Open Access
ARTICLE
Genetic algorithm-optimized backpropagation neural network establishes a diagnostic prediction model for diabetic nephropathy: Combined machine learning and experimental validation in mice
1 Division of Nephrology, Renmin Hospital of Wuhan University, Wuhan, China
2 Nephrology and Urology Research Institute of Wuhan University, Wuhan, China
3 The First College of Clinical Medical Science, Three Gorges University, Yichang, China
4 School of Life Science and Engineering, Southwest Jiaotong University, Chengdu, China
* Corresponding Author: Wei Liang,
(This article belongs to the Special Issue: Bioinformatics Study of Diseases)
BIOCELL 2023, 47(6), 1253-1263. https://doi.org/10.32604/biocell.2023.027373
Received 27 October 2022; Accepted 11 January 2023; Issue published 19 May 2023
Abstract
Background: Diabetic nephropathy (DN) is the most common complication of type 2 diabetes mellitus and the main cause of end-stage renal disease worldwide. Diagnostic biomarkers may allow early diagnosis and treatment of DN to reduce the prevalence and delay the development of DN. Kidney biopsy is the gold standard for diagnosing DN; however, its invasive character is its primary limitation. The machine learning approach provides a non-invasive and specific criterion for diagnosing DN, although traditional machine learning algorithms need to be improved to enhance diagnostic performance. Methods: We applied high-throughput RNA sequencing to obtain the genes related to DN tubular tissues and normal tubular tissues of mice. Then machine learning algorithms, random forest, LASSO logistic regression, and principal component analysis were used to identify key genes (CES1G, CYP4A14, NDUFA4, ABCC4, ACE). Then, the genetic algorithm-optimized backpropagation neural network (GA-BPNN) was used to improve the DN diagnostic model. Results: The AUC value of the GA-BPNN model in the training dataset was 0.83, and the AUC value of the model in the validation dataset was 0.81, while the AUC values of the SVM model in the training dataset and external validation dataset were 0.756 and 0.650, respectively. Thus, this GA-BPNN gave better values than the traditional SVM model. This diagnosis model may aim for personalized diagnosis and treatment of patients with DN. Immunohistochemical staining further confirmed that the tissue and cell expression of NADH dehydrogenase (ubiquinone) 1 alpha subcomplex, 4-like 2 (NDUFA4L2) in tubular tissue in DN mice were decreased. Conclusion: The GA-BPNN model has better accuracy than the traditional SVM model and may provide an effective tool for diagnosing DN.Keywords
Cite This Article
LIANG, W., ZHANG, Z., YANG, K., HU, H., LUO, Q. et al. (2023). Genetic algorithm-optimized backpropagation neural network establishes a diagnostic prediction model for diabetic nephropathy: Combined machine learning and experimental validation in mice. BIOCELL, 47(6), 1253–1263. https://doi.org/10.32604/biocell.2023.027373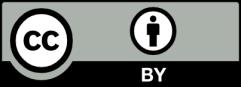