping, the number of markers and the minor allele frequency (MAF) values in either the sample set or the reference panel and the selected reference population.
Genotyping technology (arrays or sequencing) plays a crucial role in imputation performances. Since each genotyping platform spans differently along the genome, it also determines the different sets of markers that would be inferred by the imputation process. The technology impacts the number of variant sites that can be tested (Hanks et al., 2022). Moreover, not only the sample set but also the reference panels are affected by the genotyping techniques. Depending on how the reference panel has been generated (setup of variant sites included), imputation might achieve different results (i.e., only the variant sites present in the reference panel can undergo imputation). Panels of hundreds of thousands of markers widespread throughout the genome and representing different genomic locations (for example, regions having different genomic complexity) positively affect imputation, guaranteeing good-quality results. On the contrary, panels having low SNP density or a small population size would negatively impact imputation results (Das et al., 2018).
Imputation is also influenced by minor allele frequencies (the allele at a variant site having the lower frequency). Common variants (>5%), which are usually well represented across reference panels and present enough in sample sets, are smoother to impute than rarer variants. Moreover, imputation of low frequency (1%–5%) and rare (<1%) variants faces several limitations (Yu et al., 2022). Imputation can just infer known genetic information, which is stored in the reference panel; low-frequent to ultrarare (<0.1%) variants are usually not much represented in reference panels and, for this reason, would be inferred with low probabilities, resulting in unreliable calls (Zhang et al., 2021). Rare variants are in strict dependency on the chosen reference population: rare allelic frequencies are most of the time specific to a precise population, and the usage of a population not too close to the sample set may trigger a huge number of false positives (Hanks et al., 2022). However, several approaches have been developed to increase imputation accuracy for rarer variants, such as using imputation methods that combine population-based and family-based approaches (see section Present-day imputation approaches) and the usage of specific reference panels (see section Reference populations).
Despite the need for a reference panel, reference populations can backfire on imputed results. The choice of an accurate and sample-specific reference panel is crucial to ensure good quality results. However, a perfect match between sampled and reference individuals is only theoretically possible due to the large number of admixed samples in modern-day human populations (Hanks et al., 2022). Thus, the lower amount of matches between samples and reference set may increase the levels of uncertainty in imputed genotype calls, prominently for admixed populations. However, the application of state-of-the-art methodologies, together with a fine phasing of genetic data and a deeper analysis of the genetic background of samples, may mitigate population biases, generating reliable imputed results (de Marino et al., 2022). Several studies (Lin et al., 2018; Vergara et al., 2018; Bai et al., 2019) have investigated how imputation accuracy is affected by the presence of different human populations in the reference panel when analyzing underrepresented ethnic groups. The increasing distance between the sample set and the reference population negatively affects the imputation accuracy of common (>5%) and low-frequent variants (>1%); however, a small fraction of diversity in the reference panel seems to improve imputation performance in rare variants (<1%): examples have been reported for Han Chinese (Bai et al., 2019), Hawaiians (Lin et al., 2020), Turkish (Kars et al., 2021) and Latin Americans (Jiménez-Kaufmann et al., 2022). Nevertheless, genotype imputation of non-European samples still represents a challenging task, due to the predominance of European samples in most reference panels. To answer this need, several research projects started developing reference panels tailored to underrepresented populations: notable examples are the CAAPA reference panel for African Americans (O’Connell et al., 2021) (developed by the homonymous Consortium on Asthma among African-ancestry Populations in the Americas) (Mathias et al., 2016), the GAsP reference panel for Asian (GenomeAsia100K Consortium, 2019) (developed by the homonymous Genome Asia Pilot project together with the GenomeAsia 100K Project) and the ChinaMAP for Chinese (Li et al., 2021).
Finer factors affecting imputation
Other than the main factors affecting imputation quality, researchers identified finer and more specific parameters that need to be considered as a source of unreliable results: recombination rate, GC content, the distance between genotyped markers, the presence of structural variants and segmental duplications. Among these factors, a higher recombination rate, a lower GC content, the presence of structural variants, and segmental duplications have been found to be associated with lower-quality imputation results (Hanks et al., 2022), showing consistency and persistency across every method.
Quality Filtering for Good Imputation Results
In order to overcome spurious results and obtain good quality imputed data, several filtering thresholds for pre-imputation and post-imputation quality controls are commonly applied.
Before imputation, genotyped samples undergo several exclusion criteria (Li et al., 2009; de Marino et al., 2022; Hanks et al., 2022) to remove individuals having low call rates (<95%) and high missingness (>2%), significantly deviating from the Hardy-Weinberg Equilibrium (p-value < 10e-6) and duplicates. Moreover, allele labeling (reporting allele names referring to the same DNA strand for all the variant sites and all the sample sets, including the reference panel) between sampled markers and reference panel is checked, to avoid any comparison mistake, the harbinger of unreal mismatches or wrong strand reading (as for the markers where the two alleles are either A and T or C and G). In the end, samples are analyzed for their genetic background. Individuals are assayed through principal component analysis (PCA) for their genetic origins, to investigate the real ancestral background and to choose the most suitable reference panel for imputation.
To assay imputation quality and reliability, it is necessary to perform post-imputation quality control. Three main methods have been developed to assess imputation outcomes: concordance between genotypes, imputation quality score (IQS) and correlation between best-guessed genotypes (defined as R-squared or R2).
Assessing concordance between real and imputed genotypes represents a method to determine imputation quality. For every marker, genotype imputation infers a probabilistic value (in terms of genotype probabilities or alternative allele dosage), representing the probability of a genotype of being homozygous for the reference (0/0) or the alternative (1/1) allele or heterozygous (0/1). The approximation of genotype probabilities to a discrete genotypic value is far to be reliable and may lead to a higher level of wrong calls. Indeed, such an approximative method was previously tested (Abo et al., 2012) and benchmarked (Marchini and Howie, 2010) in early imputation studies, but was soon abandoned in favor of more functional metrics (de Marino et al., 2022).
Rather than approximating the probabilistic information to discrete genotypes, a more reliable way to evaluate imputation is to look at genotype probabilities directly. The first metric which was developed (Lin et al., 2010) was the imputation quality score (IQS). IQS discriminates between well-imputed and poorly imputed SNPs based on the genotype posterior probabilities. This metric compares the proportion of agreement between the haplotype-based and randomly imputed genotypes, weighted by the allelic frequencies of the imputed marker. Early usage of this metric showed remarkable improvement compared to the evaluation of the concordance approach (reported above), but since it is strongly dependent on allele frequency values, it is not suitable for rare variant sites.
Thus, the latest developed and most used metric is the R-squared (R2), defined as the correlation between the variance of the marker estimated from the counts of individual imputed alleles for every sample and the expected variance estimated from the overall allele frequencies. Hence, R2 is generally expressed as the ratio between the variance of the imputed alleles probabilities divided by the variance of the same alleles if they were perfectly imputed. In detail, R2 has been conceived in at least two different ways. Methods like MACH and Minimac express R2 values as the best approximation between the observed dosage variants on the expected dosage variants; instead, methods like Beagle and IMPUTE calculate the R2 values as the best approximation between the most likely genotype and the true unobserved expected allelic dosage (Chanda et al., 2012; Ramnarine et al., 2015).
Since its very first usage on pedigrees inference and resolution (George and Elston, 1987), genotype imputation has become a standard procedure for downstream analysis pipelines in routinary genomic studies. In the last decades, genotype imputation usage had exponential growth, thanks to both numerous advancements in genotyping and sequencing technologies together with the improvement in algorithms and methods, the empowerment of computational infrastructures, and the public availability of genetic and genomic data. Furthermore, imputation helped discover a new power in genotyped data, enriching their genetic information from their missingness. For all these reasons, genotype imputation is continuously applied to different genomic branches, such as data enrichment for genome-wide association studies, research harmonization and meta-analysis, analysis of regions of great complexity (such as MHC and HLA) or markers with rare or ultra-rare allelic frequencies. Indeed, recent studies pointed out the potential of genotype imputation in low coverage whole-genome sequencing (lcWGS) data, to confirm or discover rare and ultra-rare variants but also to provide insights on our genetic history, with the most recent application on ancient DNA (aDNA).
A Successful Combination: Genome-Wide Association Studies Empowered by Imputed Data
Genome-wide association studies are one of the most powerful techniques adopted to increase the chances of discovering novel variants and associated genes for a wide variety of traits and diseases. A large number of individuals are genotyped at a great number of polymorphic sites (ideally all the polymorphic sites of the human genome; realistically for hundreds of thousands or a few million markers), and then every locus is tested individually for the association within the trait under investigation. When, for a given polymorphic site, an allele is observed to be more common in affected individuals than in healthy individuals, the allele is reported as associated with the investigated trait or disease. This kind of analysis can be conducted at the allele or genotype level by testing for the association on all the sampled polymorphic sites (Fig. 3).
Figure 3: Genotype imputation in a genome-wide association study (GWAS) workflow.
Data sampling: DNA samples and phenotypes are collected according to different designs.
Genotyping: samples are genotyped using either genotyping arrays or next-generation sequencing technologies (WGS: whole-genome sequencing; WES: whole-exome sequencing).
Data quality control: quality controls aim to filter out genotypes that do not meet the inclusion criteria, to not hamper imputation quality.
Haplotype phasing and imputation: unphased genotypes (GT) are compared to the known haplotypes of the reference panel, to infer the underlying haplotypes and therefore missing information; imputed data (Imputation output) show the phased genotypes (GT) together with the probabilistic representations in term of allelic dosage (DS) and genotype probabilities (GP), estimated by the comparison of the unphased genotypes and the reference haplotypes.
Data quality control: low-quality imputed data are filtered out (Final imputation output).
Association tests: final output undergoes association analysis for the investigated phenotype (GWAS) (Turner, 2018).
For several years, GWASs based solely on genotyped samples which were at most assayed for tens of thousands of markers, a small portion if compared to our genome. The increasing interest in genotype imputation and the early work on related and unrelated individuals showed researchers that combining the discovery potential of GWAS together with the ability of imputation to infer genetic data from missing information would have led to an increase in the statistical power of genomic scans (Quick et al., 2020). Early evidence of this successful combination was pointed out in diseases, such as type 2 diabetes in the Finnish population (Scott et al., 2007) and familial cases affected by multiple sclerosis (Dyment et al., 2008), and in complex traits (Newton-Cheh et al., 2009), identifying novel loci explaining genetic susceptibility, risk, or sample variance.
The possibility of combining genetic information coming from genotyping techniques together with probabilistic inferred data, immediately revealed the strengths and weaknesses of this approach. On the one hand, enriching typed data for a great number of markers that have not been previously genotyped allowed to reach reliable association signals and to confirm previously reported evidence, as for psoriasis (Nair et al., 2009) but also to identify novel loci of understudied diseases, as for eosinophilic granulomatosis with polyangiitis (Lyons et al., 2019). However, on the other hand, the methods available to study genetic signals did not support the probabilistic perspective generated in the imputation process. Approximating genotype probabilities or allelic dosages to genotype was firmly discouraged (Li et al., 2009), and for this reason, novel methodologies had to be developed.
Tools to study associations on imputed data
To overcome this initial bias in managing different representations of genetic data, several models that were able to account for probabilities and to handle uncertainty were implemented and integrated into association pipelines (Marchini and Howie, 2010). One of the first developed and probably most used models is the frequentist model. This model compares for every SNP a pre-determined model of association (such as additive, dominant, recessive, and others) against a model of no association, considering the genetic information as a likelihood probability. The frequentist model was immediately implemented since the earliest snptest release (Marchini et al., 2007; Wellcome Trust Case Control Consortium, 2007). However, the model faced immediate problems regarding spurious results, mostly due to small sample sizes, low allelic frequencies, and high levels of uncertainty in imputation calls (Lu et al., 2010). Novel approaches were developed to overcome these issues, mostly based on the Bayesian statistical method (Stephens and Balding, 2009). As for the frequentist model, Bayesian methods as well compare a model of association against a model of no association but implement a more complex and parametrized modeling. Despite the similarities and differences between these two approaches, genetic data which underwent imputation had to be considered as probabilistic, either in terms of genotype probabilities or allelic dosage, to ensure robust and unbiased association signals as demonstrated in several studies spanning from computational benchmarking (Song et al., 2018; Jørsboe and Albrechtsen, 2022) to genomic analyses investigating either disorder (Vissers et al., 2019) or traits (Tan et al., 2019).
Study Harmonization and Meta-Analysis
The ability of genotype imputation to infer genetic data from missing values in the sample set turned out to be fundamental for the study combination. Cohorts from different research projects are rarely genotyped or sequenced with the same technologies and instruments. Thus, the absence of markers between two or more studies is a standard event, which happens because of the great variety of approaches and methodologies currently available. Moreover, a meta-analysis of genomic studies may reveal important outcomes, particularly related to complex traits and diseases. For example, a study on sepsis (Hernandez-Beeftink et al., 2022) conducted on more than six hundred samples from the GEN-SEP network (Guillen-Guio et al., 2020) was able to enrich its sampled dataset to over 7 million SNPs and combining it to thousands of individuals from the MESSI (Reilly et al., 2018); as a result, the study identified three independent low-frequency variants associated with reduced 28-day sepsis survival. A good example of data integration guided by genotype imputation is represented by research consortia. Consortia aims in integrating multiple results to empower research on a particular topic or phenotypes, as in the case of the CKDGen Consortium, an international collaboration for the genetic investigation of kidney functions in health and disease. In a study on chronic kidney disease (CKD) in children (Wuttke et al., 2016), they successfully integrated three pediatric CKD cohorts (Furth et al., 2006; ESCAPE Trial Group et al., 2009; Querfeld et al., 2010) and identified ten regions associated with creatinine clearance (GFRcrea), four regions with proteinuria and six regions with CKD.
Imputation of Highly Polymorphic Regions: The Example of the Major Histocompatibility Complex Region
The human major histocompatibility complex (MHC) region is also known as the human leukocyte antigen (HLA) complex. It maps on the short arm of chromosome 6 (6p21.3) (Choo, 2007; Douillard et al., 2021), and it is known to be the most gene-dense region along the human genome (~260 genes in ~4 Mb of length) (Trowsdale and Knight, 2013; Kennedy et al., 2017). The MHC genes can be classified into three different groups by sequence similarity and function: MHC class I, class II, and class III (Choo, 2007; Douillard et al., 2021).
A summary of the human leukocyte antigen region
The HLA complex presents the highest level of polymorphisms in human genomes (Choo, 2007). The high number of polymorphisms belonging to this region makes its genotyping quite challenging because of the continuous discoveries of new HLA alleles (Stefani et al., 2022; Naito and Okada, 2022).
All known HLA alleles are reported in the IPD-IMGT/HLA database (Robinson et al., 2013); so far, HLA-A and HLA-C present more than 4000 known alleles each, and HLA-B reports over 5000 different alleles (according to the IPD-IMGT/HLA database release 3.50). Therefore, many of these alleles have a very low frequency in the worldwide population (Cook et al., 2021). It is noteworthy that the complexity of the HLA region depends on the huge number of variants mapping in the regions and that the variants sites might have multiple complex alleles (indels and/or more than one single nucleotide).
Thanks to several bioinformatic strategies, it was possible to investigate short genomic sequences coming from whole genome sequencing (WGS) or whole exome sequencing (WES), pointing out the different HLA types (Erlich, 2015). The knowledge of HLA variants and alleles makes it possible to infer HLA patterns. However, the standard WGS-based imputation estimates missing genotypes of the HLA region inaccurately (Uffelmann et al., 2021).
Association studies on the major histocompatibility complex/human leukocyte antigen region
With the advent of the Human Genome Project (Collins and Fink, 1995), the MHC region started to be unraveled, stating its complexity (Meyer and Nunes, 2017; Kennedy et al., 2017). Many GWAS for complex traits, such as cardiovascular, metabolic, and neurological diseases, have reported several associations with markers of the MHC region (Kennedy et al., 2017; Naito and Okada, 2022).
The extreme complexity of this region always makes imputation quite difficult (Uffelmann et al., 2021). Due to the high levels of linkage disequilibrium (several extended haplogroups have been identified) and the great number of polymorphisms, it is important that the imputation process can use a good reference panel containing as many haplotypes as possible (Erlich, 2015; Meyer and Nunes, 2017; Cook et al., 2021).
Imputation of the major histocompatibility complex/human leukocyte antigen region
One of the major challenges to successfully performing genotype imputation in this complex region is to find a good and detailed reference panel. Thus, panels specific to the MHC/HLA region need to be accurately fine-mapped and comprise as many samples as possible, to overcome the enormous number of polymorphisms (Cook et al., 2021). When using sequencing, reliable imputation of the HLA region does need high read (short sequences) coverage and depth to call all the variability of the HLA region (Douillard et al., 2021).
Tools for imputation of the human leukocyte antigen complex
Several tools for imputation on HLA can infer missing HLA genotypes based on reference datasets and/or individual SNPs (Douillard et al., 2021).
Over the years, many imputation algorithms able to tackle LD features of the MHC region were developed (Meyer and Nunes, 2017; Naito and Okada, 2022). All the algorithms for HLA imputation are based on probabilistic approaches. The most known and used are HLA*IMP (Dilthey et al., 2011), specific to the European population, which was subsequently improved in HLA*IMP:02 (Dilthey et al., 2013), able to handle multiple populations; indeed, SNP2HLA (Jia et al., 2013), based on Beagle, was implemented to impute not only the HLA-specific alleles but also the related aminoacidic sequence; finally, CookHLA (Cook et al., 2021) improved SNP2HLA algorithm, accounting better for linkage disequilibrium. Due to the complexity of studying HLA regions with imputed data, researchers developed HLA-TAPAS (Luo et al., 2021), a three-in-one solution for reference panel construction, imputation, and association studies on HLA genetic data.
A reference panel for human leukocyte antigen imputation
As for all the genome regions and populations, imputation accuracy is influenced by the choice of the reference panel. At first, the HLA-specific reference panel was built for a single ancestry, considering the haplotype structures (due to the linkage disequilibrium in the region) of the European population (Dilthey et al., 2011). Moreover, the advent of NGS technologies boosted the construction of larger HLA-specific reference panels, leading to a more accurate investigation between HLA genotypes and diseases (Naito and Okada, 2022). Most recent research suggested that using multi-ethnic reference panels (Luo et al., 2021) could guarantee a more accurate genotype imputation (Meyer and Nunes, 2017; Douillard et al., 2021; Naito and Okada, 2022). A multi-ethnic reference panel was built from a high-coverage WGS dataset (Luo et al., 2021) and made available on the Michigan Imputation Server, together with an optimized procedure for HLA imputation. However, the accuracy and the sensitivity of the method are a major concern, as mixed populations can still lead to inaccurate results even if better than the ones using the standard reference panels (Douillard et al., 2021).
Imputation of Low-Coverage Sequencing Regions
In the last two decades, a number of different genotyping arrays, based on different sets of polymorphic sites, have been used for several investigations, including the assessment of genetic distance (i.e., similarity studies) between individuals or populations, and GWAS for complex traits. It is known that when polymorphic site data are not obtained from a random sample of polymorphisms, the results of genetic studies can be distorted because of ascertainment biases (Geibel et al., 2021b). In this regard, genotype imputation improves the statistical power of GWAS (Das et al., 2018, Geibel et al., 2021a).
Compared to the whole set of possible detectable genotypes in the genome, the arrays can assay only a relatively small subset of pre-selected and fixed polymorphic sites, allowing the calling of low-density array-based genotypes.
With the emerging genotyping-by-sequencing technology, marker discovery and genotyping occur simultaneously, resulting in minimal ascertainment bias and therefore improving the accuracy of the genetic studies (i.e., genetic-based similarity, genotype imputation, and association studies) (Heslot et al., 2013).
However, despite the advancements in sequencing technologies and platforms, a whole genome sequencing-based study on several thousands of samples is still prohibitively expensive for many laboratories. In general, genotype imputation is used together with very large reference panels to increase the number of accurately imputed variants. To carry out a quality imputation, it is necessary to have certain attention, according to the cases under investigation. It may be possible that no large reference panels exist for the studied population or that the reference panel contains a too small number of individuals.
It has been suggested that to improve the accuracy of imputation on small sample sets of individuals and on low-frequency variants, it is crucial to maximize the genetics similarity between the sample set and the reference panel and to include in the reference panel as many polymorphic sites as possible (Korkuć et al., 2019). Moreover, in smaller populations, determining the optimal imputation strategy would be extremely challenging when high-density genotypes are not available. In non-human populations (for instance, cattle or poultry), one of the most used strategies is to sequence a subset of a population that is employed as the reference panel to perform genotype imputation with high accuracy (Jiang et al., 2022). Of note, according to the context, the parameters of the imputation software must be carefully fine-tuned, and the metrics to assess imputation quality (either in terms of reliability or accuracy) must be interpreted with caution, specifically if the genetic distance between the sample set and reference population is elevated (Roshyara and Scholz, 2015).
As a rule, it is recommended to use a large reference panel (better if individuals present a very small genetic distance from individuals of the investigated population) or, if not possible, to use genetically best-matched reference panels in which the individuals are of the same ethnicity of the individuals of the studied population. Larger reference panels are associated with higher computational costs and longer computation times for phasing and imputation. The need for new and more efficient software is therefore very compelling in this field today.
Although it may sound bizarre, imputation can be used not only within genotyping array-based studies but also in sequencing-based association studies. Among the various possible employment in the context of sequencing, it has been shown that imputation can be a very useful tool in the sequencing of low-coverage genomes and in the case of off-target regions from exome sequencing. Imputation was reported to be an efficient tool on extremely low-coverage sequencing (0.1–0.5x) data to capture almost as much of the common (>5%) and low-frequency (1%–5%) variation across the genome with results that are very similar to the ones from classical SNP arrays, in many different ethnic groups (Pasaniuc et al., 2012). In this context, it was also shown that imputation can be used to impute variants mapping in the off-target regions of exome sequencing to some extent to the whole genome. Indeed, imputation on exome sequencing data is still under investigation; the first evidence showed that regions with ~3000 rare and common variants per 1 megabase, result in good quality imputed data. More interestingly, low coverage WGS (lcWGS) appears now to be an alternative technology to genotyping arrays for common genetic variant assessment in the context of genome-wide polygenic scores (PGS) calculation (Homburger et al., 2019). Indeed, lcWGS proved to have brilliant performance in imputation results and accuracy, showing comparable capabilities to genotyping array and overcoming ascertainment bias inherent to the variant selection of genotype array.
In the last decade, genotype imputation was employed in ancient DNA (aDNA) investigation (Genome of the Netherlands Consortium, 2014). The ability of genotype imputation to infer and reconstruct genotypes of sampled individuals seemed particularly suitable for ancient samples. Indeed, DNA from ancient samples is totally subjected to degradation processes, including cross-linking, deamination, and fragmentation, which may lead to several difficulties in extraction, sequencing, and clear genotyping results (Allentoft et al., 2012). Although genotype imputation is capable of enriching samples with novel imputed variants, the technical difficulties in obtaining clear genetic signals as well as the unavailability of a specific reference panel for an ancient human genome, challenged modern methodologies and techniques. Due to the great uncertainty that aDNA analyses constantly face, new strategies seemed necessary. Low-coverage ancient DNA sequencing seemed to mitigate the quality issues of ancient samples; thus, it was a suitable method to deal with the probabilistic representation of genotypes, the core of the imputation process (Ausmees et al., 2022).
To achieve good quality results, the great majority of research on aDNA made use of low coverage (2X) and ultra-low coverage (0.5X–1X) genotyping and sequencing strategies combined with phasing and genotype imputation methods (Pasaniuc et al., 2012), particularly using Beagle4 and Beagle5 (Hui et al., 2020). This combination of techniques turned out to be particularly successful, confirming genomic shifts and fluxes across ages in Hungarian samples from the Neolithic, Copper, Bronze, and Iron Ages (Gamba et al., 2014) and investigating ancient population substructures in the Portuguese population from the Neolithic to the Bronze Age (Martiniano et al., 2017).
Furthermore, several strategies were implemented to overcome reference bias. The absence of an ancient reference panel, whose development has become of great interest in the most recent research (Ausmees et al., 2022; Biddanda et al., 2022), forced the usage of reference panels made of modern-day samples. A two-step strategy was implemented on a modern reference panel using Beagle software, which demonstrated to achieve good results of imputation on low-coverage samples (Hui et al., 2020). This two-step procedure works as follow: (1) imputation is performed on a reference panel as similar as possible to the sample set, in term of genetic background and admixture; in this first step, the size of the reference panel is not taken into account; then, (2) the computed genotype likelihoods are added to the sample set and compared to a larger worldwide reference panel. Applications of the approach have been demonstrated to be successful, for example, in the fine estimation of Iberian population ancestries (Villalba-Mouco et al., 2019).
Past Achievements and Future Challenges
Genotype imputation is becoming increasingly common in genomic research, being nowadays part of the standard pipelines for genome-wide association studies. Since the very first approach of imputation to genetic data, several applications have been hypothesized, and numerous questions and challenges have arisen. No more than fifteen years ago, the imputation of HLA regions or the development of large reference panels constituted enormous limitations that may have stopped researchers from using genotype imputation in the genomic investigation.
Thanks to the advancements in genotyping and sequencing techniques as well as in computational implementation, imputation has become capable of combining cohorts to deeply investigate traits and diseases through the usage of meta-analysis and empowering genome-wide association studies by enriching samples with new untyped markers to discover newly associated variants and genes involved in traits and diseases.
Furthermore, the decreasing genotyping and sequencing costs permitted the genotyping of several hundreds of thousands of either markers or individuals. This enormous boost in the amount of genetic data enabled researchers to build up a larger reference panel, which could represent world-wide populations and carry information on both common and rare variants. However, population and subpopulation representation remain biased, particularly in terms of low-represented ancestries and ultra-rare variants, which may be of crucial relevance for determining risks related to modern-day diseases and for modeling polygenic risk scores. To overcome reference limitations, several recent studies proposed the combination of low-coverage whole genome sequencing and imputation and the integration of imputed samples into reference panels to lower per-sample costs and generate more specific and performant reference panels.
A powerful example of genotype imputation procedures is represented by the online next-generation imputation platforms, the Michigan Imputation Server and the TOPMed Imputation Server. These platforms allow standardization of phasing and genotype imputation processes, providing state-of-the-art tools and curated reference panels to answer either average or advanced needs for genotype imputation.
To sum up, genotype imputation is an impressive resource that can boost genetic and genomic research. Imputation resolutions are at the sample level; however, its perspective stands in the general sampled population as well as in the matching reference panel. The uncertainty, which is an intrinsic feature of this inferential technique, cannot be explained at the individual level but have a sense from a population perspective. Indeed, genotype probabilities and allelic dosages are a new descriptive measurement in genetics, mostly used for discrete genotype variables, and must be managed carefully using proper tools and parametrization. This specific probabilistic representation would allow better modelling of traits and disease as well as a better understanding of rare and ultra-rare variants, which may be fundamental to investigate the causes and hypothesize predictive models of present-day pathologies.
Funding Statement: The authors received no specific funding for this study.
Author Contributions: Manuscript conception and design: Mirko TRECCANI and Giovanni MALERBA; literature collection: Mirko TRECCANI, Elena LOCATELLI, Cristina PATUZZO, and Giovanni MALERBA; manuscript preparation: Mirko TRECCANI, Elena LOCATELLI, Cristina PATUZZO, and Giovanni MALERBA; realization of graphical content: Elena LOCATELLI; draft content revision: Cristina PATUZZO; manuscript revision and finalization: Giovanni MALERBA. All authors approved the final version of the manuscript.
Availability of Data and Materials: Data sharing is not applicable to this article as no datasets were generated or analyzed during the current study.
Ethics Approval: Not applicable.
Conflicts of Interest: The authors declare that they have no conflicts of interest to report regarding the present study.
References
Abo R, Hebbring S, Ji Y, Zhu H, Zeng ZB et al. (2012). Merging pharmacometabolomics with pharmacogenomics using ‘1000 Genomes’ single-nucleotide polymorphism imputation: Selective serotonin reuptake inhibitor response pharmacogenomics. Pharmacogenetics and Genomics 22: 247–253. https://doi.org/10.1097/FPC.0b013e32835001c9 [Google Scholar] [PubMed] [CrossRef]
Allentoft ME, Collins M, Harker D, Haile J, Oskam CL et al. (2012). The half-life of DNA in bone: Measuring decay kinetics in 158 dated fossils. Proceedings of the Royal Society B: Biological Sciences 279: 4724–4733. https://doi.org/10.1098/rspb.2012.1745 [Google Scholar] [PubMed] [CrossRef]
Ausmees K, Sanchez-Quinto F, Jakobsson M, Nettelblad C (2022). An empirical evaluation of genotype imputation of ancient DNA. G3 Genes/Genomes/Genetics 12: jkac089. https://doi.org/10.1093/g3journal/jkac089 [Google Scholar] [PubMed] [CrossRef]
Bai WY, Zhu XW, Cong PK, Zhang XJ, Richards JB, Zheng HF (2019). Genotype imputation and reference panel: A systematic evaluation on haplotype size and diversity. Briefing in Bioinformatics, bbz108. https://doi.org/10.1093/bib/bbz108 [Google Scholar] [PubMed] [CrossRef]
Biddanda A, Steinrücken M, Novembre J (2022). Properties of 2-locus genealogies and linkage disequilibrium in temporally structured samples. Genetics 221: iyac038. https://doi.org/10.1093/genetics/iyac038 [Google Scholar] [PubMed] [CrossRef]
Browning SR, Browning BL (2007). Rapid and accurate haplotype phasing and missing-data inference for whole-genome association studies by use of localized haplotype clustering. American Journal of Human Genetics 81: 1084–1097. https://doi.org/10.1086/521987 [Google Scholar] [PubMed] [CrossRef]
Browning BL, Browning SR (2016). Genotype Imputation with millions of reference samples. American Journal of Human Genetics 98: 116–126. https://doi.org/10.1016/j.ajhg.2015.11.020 [Google Scholar] [PubMed] [CrossRef]
Browning BL, Tian X, Zhou Y, Browning SR (2021). Fast two-stage phasing of large-scale sequence data. American Journal of Human Genetics 108: 1880–1890. https://doi.org/10.1016/j.ajhg.2021.08.005 [Google Scholar] [PubMed] [CrossRef]
Browning BL, Zhou Y, Browning SR (2018). A one-penny imputed genome from next-generation reference panels. American Journal of Human Genetics 103: 338–348. https://doi.org/10.1016/j.ajhg.2018.07.015 [Google Scholar] [PubMed] [CrossRef]
Burdick JT, Chen WM, Abecasis GR, Cheung VG (2006). In silico method for inferring genotypes in pedigrees. Nature Genetics 38: 1002–1004. https://doi.org/10.1038/ng1863 [Google Scholar] [PubMed] [CrossRef]
Chanda P, Yuhki N, Li M, Bader JS, Hartz A, Boerwinkle E, Kao WH, Arking DE (2012). Comprehensive evaluation of imputation performance in African Americans. Journal of Human Genetics 57: 411–421. https://doi.org/10.1038/jhg.2012.43 [Google Scholar] [PubMed] [CrossRef]
Chang CC, Chow CC, Tellier LC, Vattikuti S, Purcell SM, Lee JJ (2015). Second-generation PLINK: Rising to the challenge of larger and richer datasets. GigaScience 4: 7. https://doi.org/10.1186/s13742-015-0047-8 [Google Scholar] [PubMed] [CrossRef]
Chen W, Li B, Zeng Z, Sanna S, Sidore C, Busonero F, Kang HM, Li Y, Abecasis GR (2013). Genotype calling and haplotyping in parent-offspring trios. Genome Research 23: 142–151. https://doi.org/10.1101/gr.142455.112 [Google Scholar] [PubMed] [CrossRef]
Cheung CY, Thompson EA, Wijsman EM (2013). GIGI: An approach to effective imputation of dense genotypes on large pedigrees. American Journal of Human Genetics 92: 504–516. https://doi.org/10.1016/j.ajhg.2013.02.011 [Google Scholar] [PubMed] [CrossRef]
Choo SY (2007). The HLA system: Genetics, immunology, clinical testing, and clinical implications. Yonsei Medical Journal 48: 11–23. https://doi.org/10.3349/ymj.2007.48.1.11 [Google Scholar] [PubMed] [CrossRef]
Collins FS, Fink L (1995). The human genome project. Alcohol Health and Research World 19: 190–195. [Google Scholar] [PubMed]
Cook S, Choi W, Lim H, Luo Y, Kim K, Jia X, Raychaudhuri S, Han B (2021). Accurate imputation of human leukocyte antigens with CookHLA. Nature Communications 12: 1264. https://doi.org/10.1038/s41467-021-21541-5 [Google Scholar] [PubMed] [CrossRef]
Cunningham F, Allen JE, Allen J, Alvarez-Jarreta J, Amode MR et al. (2022). Ensembl 2022. Nucleic Acids Research 50: D988–D995. https://doi.org/10.1093/nar/gkab1049 [Google Scholar] [PubMed] [CrossRef]
Das S, Abecasis GR, Browning BL (2018). Genotype imputation from large reference panels. Annual Review of Genomics and Human Genetics 19: 73–96. https://doi.org/10.1146/annurev-genom-083117-021602 [Google Scholar] [PubMed] [CrossRef]
Das S, Forer L, Schönherr S, Sidore C, Locke AE et al. (2016). Next-generation genotype imputation service and methods. Nature Genetics 48: 1284–1287. https://doi.org/10.1038/ng.3656 [Google Scholar] [PubMed] [CrossRef]
de Marino A, Mahmoud AA, Bose M, Bircan KO, Terpolovsky A, Bamunusinghe V, Bohn S, Khan U, Novković B, Yazdi PG (2022). A comparative analysis of current phasing and imputation software. PLoS One 17: e0260177. https://doi.org/10.1371/journal.pone.0260177 [Google Scholar] [PubMed] [CrossRef]
Degenhardt F, Wendorff M, Wittig M, Ellinghaus E, Datta LW et al. (2019). Construction and benchmarking of a multi-ethnic reference panel for the imputation of HLA class I and II alleles. Human Molecular Genetics 28: 2078–2092. https://doi.org/10.1093/hmg/ddy443 [Google Scholar] [PubMed] [CrossRef]
Delaneau O, Zagury JF, Robinson MR, Marchini JL, Dermitzakis ET (2019). Accurate, scalable and integrative haplotype estimation. Nature Communications 10: 5436. https://doi.org/10.1038/s41467-019-13225-y [Google Scholar] [PubMed] [CrossRef]
Dilthey A, Leslie S, Moutsianas L, Shen J, Cox C, Nelson MR, McVean G (2013). Multi-population classical HLA type imputation. PLoS Computational Bioliogy 9: e1002877. https://doi.org/10.1371/journal.pcbi.1002877 [Google Scholar] [PubMed] [CrossRef]
Dilthey AT, Moutsianas L, Leslie S, McVean G (2011). HLA*IMP—An integrated framework for imputing classical HLA alleles from SNP genotypes. Bioinformatics 27: 968–972. https://doi.org/10.1093/bioinformatics/btr061 [Google Scholar] [PubMed] [CrossRef]
Douillard V, Castelli EC, Mack SJ, Hollenbach JA, Gourraud PA, Vince N, Limou S (2021). Approaching genetics through the MHC lens: Tools and methods for HLA research. Frontiers in Genetics 12: 774916. https://doi.org/10.3389/fgene.2021.774916 [Google Scholar] [PubMed] [CrossRef]
Dyment DA, Cader MZ, Herrera BM, Ramagopalan SV, Orton SM, Chao M, Willer CJ, Sadovnick AD, Risch N, Ebers GC (2008). A genome scan in a single pedigree with a high prevalence of multiple sclerosis. Journal of Neurology, Neurosurgery and Psychiatry 79: 158–162. https://doi.org/10.1136/jnnp.2007.122705 [Google Scholar] [PubMed] [CrossRef]
Erlich HA (2015). HLA typing using next generation sequencing: An overview. Human Immunology 76: 887–890. https://doi.org/10.1016/j.humimm.2015.03.001 [Google Scholar] [PubMed] [CrossRef]
ESCAPE Trial Group, Wühl E, Trivelli A, Picca S, Litwin M et al. (2009). Strict blood-pressure control and progression of renal failure in children. The New England Journal of Medicine 361: 1639–1650. https://doi.org/10.1056/NEJMoa0902066 [Google Scholar] [PubMed] [CrossRef]
Everest E, Ahangari M, Uygunoglu U, Tutuncu M, Bulbul A et al. (2022). Investigating the role of common and rare variants in multiplex multiple sclerosis families reveals an increased burden of common risk variation. Scientific Reports 12: 16984. https://doi.org/10.1038/s41598-022-21484-x [Google Scholar] [PubMed] [CrossRef]
Fulker DW, Cherny SS, Sham PC, Hewitt JK (1999). Combined linkage and association sib-pair analysis for quantitative traits. American Journal of Human Genetics 64: 259–267. https://doi.org/10.1086/302193 [Google Scholar] [PubMed] [CrossRef]
Furth SL, Cole SR, Moxey-Mims M, Kaskel F, Mak R, Schwartz G, Wong C, Muñoz A, Warady BA (2006). Design and methods of the Chronic Kidney Disease in Children (CKiD) prospective cohort study. Clinical Journal of the American Society of Nephrology 1: 1006–1015. https://doi.org/10.2215/CJN.01941205 [Google Scholar] [PubMed] [CrossRef]
Gamba C, Jones ER, Teasdale MD, McLaughlin RL, Gonzalez-Fortes G et al. (2014). Genome flux and stasis in a five millennium transect of European prehistory. Nature Communications 5: 5257. https://doi.org/10.1038/ncomms6257 [Google Scholar] [PubMed] [CrossRef]
Geibel J, Reimer C, Pook T, Weigend S, Weigend A, Simianer H (2021a). How imputation can mitigate SNP ascertainment Bias. BMC Genomics 22: 340. https://doi.org/10.1186/s12864-021-07663-6 [Google Scholar] [PubMed] [CrossRef]
Geibel J, Reimer C, Weigend S, Weigend A, Pook T, Simianer H (2021b). How array design creates SNP ascertainment bias. PLoS One 16: e0245178. https://doi.org/10.1371/journal.pone.0245178 [Google Scholar] [PubMed] [CrossRef]
Genome of the Netherlands Consortium (2014). Whole-genome sequence variation, population structure and demographic history of the Dutch population. Nature Genetics 46: 818–825. https://doi.org/10.1038/ng.3021 [Google Scholar] [PubMed] [CrossRef]
GenomeAsia100K Consortium (2019). The GenomeAsia 100K Project enables genetic discoveries across Asia. Nature 576: 106–111. https://doi.org/10.1038/s41586-019-1793-z [Google Scholar] [PubMed] [CrossRef]
Genomes Project Consortium, Abecasis GR, Altshuler D, Auton A, Brooks LD, Durbin RM, Gibbs RA, Hurles ME, McVean GA (2010). A map of human genome variation from population-scale sequencing. Nature 467: 1061–1073. https://doi.org/10.1038/nature09534 [Google Scholar] [PubMed] [CrossRef]
Genomes Project Consortium, Abecasis GR, Auton A, Brooks LD, DePristo MA, Durbin RM, Handsaker RE, Kang HM, Marth GT, McVean GA (2012). An integrated map of genetic variation from 1,092 human genomes. Nature 491: 56–65. https://doi.org/10.1038/nature11632 [Google Scholar] [PubMed] [CrossRef]
Genomes Project Consortium, Auton A, Brooks LD, Durbin RM, Garrison EP et al. (2015). A global reference for human genetic variation. Nature 526: 68–74. https://doi.org/10.1038/nature15393 [Google Scholar] [PubMed] [CrossRef]
George VT, Elston RC (1987). Testing the association between polymorphic markers and quantitative traits in pedigrees. Genetic Epidemiology 4: 193–201. https://doi.org/10.1002/gepi.1370040304 [Google Scholar] [PubMed] [CrossRef]
Guillen-Guio B, Lorenzo-Salazar JM, Ma SF, Hou PC, Hernandez-Beeftink T et al. (2020). Sepsis-associated acute respiratory distress syndrome in individuals of European ancestry: A genome-wide association study. The Lancet Respiratory Medicine 8: 258–266. https://doi.org/10.1016/S2213-2600(19)30368-6 [Google Scholar] [PubMed] [CrossRef]
Hanks SC, Forer L, Schönherr S, LeFaive J, Martins T et al. (2022). Extent to which array genotyping and imputation with large reference panels approximate deep whole-genome sequencing. American Journal of Human Genetics 109: 1653–1666. https://doi.org/10.1016/j.ajhg.2022.07.012 [Google Scholar] [PubMed] [CrossRef]
Hernandez-Beeftink T, Guillen-Guio B, Lorenzo-Salazar JM, Corrales A, Suarez-Pajes E et al. (2022). A genome-wide association study of survival in patients with sepsis. Critical Care 26: 341. https://doi.org/10.1186/s13054-022-04208-5 [Google Scholar] [PubMed] [CrossRef]
Heslot N, Rutkoski J, Poland J, Jannink JL, Sorrells ME (2013). Impact of marker ascertainment bias on genomic selection accuracy and estimates of genetic diversity. PLoS One 8: e74612. https://doi.org/10.1371/journal.pone.0074612 [Google Scholar] [PubMed] [CrossRef]
Homburger JR, Neben CL, Mishne G, Zhou AY, Kathiresan S, Khera AV (2019). Low coverage whole genome sequencing enables accurate assessment of common variants and calculation of genome-wide polygenic scores. Genome Medicine 11: 74. https://doi.org/10.1186/s13073-019-0682-2 [Google Scholar] [PubMed] [CrossRef]
Howie BN, Donnelly P, Marchini J (2009). A flexible and accurate genotype imputation method for the next generation of genome-wide association studies. PLoS Genetics 5: e1000529. https://doi.org/10.1371/journal.pgen.1000529 [Google Scholar] [PubMed] [CrossRef]
Hui R, D’Atanasio E, Cassidy LM, Scheib CL, Kivisild T (2020). Evaluating genotype imputation pipeline for ultra-low coverage ancient genomes. Scientific Reports 10: 18542. https://doi.org/10.1038/s41598-020-75387-w [Google Scholar] [PubMed] [CrossRef]
International HapMap Consortium (2005). A haplotype map of the human genome. Nature 437: 1299–1320. https://doi.org/10.1038/nature04226 [Google Scholar] [PubMed] [CrossRef]
International HapMap Consortium, Frazer KA, Ballinger DG, Cox DR, Hinds DA et al. (2007). A second generation human haplotype map of over 3.1 million SNPs. Nature 449: 851–861. https://doi.org/10.1038/nature06258 [Google Scholar] [PubMed] [CrossRef]
Jia X, Han B, Onengut-Gumuscu S, Chen WM, Concannon PJ, Rich SS, Raychaudhuri S, de Bakker PI (2013). Imputing amino acid polymorphisms in human leukocyte antigens. PLoS One 8: e64683. https://doi.org/10.1371/journal.pone.0064683 [Google Scholar] [PubMed] [CrossRef]
Jiang Y, Song H, Gao H, Zhang Q, Ding X (2022). Exploring the optimal strategy of imputation from SNP array to whole-genome sequencing data in farm animals. Frontiers in Genetics 13: 963654. https://doi.org/10.3389/fgene.2022.963654 [Google Scholar] [PubMed] [CrossRef]
Jiménez-Kaufmann A, Chong AY, Cortés A, Quinto-Cortés CD, Fernandez-Valverde SL et al. (2022). Imputation performance in Latin American populations: Improving rare variants representation with the inclusion of native American genomes. Frontiers in Genetics 12: 719791. https://doi.org/10.3389/fgene.2021.719791 [Google Scholar] [PubMed] [CrossRef]
Johnson EO, Hancock DB, Levy JL, Gaddis NC, Saccone NL, Bierut LJ, Page GP (2013). Imputation across genotyping arrays for genome-wide association studies: Assessment of bias and a correction strategy. Human Genetics 132: 509–522. https://doi.org/10.1007/s00439-013-1266-7 [Google Scholar] [PubMed] [CrossRef]
Jørsboe E, Albrechtsen A (2022). Efficient approaches for large-scale GWAS with genotype uncertainty. G3 Genes/Genomes/Genetics 12: jkab385. https://doi.org/10.1093/g3journal/jkab385 [Google Scholar] [PubMed] [CrossRef]
Kars ME, Başak AN, Onat OE, Bilguvar K, Choi J et al. (2021). The genetic structure of the Turkish population reveals high levels of variation and admixture. Proceedings of the National Academy of Sciences of the United States of America 118: e2026076118. https://doi.org/10.1073/pnas.2026076118 [Google Scholar] [PubMed] [CrossRef]
Kember RL, Vickers-Smith R, Xu H, Toikumo S, Niarchou M et al. (2022). Cross-ancestry meta-analysis of opioid use disorder uncovers novel loci with predominant effects in brain regions associated with addiction. Nature Neuroscience 25: 1279–1287. https://doi.org/10.1038/s41593-022-01160-z [Google Scholar] [PubMed] [CrossRef]
Kennedy AE, Ozbek U, Dorak MT (2017). What has GWAS done for HLA and disease associations? International Journal of Immunogenetics 44: 195–211. https://doi.org/10.1111/iji.12332 [Google Scholar] [PubMed] [CrossRef]
Khankhanian P, Din L, Caillier SJ, Gourraud PA, Baranzini SE (2015). SNP imputation bias reduces effect size determination. Frontiers in Genetics 6: 30. https://doi.org/10.3389/fgene.2015.00030 [Google Scholar] [PubMed] [CrossRef]
Korkuć P, Arends D, Brockmann GA (2019). Finding the optimal imputation strategy for small cattle populations. Frontiers in Genetics 10: 52. https://doi.org/10.3389/fgene.2019.00052 [Google Scholar] [PubMed] [CrossRef]
Kowalski MH, Qian H, Hou Z, Rosen JD, Tapia AL et al. (2019). Use of >100,000 NHLBI Trans-Omics for Precision Medicine (TOPMed) Consortium whole genome sequences improves imputation quality and detection of rare variant associations in admixed African and Hispanic/Latino populations. PLoS Genetics 15: e1008500. https://doi.org/10.1371/journal.pgen.1008500 [Google Scholar] [PubMed] [CrossRef]
Kreiner-Møller E, Medina-Gomez C, Uitterlinden AG, Rivadeneira F, Estrada K (2015). Improving accuracy of rare variant imputation with a two-step imputation approach. European Journal of Human Genetics 23: 395–400. https://doi.org/10.1038/ejhg.2014.91 [Google Scholar] [PubMed] [CrossRef]
Lent S, Deng X, Cupples LA, Lunetta KL, Liu CT, Zhou Y (2016). Imputing rare variants in families using a two-stage approach. BMC Proceedings 10: 209–214. https://doi.org/10.1186/s12919-016-0032-y [Google Scholar] [PubMed] [CrossRef]
Li M, Atmaca-Sonmez P, Othman M, Branham KE, Khanna R et al. (2006). CFH haplotypes without the Y402H coding variant show strong association with susceptibility to age-related macular degeneration. Nature Genetics 38: 1049–1054. https://doi.org/10.1038/ng1871 [Google Scholar] [PubMed] [CrossRef]
Li L, Huang P, Sun X, Wang S, Xu M et al. (2021). The ChinaMAP reference panel for the accurate genotype imputation in Chinese populations. Cell Research 31: 1308–1310. https://doi.org/10.1038/s41422-021-00564-z [Google Scholar] [PubMed] [CrossRef]
Li Y, Willer C, Sanna S, Abecasis G (2009). Genotype imputation. Annual Review of Genomics and Human Genetics 10: 387–406. https://doi.org/10.1146/annurev.genom.9.081307.164242 [Google Scholar] [PubMed] [CrossRef]
Lin M, Caberto C, Wan P, Li Y, Lum-Jones A et al. (2020). Population-specific reference panels are crucial for genetic analyses: an example of the CREBRF locus in Native Hawaiians. Human Molecular Genetics 29: 2275–2284. https://doi.org/10.1093/hmg/ddaa083 [Google Scholar] [PubMed] [CrossRef]
Lin P, Hartz SM, Zhang Z, Saccone SF, Wang J et al. (2010). A new statistic to evaluate imputation reliability. PLoS One 5: e9697. https://doi.org/10.1371/journal.pone.0009697 [Google Scholar] [PubMed] [CrossRef]
Lin Y, Liu L, Yang S, Li Y, Lin D, Zhang X, Yin X (2018). Genotype imputation for Han Chinese population using Haplotype Reference Consortium as reference. Human Genetics 137: 431–436. https://doi.org/10.1007/s00439-018-1894-z [Google Scholar] [PubMed] [CrossRef]
Liu CT, Deng X, Fisher V, Heard-Costa N, Xu H, Zhou Y, Vasan RS, Cupples LA (2019). Revisit population-based and family-based genotype imputation. Scientific Reports 9: 1800. https://doi.org/10.1038/s41598-018-38469-4 [Google Scholar] [PubMed] [CrossRef]
Liu EY, Li M, Wang W, Li Y (2013). MaCH-admix: Genotype imputation for admixed populations. Genetic Epidemiology 37: 25–37. https://doi.org/10.1002/gepi.21690 [Google Scholar] [PubMed] [CrossRef]
Loh PR, Danecek P, Palamara PF, Fuchsberger C, Reshef A et al. (2016). Reference-based phasing using the Haplotype Reference Consortium panel. Nature Genetics 48: 1443–1448. https://doi.org/10.1038/ng.3679 [Google Scholar] [PubMed] [CrossRef]
Loh PR, Palamara PF, Price AL (2016). Fast and accurate long-range phasing in a UK Biobank cohort. Nature Genetics 48: 811–816. https://doi.org/10.1038/ng.3571 [Google Scholar] [PubMed] [CrossRef]
Lu K, Jiang L, Tsiatis AA (2010). Multiple imputation approaches for the analysis of dichotomized responses in longitudinal studies with missing data. Biometrics 66: 1202–1208. https://doi.org/10.1111/j.1541-0420.2010.01405.x [Google Scholar] [PubMed] [CrossRef]
Luo Y, Kanai M, Choi W, Li X, Sakaue S et al. (2021). A high-resolution HLA reference panel capturing global population diversity enables multi-ancestry fine-mapping in HIV host response. Nature Genetics 53: 1504–1516. https://doi.org/10.1038/s41588-021-00935-7 [Google Scholar] [PubMed] [CrossRef]
Lyons PA, Peters JE, Alberici F, Liley J, Coulson RMR et al. (2019). Genome-wide association study of eosinophilic granulomatosis with polyangiitis reveals genomic loci stratified by ANCA status. Nature Communications 10: 5120. https://doi.org/10.1038/s41467-019-12515-9 [Google Scholar] [PubMed] [CrossRef]
Malerba G, Lauciello MC, Scherpbier T, Trabetti E, Galavotti R et al. (2000). Linkage analysis of chromosome 12 markers in Italian families with atopic asthmatic children. American Journal of Respiratory and Critical Care Medicine 162: 1587–1590. https://doi.org/10.1164/ajrccm.162.4.9909031 [Google Scholar] [PubMed] [CrossRef]
Marchani EE, Chapman NH, Cheung CY, Ankenman K, Stanaway IB, Coon HH, Nickerson D, Bernier R, Brkanac Z, Wijsman EM (2012). Identification of rare variants from exome sequence in a large pedigree with autism. Human Heredity 74: 153–164. https://doi.org/10.1159/000346560 [Google Scholar] [PubMed] [CrossRef]
Marchini J, Howie B (2010). Genotype imputation for genome-wide association studies. Nature Reviews Genetics 11: 499–511. https://doi.org/10.1038/nrg2796 [Google Scholar] [PubMed] [CrossRef]
Marchini J, Howie B, Myers S, McVean G, Donnelly P (2007). A new multipoint method for genome-wide association studies by imputation of genotypes. Nature Genetics 39: 906–913. https://doi.org/10.1038/ng2088 [Google Scholar] [PubMed] [CrossRef]
Martiniano R, Cassidy LM, Ó’Maoldúin R, McLaughlin R, Silva NM et al. (2017). The population genomics of archaeological transition in west Iberia: Investigation of ancient substructure using imputation and haplotype-based methods. PLoS Genetics 13: e1006852. https://doi.org/10.1371/journal.pgen.1006852 [Google Scholar] [PubMed] [CrossRef]
Mathias RA, Taub MA, Gignoux CR, Fu W, Musharoff S et al. (2016). A continuum of admixture in the Western Hemisphere revealed by the African Diaspora genome. Nature Communications 7: 12522. https://doi.org/10.1038/ncomms12522 [Google Scholar] [PubMed] [CrossRef]
McBride OW, Battey J, Hollis GF, Swan DC, Siebenlist U, Leder P (1982a). Localization of human variable and constant region immunoglobulin heavy chain genes on subtelomeric band q32 of chromosome 14. Nucleic Acids Research 10: 8155–8170. https://doi.org/10.1093/nar/10.24.8155 [Google Scholar] [PubMed] [CrossRef]
McBride OW, Hieter PA, Hollis GF, Swan D, Otey MC, Leder P (1982b). Chromosomal location of human kappa and lambda immunoglobulin light chain constant region genes. The Journal of Experimental Medicine 155: 1480–1490. https://doi.org/10.1084/jem.155.5.1480 [Google Scholar] [PubMed] [CrossRef]
McCarthy S, Das S, Kretzschmar W, Delaneau O, Wood AR et al. (2016). A reference panel of 64,976 haplotypes for genotype imputation. Nature Genetics 48: 1279–1283. https://doi.org/10.1038/ng.3643 [Google Scholar] [PubMed] [CrossRef]
Meyer D, Nunes K (2017). HLA imputation, what is it good for? Human Immunology 78: 239–241. https://doi.org/10.1016/j.humimm.2017.02.007 [Google Scholar] [PubMed] [CrossRef]
Mijatovic V, Iacobucci I, Sazzini M, Xumerle L, Mori A, Pignatti PF, Martinelli G, Malerba G (2012). Imputation reliability on DNA biallelic markers for drug metabolism studies. BMC Bioinformatics 13: S7. https://doi.org/10.1186/1471-2105-13-S14-S7 [Google Scholar] [PubMed] [CrossRef]
Nair RP, Duffin KC, Helms C, Ding J, Stuart PE et al. (2009). Genome-wide scan reveals association of psoriasis with IL-23 and NF-κB pathways. Collaborative Association Study of Psoriasis 41: 199–204. https://doi.org/10.1038/ng.311 [Google Scholar] [PubMed] [CrossRef]
Naito T, Okada Y (2022). HLA imputation and its application to genetic and molecular fine-mapping of the MHC region in autoimmune diseases. Seminars in Immunopathology 44: 15–28. https://doi.org/10.1007/s00281-021-00901-9 [Google Scholar] [PubMed] [CrossRef]
Nettelblad C (2012). Inferring haplotypes and parental genotypes in larger full sib-ships and other pedigrees with missing or erroneous genotype data. BMC Genetics 13: 85. https://doi.org/10.1186/1471-2156-13-85 [Google Scholar] [PubMed] [CrossRef]
Newton-Cheh C, Johnson T, Gateva V, Tobin MD, Bochud M et al. (2009). Genome-wide association study identifies eight loci associated with blood pressure. Nature Genetics 41: 666–676. https://doi.org/10.1038/ng.361 [Google Scholar] [PubMed] [CrossRef]
Orho-Melander M, Melander O, Guiducci C, Perez-Martinez P, Corella D et al. (2008). Common missense variant in the glucokinase regulatory protein gene is associated with increased plasma triglyceride and C-reactive protein but lower fasting glucose concentrations. Diabetes 57: 3112–3121. https://doi.org/10.2337/db08-0516 [Google Scholar] [PubMed] [CrossRef]
O’Connell J, Yun T, Moreno M, Li H, Litterman N et al. (2021). A population-specific reference panel for improved genotype imputation in African Americans. Communications Biology 4: 1269. https://doi.org/10.1038/s42003-021-02777-9 [Google Scholar] [PubMed] [CrossRef]
Pasaniuc B, Rohland N, McLaren PJ, Garimella K, Zaitlen N et al. (2012). Extremely low-coverage sequencing and imputation increases power for genome-wide association studies. Nature Genetics 44: 631–635. https://doi.org/10.1038/ng.2283 [Google Scholar] [PubMed] [CrossRef]
Pilia G, Chen WM, Scuteri A, Orru M, Albai Dei et al. (2006). Heritability of cardiovascular and personality traits in 6,148 Sardinians. PLoS Genetics 2: e132. https://doi.org/10.1371/journal.pgen.0020132 [Google Scholar] [PubMed] [CrossRef]
Purcell S, Neale B, Todd-Brown K, Thomas L, Ferreira MA et al. (2007). PLINK: A tool set for whole-genome association and population-based linkage analyses. American Journal of Human Genetics 81: 559–575. https://doi.org/10.1086/519795 [Google Scholar] [PubMed] [CrossRef]
Querfeld U, Anarat A, Bayazit AK, Bakkaloglu AS, Bilginer Y et al. (2010). The cardiovascular comorbidity in children with chronic kidney disease (4C) study: Objectives, design, and methodology. Clinical Journal of the American Society of Nephrology 5: 1642–1648. https://doi.org/10.2215/CJN.08791209 [Google Scholar] [PubMed] [CrossRef]
Quick C, Anugu P, Musani S, Weiss ST, Burchard EG et al. (2020). Sequencing and imputation in GWAS: Cost-effective strategies to increase power and genomic coverage across diverse populations. Genetic Epidemiology 44: 537–549. https://doi.org/10.1002/gepi.22326 [Google Scholar] [PubMed] [CrossRef]
Ramnarine S, Zhang J, Chen LS, Culverhouse R, Duan W et al. (2015). When does choice of accuracy measure alter imputation accuracy assessments? PLoS One 10: e0137601. https://doi.org/10.1371/journal.pone.0137601 [Google Scholar] [PubMed] [CrossRef]
Razali RM, Rodriguez-Flores J, Ghorbani M, Naeem H, Aamer W et al. (2021). Thousands of Qatari genomes inform human migration history and improve imputation of Arab haplotypes. Nature Communications 12: 5929. https://doi.org/10.1038/s41467-021-25287-y [Google Scholar] [PubMed] [CrossRef]
Reilly JP, Wang F, Jones TK, Palakshappa JA, Anderson BJ et al. (2018). Plasma angiopoietin-2 as a potential causal marker in sepsis-associated ARDS development: Evidence from Mendelian randomization and mediation analysis. Intensive Care Medicine 44: 1849–1858. https://doi.org/10.1007/s00134-018-5328-0 [Google Scholar] [PubMed] [CrossRef]
Robinson J, Halliwell JA, McWilliam H, Lopez R, Parham P, Marsh SG (2013). The IMGT/HLA database. Nucleic Acids Research 41: D1222–D1227. https://doi.org/10.1093/nar/gks949 [Google Scholar] [PubMed] [CrossRef]
Roshyara NR, Horn K, Kirsten H, Ahnert P, Scholz M (2016). Comparing performance of modern genotype imputation methods in different ethnicities. Scientific Reports 6: 34386. https://doi.org/10.1038/srep34386 [Google Scholar] [PubMed] [CrossRef]
Roshyara NR, Scholz M (2015). Impact of genetic similarity on imputation accuracy. BMC Genetics 16: 90. https://doi.org/10.1186/s12863-015-0248-2 [Google Scholar] [PubMed] [CrossRef]
Rubinacci S, Delaneau O, Marchini J (2020). Genotype imputation using the Positional Burrows Wheeler Transform. PLoS Genetics 16: e1009049. https://doi.org/10.1371/journal.pgen.1009049 [Google Scholar] [PubMed] [CrossRef]
Saad M, Wijsman EM (2014a). Power of family-based association designs to detect rare variants in large pedigrees using imputed genotypes. Genetic Epidemiology 38: 1–9. https://doi.org/10.1002/gepi.21776 [Google Scholar] [PubMed] [CrossRef]
Saad M, Wijsman EM (2014b). Combining family- and population-based imputation data for association analysis of rare and common variants in large pedigrees. Genetic Epidemiology 38: 579–590. https://doi.org/10.1002/gepi.21844 [Google Scholar] [PubMed] [CrossRef]
Sargolzaei M, Chesnais JP, Schenkel FS (2014). A new approach for efficient genotype imputation using information from relatives. BMC Genomics 15: 478. https://doi.org/10.1186/1471-2164-15-478 [Google Scholar] [PubMed] [CrossRef]
Sazonovs A, Barrett JC (2018). Rare-variant studies to complement genome-wide association studies. Annual Review of Genomics and Human Genetics 19: 97–112. https://doi.org/10.1146/annurev-genom-083117-021641 [Google Scholar] [PubMed] [CrossRef]
Scott LJ, Mohlke KL, Bonnycastle LL, Willer CJ, Li Y et al. (2007). A genome-wide association study of type 2 diabetes in Finns detects multiple susceptibility variants. Science 316: 1341–1345. https://doi.org/10.1126/science.1142382 [Google Scholar] [PubMed] [CrossRef]
Scuteri A, Sanna S, Chen WM, Uda M, Albai G et al. (2007). Genome-wide association scan shows genetic variants in the FTO gene are associated with obesity-related traits. PLoS Genetics 3: e115. https://doi.org/10.1371/journal.pgen.0030115 [Google Scholar] [PubMed] [CrossRef]
Shi S, Yuan N, Yang M, Du Z, Wang J, Sheng X, Wu J, Xiao J (2018). Comprehensive assessment of genotype imputation performance. Human Heredity 83: 107–116. https://doi.org/10.1159/000489758 [Google Scholar] [PubMed] [CrossRef]
Song M, Wheeler W, Caporaso NE, Landi MT, Chatterjee N (2018). Using imputed genotype data in the joint score tests for genetic association and gene-environment interactions in case-control studies. Genetic Epidemiology 42: 146–155. https://doi.org/10.1002/gepi.22093 [Google Scholar] [PubMed] [CrossRef]
Spencer CCA, Su Z, Donnelly P, Marchini J (2009). Designing genome-wide association studies: Sample size, power, imputation, and the choice of genotyping chip. PLoS Genetics 5: e1000477. https://doi.org/10.1371/journal.pgen.1000477 [Google Scholar] [PubMed] [CrossRef]
Stefani C, Sangalli A, Locatelli E, Federico T, Malerba G et al. (2022). Increased prevalence of HLA-C unstable variants in HIV-1 rapid progressor patients. International Journal of Molecular Sciences 23: 14852. https://doi.org/10.3390/ijms232314852 [Google Scholar] [PubMed] [CrossRef]
Stephens M, Balding DJ (2009). Bayesian statistical methods for genetic association studies. Nature Reviews Genetics 10: 681–690. https://doi.org/10.1038/nrg2615 [Google Scholar] [PubMed] [CrossRef]
Stephens M, Donnelly P (2000). Inference in molecular population genetics. Journal of the Royal Statistical Society Series B: Statistical Methodology 62: 605–635. https://doi.org/10.1111/1467-9868.00254 [Google Scholar] [CrossRef]
Tan AG, Kifley A, Flood VM, Holliday EG, Scott RJ, Cumming RG, Mitchell P, Wang JJ (2019). Evaluating the associations between obesity and age-related cataract: A Mendelian randomization study. The American Journal of Clinical Nutrition 110: 969–976. https://doi.org/10.1093/ajcn/nqz167 [Google Scholar] [PubMed] [CrossRef]
Trowsdale J, Knight JC (2013). Major histocompatibility complex genomics and human disease. Annual Review of Genomics and Human Genetics 14: 301–323. https://doi.org/10.1146/annurev-genom-091212-153455 [Google Scholar] [PubMed] [CrossRef]
Turner SD (2018). qqman: an R package for visualising GWAS results using Q-Q and manhattan plots. Journal of Open Source Software 3: 731. https://doi.org/10.21105/joss.00731 [Google Scholar] [CrossRef]
Uffelmann E, Huang QQ, Munung NS, de Vries J, Okada Y, Martin AR, Martin HC, Lappalainen T, Posthuma D (2021). Genome-wide association studies. Nature Reviews Methods Primers 1: 59. https://doi.org/10.1038/s43586-021-00056-9 [Google Scholar] [CrossRef]
Ullah E, Mall R, Abbas MM, Kunji K, Nato AQ, Bensmail H, Wijsman EM, Saad M (2019). Comparison and assessment of family- and population-based genotype imputation methods in large pedigrees. Genome Research 29: 125–134. https://doi.org/10.1101/gr.236315.118 [Google Scholar] [PubMed] [CrossRef]
Vergara C, Parker MM, Franco L, Cho MH, Valencia-Duarte AV, Beaty TH, Duggal P (2018). Genotype imputation performance of three reference panels using African ancestry individuals. Human Genetics 137: 281–292. https://doi.org/10.1007/s00439-018-1881-4 [Google Scholar] [PubMed] [CrossRef]
Villalba-Mouco V, van de Loosdrecht MS, Posth C, Mora R, Martínez-Moreno J et al. (2019). Survival of late pleistocene hunter-gatherer ancestry in the Iberian Peninsula. Current Biology 29: 1169–1177.e7. https://doi.org/10.1016/j.cub.2019.02.006 [Google Scholar] [PubMed] [CrossRef]
Vissers LET, Sluijs I, van der Schouw YT, Forouhi NG, Imamura F et al. (2019). Dairy product intake and risk of type 2 diabetes in EPIC-InterAct: A mendelian randomization study. Diabetes Care 42: 568–575. https://doi.org/10.2337/dc18-2034 [Google Scholar] [PubMed] [CrossRef]
Wellcome Trust Case Control Consortium (2007). Genome-wide association study of 14,000 cases of seven common diseases and 3,000 shared controls. Nature 447: 661–678. https://doi.org/10.1038/nature05911 [Google Scholar] [PubMed] [CrossRef]
Whalen A, Gorjanc G, Hickey JM (2019). Family-specific genotype arrays increase the accuracy of pedigree-based imputation at very low marker densities. Genetics Selection Evolution 51: 33. https://doi.org/10.1186/s12711-019-0478-2 [Google Scholar] [PubMed] [CrossRef]
Wuttke M, Wong CS, Wühl E, Epting D, Luo L et al. (2016). Genetic loci associated with renal function measures and chronic kidney disease in children: The pediatric investigation for genetic factors linked with renal progression consortium. Nephrology Dialysis Transplantation 31: 262–269. https://doi.org/10.1093/ndt/gfv342 [Google Scholar] [PubMed] [CrossRef]
Yu WY, Yan SS, Zhang SH, Ni JJ, Li B, Pei YF, Zhang L (2022). Efficient identification of trait-associated loss-of-function variants in the UK Biobank cohort by exome-sequencing based genotype imputation. Genetic Epidemiology 47: 121–134. https://doi.org/10.1002/gepi.22511 [Google Scholar] [PubMed] [CrossRef]
Zeggini E, Scott LJ, Saxena R, Voight BF, Marchini JL et al. (2008). Meta-analysis of genome-wide association data and large-scale replication identifies additional susceptibility loci for type 2 diabetes. Nature Genetics 40: 638–645. https://doi.org/10.1038/ng.120 [Google Scholar] [PubMed] [CrossRef]
Zhang Z, Xiao X, Zhou W, Zhu D, Amos CI (2021). False positive findings during genome-wide association studies with imputation: Influence of allele frequency and imputation accuracy. Human Molecular Genetics 31: 146–155. https://doi.org/10.1093/hmg/ddab203 [Google Scholar] [PubMed] [CrossRef]
Cite This Article
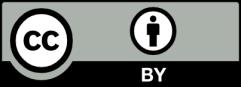