Open Access
REVIEW
Review on analytical technologies and applications in metabolomics
School of Pharmacy, Heilongjiang University of Chinese Medicine, Harbin, China
* Corresponding Author: XIN MENG. Email:
BIOCELL 2024, 48(1), 65-78. https://doi.org/10.32604/biocell.2023.045986
Received 14 September 2023; Accepted 06 November 2023; Issue published 30 January 2024
Abstract
Over the past decade, the swift advancement of metabolomics can be credited to significant progress in technologies such as mass spectrometry, nuclear magnetic resonance, and multivariate statistics. Currently, metabolomics garners widespread application across diverse fields including drug research and development, early disease detection, toxicology, food and nutrition science, biology, prescription, and chinmedomics, among others. Metabolomics serves as an effective characterization technique, offering insights into physiological process alterations in vivo. These changes may result from various exogenous factors like environmental conditions, stress, medications, as well as endogenous elements including genetic and protein-based influences. The potential scientific outcomes gleaned from these insights have catalyzed the formulation of innovative methods, poised to further broaden the scope of this domain. Today, metabolomics has evolved into a valuable and widely accepted instrument in the life sciences. However, comprehensive reviews focusing on the sample preparation and analytical methodologies employed in metabolomics within the life sciences are surprisingly scant. This review aims to fill that gap, providing an overview of current trends and recent advancements in metabolomics. Particular emphasis is placed on sample preparation, sophisticated analytical techniques, and their applications in life science research.Graphical Abstract
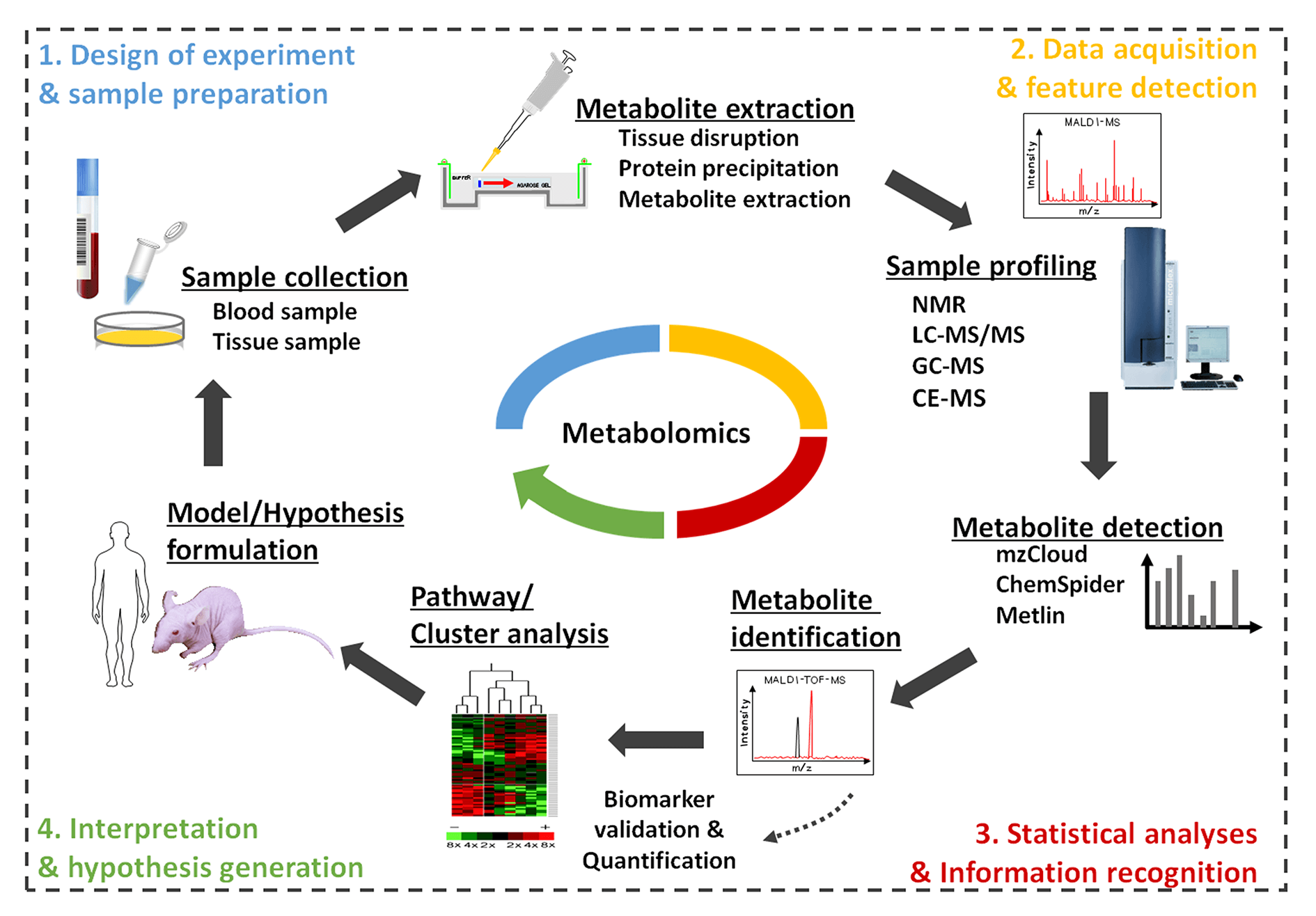
Keywords
Metabolomics, along with proteomics, transcriptomics, and genomics that constitutes the foundation of systems biology, have generated interest in metabolism in biology and medicine, especially in the areas of biomarker discovery. Metabolites, as important indicators of pathological or physiological status, could provide more information to identify biomarkers of disease, so that it could help to understand their progression and occurrence [1,2].
Metabolomics studies capture global biochemical events by analyzing a large number of small molecules in biological fluids, organs, tissues, or cells and then apply informatics techniques to define metabolomics characteristics, thereby mapping disease risk to metabolic pathways [3,4]. Typical metabolomics studies follow a similar workflow as depicted in Fig. 1, including sampling, sample preparation and extraction, analysis, listing statistically relevant candidate peaks, visualize variable data, extract differential peaks, establish a series of putative metabolites, and identify metabolites [5,6]. The widespread use of metabolomics has been achieved in drug discovery, systems biology, disease detection, drug research, nutrition and food science, toxicology and others [7–9]. In this review, the latest advancements and current trends in metabolomics were discussed in this article, with a focus on sample preparation and advanced analytical methods, applications in life science.
Figure 1: Typical metabolomics workflow.
In recent years, there has been remarkable progress in sample processing techniques within the field of metabolomics, with technologies like Microfluidic Technology at the forefront. Microfluidic Technology boasts numerous advantages in sample handling. It efficiently processes minute quantities of biological samples, ensuring precise control over fluid dynamics and enabling high-throughput analysis of multiple samples concurrently. Its automated features reduce human intervention and the likelihood of errors, simultaneously minimizing the risk of contamination, resulting in expedited and cost-effective analyses. The multifunctionality of this technology allows for the seamless integration of diverse processing steps, providing robust support for metabolomics research. When monitoring cellular responses to disturbances such as medication and disease, metabolic profiles of tissues and biofluids provide a panoramic view of changes in endogenous metabolite abundance. Metabolomics, with its myriad advantages, serves as a potent diagnostic tool, employing a range of biological fluids like urine, blood, plasma, saliva, synovial fluid, cerebrospinal fluid, semen, and tissue for analyses.
Urine, a byproduct of metabolic fluids excreted from the kidneys, flowed through the ureter to the bladder. During urination, urine is quickly excreted from the urethra. Cellular metabolism produces many metabolites that need to be removed from the bloodstream. Micturition is the main way to release water-soluble metabolites from the body.
These metabolites can be detected by urine analysis. Urine collection is a convenient biological sampling strategy, which possesses the advantages of providing time-averaged patterns of metabolites and capturing metabolites of exogenous compounds. Solid debris in urine should be removed by centrifugation at 10000~13000×g at 4°C for 5 to 10 min [10,11]. Fractions of the urine supernatants, filtered with 0.20–0.22 μm filter membrane, should be stored at −80°C [12,13]. In rodent urine, a quadruple volume of methanol is added to remove Macropeptide components and precipitatable proteins, which are freeze to −20°C for 20 min, and then centrifuged [2,14]. In nitrogen flow, the supernatant containing metabolites is evaporated to dry. Usually, the supernatant of methanol is freeze-dried for nuclear magnetic resonance (NMR) spectroscopic analysis [15,16], and then the freeze dried supernatants are dispersed in CDCl3, D2O or CD3OD [17]. While dried materials are dissolved in 0.1% formic acid or water for liquid chromatography-mass spectrometry (LC-MS) analysis [18].
Blood and other biological fluids
Blood samples are the most common sampling biological fluids, which can provide metabolic information at the time of sampling. There are large amounts of phospholipids and proteins in blood and other biological fluids [19,20]. The fluids could be extracted by the Bligh–Dyer method to separate and obtain lipid and water-soluble metabolite fractions, with butylated hydroxyl to luene to prevent oxidation. In most cases, add methanol/deuterated chloroform (v/v, 1:1) to blood samples (serum, plasma, whole blood). After vortexing for 10–15 s, supernatants are collected after series of centrifugation process. The supernatants could be dried under nitrogen or be frozen immediately to store until analysis [21]. Additionally, for more expeditious sample processing, the samples of plasma and serum could be filtered, although the result will lead to the loss of hydrophobic metabolites [22,23]. Precious blood samples, mixed with the internal chemical shift reference material and a saline solution in D2O, can be analyzed by NMR directly so that the samples could be re-used for other assays or experiments [16,24].
Tissue is a cellular framework between cells and organs, consisting of many similar cells and interstitial cells. In vivo, the concentration of metabolites in body fluid reflects the overall effect in vivo. However, in the same tissue, different positions and different types of cells are metabolized simultaneously. In animal and human models, biopsy or tissue samples are more and more become the hot spot of research. When collecting tissue samples, it should be quickly frozen in −196°C liquid nitrogen until extracted to prevent further metabolism [25,26].
Normally, samples are cleaned with cold saline to reduce the interference of blood metabolites in the detection of tissue metabolites. In extracting tissue metabolites, frozen tissue needs to be ground into powder in the pestle and freezed with liquid nitrogen. It can also be used for liquid nitrogen impact crushing and the use of French Press (for plant tissue). The frozen powder tissue was then extracted by cold methanol (4 v/g) or 0.1% perchloric acid to precipitate and inactivate the enzyme [27], and the precipitated protein was removed by centrifugation. Add chloroform and water to remove lipids [28,29]. Perchloric acid was removed from the extract by potassium hydroxide neutralization. Because the solubility of potassium perchlorate in water should be very low, it can be removed by centrifugation [30].
For adherent cells growing on culture plates or suspension cells, Pasteur pipettes connected to vacuum lines are an effective way to remove the medium quickly [31,32]. After removing the culture medium, the cells should be washed with isotonic saline and rotated for a few seconds. The cells were then extracted from methanol freeze to dry ice temperature (−43°C). If no immediate extraction is needed, it should be kept in liquid nitrogen [33]. The preparation of cell samples must prevent further metabolism. During the analysis, cells were removed from the culture plate with a cell scraper [34,35]. The mixture was centrifuged (4000×g) for 30 min and the supernatant was sucked out. Methanol is evaporated in nitrogen flow. The specific operation methods need to be partly adjusted according to the experimental cells and experimental types.
In the future, sample processing in metabolomics is set to undergo significant transformations. These will focus on refining precision and bolstering efficiency. A key development will be the enhanced automation in sample preparation processes, ensuring consistent outcomes, minimizing human errors, and thus bolstering the reproducibility of findings across various studies. It’s anticipated that the field will align more closely with other ‘omics’ technologies, necessitating innovative preparation techniques to safeguard the integrity of diverse molecules under study. Furthermore, cutting-edge preservation methodologies, encompassing emerging cryopreservation techniques and novel chemical stabilizers, might drastically extend sample shelf life without compromising their quality.
Targeted and untargeted analysis
Targeted metabolomics enables researchers to investigate specific compounds or compound groups within metabolites [36–38]. In scenarios with limited known data, untargeted analyses are more appropriate. These analyses are intricate due to the vast number of metabolites present, ranging from hundreds in prokaryotes to thousands in humans [39–41]. Nonetheless, untargeted analysis is instrumental in preliminary research, granting a wealth of information about the sample.
At present, metabolites can be analyzed by gas chromatography-mass spectrometry (GC-MS) or LC-MS to study gene-induced metabolic disorders [42,43]. In clinical practice, the targeting analysis of certain known compounds is usually used to determine the occurrence and development of the disease. Modern mass spectrometry can track hundreds of compounds or even more in one analysis [44,45]. When metabolic changes in the disease are not clear, researchers should adopt a more open analytical method, non-targeted metabolomics studies, for non-discriminatory analysis. This method can provide comprehensive information about metabolic changes [46].
Instrumentation in Metabolomics Research
Currently, NMR spectroscopy and mass spectrometry, either used singly or in combination, dominate the field of metabolomics research. Both NMR and MS offer distinct advantages and challenges in metabolite analysis, as summarized in Table 1. Although NMR is more stable and repeatable, can provide more structural information, its instrument cost is high, the sensitivity is low. When using NMR analysis, many low metabolites are not easy to detect, and then affect the analysis results [47,48]. Although mass spectrometry has higher sensitivity, the results of mass spectrometry are related to the composition and proportion of mobile phase and the structure of the analyte. When using an electrospray ion source, the ionization efficiency of different molecules is different, resulting in different analytical results. At present, MS is still widely used in metabolomics research [20,49,50].
Nuclear magnetic resonance spectroscopy
NMR analysis can detect molecules with nonzero magnetic moments, such as 1H, 13C, 14N, and 31P. Metabolites usually contain hydrogen atoms, and the abundance of 1H atoms (99.98%) is very high, nuclear magnetic signal is relatively strong, so metabolomics often test and analyze the hydrogen spectrum. In NMR, each metabolite has its own unique spectrum, and each absorption peak represents the chemical and magnetic environment of each proton [51,52]. In the metabolomics samples, mixed samples of different metabolites produce complex NMR spectra.
Currently, the 800 MHz NMR spectrometer is more advanced in metabolomics research. The greater the magnetic field intensity, the higher the resolution. In order to obtain better resolution, improve the magnetic field intensity as much as possible. Compared with earlier instruments, today’s NMR spectrometers can automatically load and unload metabolite extracts [52,53]. Many instruments also use cryo probe and micro probe to increase the sensitivity of the instrument. The analysis of metabolites is based on the pulse sequence and the nuclear magnetic resonance phenomenon of hydrogen atoms [53–55].
NMR, as a non-destructive analytical technique, can be used to analyze the samples after NMR spectrum analysis, and then to analyze GC- or LC-MS. The fine structure of metabolites can be observed by NMR analysis, which provides valuable information for the discovery of new compounds [56,57]. In addition, magic angle NMR can directly observe metabolite signals in tissues without preliminary extraction, thus reducing the errors caused by the pretreatment process. It is also suitable for in vivo analysis [58,59].
In the analysis, the amount of sample required for nuclear magnetic resonance analysis is higher than that of mass spectrometry. NMR analysis of humoral metabolites requires at least 0.5 milliliters of urine, serum and plasma. If it is solid, it needs 0.5 g tissue or feces [60,61]. If analyze the cells cultured in vitro, it need at least 10 million cells [62]. If a small sample is used, a large number of low abundance metabolites will be lost. A small diameter NMR tube can be used when using less NMR samples. The use of heparin is superior to EDTA or citrate in the analysis of plasma because heparin interferes less with the hydrogen spectrum [63]. In metabolomics, many databases can be used to identify metabolites in samples when using NMR for targeted and non-targeted analysis [64]. Now NMR spectroscopy has developed a new pulse procedure for analyzing lipids [51,65].
The magic angle spinning (MAS) technique has gained traction for intact cell or tissue analysis. By rapidly rotating the sample at an angle of 54.7 degrees (5 kHz) within the instrument, MAS mitigates resonance broadening and information loss stemming from short relaxation times and sample heterogeneity [66–68]. This method has been used to analyze the whole cell of the microalgae Thalassiosirapseudonana, the disease biomarkers of red abalone and to determine the mode of action of biochemical substances, to analyze the metabolite concentration changes of Saccharomyces cerevisiae mutants [67,68], and to determine the functional association between unknown functional genes and known genes [68].
Mass spectrometry is based on the separation of metabolites through certain methods, such as liquid phase, gas phase, capillary electrophoresis as depicted in Fig. 2, the sample of metabolites are separated, and then into the mass spectrometer, ionized by ion source, analyzed, resulting in a spectrum.
Figure 2: Various configurations of mass spectrometers.
Gas chromatography-mass spectrometry: Gas chromatography is usually employed to analyze volatile components. Metabolomics originally used gas chromatography to analyze volatile components in plants [34,69,70]. As a result of the development of gas chromatography and mass spectrometry technology, it is commonly used gas chromatography and mass spectrometry analysis of common biological samples, such as urine, serum, plasma, bacteria, cells and so on [71–73]. If the sample is a volatile compound, it can be injected by a headspace sampler. Headspace sampling only measures gas molecules captured in the top air. In most cases, metabolites in biological samples need to be extracted first, then derived, and then entered the gas chromatograph [74,75]. Most metabolites contain polar functional groups and are not volatile or heat-labile at separation temperature. Therefore, derivatization is necessary before gas chromatography.
In recent years, many papers have reported the use of derivatization to analyze metabolites by gas chromatography-mass spectrometry, such as the use of oxime reagent derivatization, then methylsilylation, analysis of carbohydrates, organic acids, amino acids and so on [76–78]. Metabolites containing hydroxyl, amine, amide, phosphate and mercaptan groups can be derived by alkylmethylsilane method. Recently, many metabolomics studies of plant metabolites or urine samples have adopted GC-MS analysis techniques of methylsilylation derivatization or oximation and methylsilylation derivatization [34,76,79]. GC-MS, as a high-throughput metabolomics analysis method, can be used to quantify a large number of metabolites in a single assay [72,80,81]. This method has obvious advantages in the determination of small metabolites (<400 Da), but it is not reliable in the determination of thermolabile compounds [82–84].
As a mature analytical technology, GC-MS has been widely applied in metabolomics. GC-MS can be applied to urine screening, disease research, microbial research, clinical research, etc. [85–87]. The metabolites and volatile substances in plant cells were analyzed by GC-MS to study the effects of gene and environmental changes on metabolism [50,88]. Multidimensional analysis (e.g., GC×GC-TOF-MS) will enhance the specificity and sensitivity of the analysis, and will make GC-MS more widely used in metabolomics research [80,89].
Liquid chromatography-mass spectrometry: Liquid chromatography mass spectrometry is used to separate metabolites in samples by high performance liquid chromatography. Then, mass spectrometry was analyzed by electrospray ionization or ionization of atmospheric chemical ion sources. The transfer of charged ions from the liquid phase to the gas phase in the LC-MS analysis requires no derivatization. ESI ion sources were used to analyze peptides and proteins, carboxylates, nitrogen compounds and amines [38,90,91]. For neutral molecular metabolites, atmospheric pressure chemical ionization can be used to enhance the formation of [M+H]+ or [M-H]+ or complex molecular ions [92–94]. In metabolomics analysis, LC-MS can be used to quantitative analyze metabolites suspected to be known compounds. This method is usually performed on triple quadrupole or Qtrap mass spectrometer and is widely used to analyze known biochemical molecules and drugs.
In recent years, LC-MS has been widely applied in metabolomics for clinical research [95,96]. In this way, biomarkers and drugs of many diseases in animals and humans are found to interfere with diseases. Using screening technology, some characteristic metabolites will be identified, followed by target analysis. At present, LC-NMR-MS technology is being explored in metabolomics to help identify metabolites [97,98].
Capillary electrophoresis-mass spectrometry: Capillary electrophoresis (CE) has been used in metabolomics analysis for a long time, and it is very effective in separating hydrophilic compounds [99,100]. Compared with liquid chromatography, capillary electrophoresis can be analyzed with a lower sample size (1–20 nl) [101,102]. Because of the technical problems of CE-ESI interface, the application of CE-MS in metabolomics has been greatly restricted.
In the analysis of metabolites in serum and cerebrospinal fluid samples, these fluids should be ultrafiltered to avoid protein precipitation in capillaries [103,104]. In addition, water can be used to dilute plasma, cerebrospinal fluid and urine at a ratio of 1:1 to improve the analytical results. In urine analysis, 9 nl samples can analyze more than 3500 characteristics. Up to now, the sheathless fluid interface of CE-MS has been widely used in the study of cationic metabolites, such as drug beta-glucosidate non-aqueous CE-MS method. CE-MS with no sheath fluid interface has broad application prospects in metabonomics research.
CE-MS, as an effective method for analyzing charged metabolites, can be used to detect metabolites containing hydroxyl, amino, phosphate and carboxyl groups [105,106]. The advantage of CE-MS is that it requires less samples. In metabolomics, CE-MS was used to analyze the metabolites of Bacillus subtilis, and 1692 peaks were obtained. At present, CE-MS analysis of metabolites is widely used in environmental, biomedical, clinical medicine, plant metabolism and so on [100,107].
Atmospheric pressure scanning microprobe matrix-assisted laser desorption ionization mass spectrometry imaging: Spatial Metabolomics is a method based on mass spectrometry imaging technology for the analysis of samples in metabolomics. It allows for the extension of information about the types and quantities of metabolites in a sample from the realm of metabolomics to the spatial dimension, enabling the analysis of differential changes in biological metabolism processes in terms of qualitative, quantitative, and spatial aspects [108]. Atmospheric pressure scanning microprobe matrix-assisted laser desorption ionization mass spectrometry imaging (AP-SMALDI-MSI) is employed for direct scanning and imaging of biological samples in operating environments ranging from atmospheric to intermediate pressure. This technique uses matrix-assisted laser desorption ionization (MALDI) to measure the mass-to-charge ratio (m/z) of ions with a mass spectrometer and utilizes specialized mass spectrometry imaging software to generate molecular images. This technology not only enables the untargeted detection of the composition and relative abundance of metabolites in samples but also provides information on the specific spatial distribution of metabolites within the sample. As a result, it holds significant promise for applications in various fields, including clinical medicine, molecular biology, botany, and zoology [109].
With the rapid advancement of metabolomics, specialized analytical instrument technologies for detecting small molecules within biological systems have also made significant progress. Presently, these instruments offer higher sensitivity, resolution, and accuracy. In the future, we anticipate further miniaturization of these instruments and their integration with artificial intelligence and machine learning technologies to enhance the efficiency of data processing and interpretation. Simultaneously, the development of multi-omics analysis platforms will provide support for a more comprehensive understanding of biological systems. In summary, the prospects for metabolomics analytical instruments are promising, and technological advancements will continue to drive our understanding of the metabolic pathways behind health and disease.
Metabolomics, with its powerful phenotyping ability, has emerged as an invaluable tool in understanding life systems and influencing health determinants [110–112]. With the continuous development of metabolomics research technology, many clinical researchers and drug researchers have provided new ideas and methods [113–115]. Currently, metabolomics is commonly used in disease research, drug development, Chinmedomics, nutrition and gut microflora research [110,116,117]. This evolving technology is mainly applied as follows.
Chinese medicine is an ancient practice of the Chinese people to prevent and cure diseases from ancient times to the present [118,119]. With the development of modern science and medicine, as an effective treatment method, millions of people in the world still use traditional Chinese medicine. Chinmedomics, which integrates serum pharmacochemistry with metabolomics, uses modern science and technology to clarify the scientific value of traditional Chinese medicine, and uses a unique method combined with metabolomics and chemometrics to clarify the major changes of metabolites in the body under the condition of comprehensive symptoms and to analyze the effective components of Chinese medicine in treating diseases [120–123]. Given the multi-target, multi-component treatment approach of traditional Chinese medicine and the holistic focus of metabolomics, Chinmedomics represents a harmonious integration of both domains. By discovering biomarkers unique to specific Traditional Chinese Medicine syndromes, it offers a modern scientific interpretation of ancient TCM theories [121,124–126].
In functional genomics, metabolomics can effectively characterize the metabolite changes caused by gene expression [42,127,128]. Through changes in metabolites, functional gene changes can be speculated, such as insertion or deletion [45,129,130]. At the same time, metabolomics can be used to determine the success of gene editing by comparing the metabolites before and after gene insertion or deletion. In recent years, researchers have also studied the function of location genes through metabolomics [130–132]. This technology has gradually formed metabologenomics, which is a new method combining biometabolites with genomics. Metabologenomics uses bioinformatics matching methods to identify biosynthetic small molecules by non-targeted metabolomics analysis of metabolites for discovering new natural products and determining gene functions [133–135].
The application of metabolomics in pharmacology gives rise to a new field called “pharmacometabolomics” [136–138]. The basic tenet of pharmacometabolomics is to determine the metabolism of the drug itself and its influence on the metabolic process of the organism during treatment. It is also used to identify specific metabolic pathways that are responsible for drug-mediated outcomes. Pharmacometabolomics is applied to a wide range of research areas, including drug discovery, pharmacology, clinical pharmacology, personalized drug therapy and clinical trials [138–140]. Such information subsequently facilitates the discovery of biomarkers that are associated with pathologic conditions and play vital roles in mapping the effectiveness of drug therapies through various metabolomics approaches [141]. The identification of metabolic pathways affected by a specific drug treatment through metabolomics, as well as a subsequent analysis of genetic variations within these metabolic pathway components could help to achieve personalized medicine [138,142,143]. Profiling of drug-induced changes in metabolites or creating metabolite signatures of different disease subtypes, such as invasive or non-invasive tumors, through high-resolution targeted metabolomics tools serves an important biomarker discovery and diagnostic tool development [143–145].
Nutrimetabonomics is a combination of diet and metabolomics [146–148]. It is mainly used to study the molecular relationship between nutrients and biochemical processes, to analyze the effects of food nutrients on metabolism, and to study the cross-genomic metabolite interactions between mammalian hosts and gut microflora as depicted in Fig. 3 [149–151].
Figure 3: Various configurations of mass spectrometers.
Metabolites in body fluids are usually influenced by endogenous and exogenous factors, endogenous factors such as gender, age, underlying diseases, etc. Small bowel tumors are also one of the potential sources of influence on metabolites [152]. Exogenous factors are mainly diet and medicine, and the diet is divided into nutrients and non-nutrients. Metabolomics is an effective method to determine biological characteristics and metabolic fingerprints, which can detect the final impact of these factors on life [153,154].
In the last decade, metabolomics has been used as an effective research method to study the interaction between gut microbiota and mammalian metabolism [155,156]. Gut microbiota constitute a microecosystem in organisms. Their metabolites interact with the metabolites of organisms and participate in physiological activities, such as immune regulation, fat metabolism, energy production, digestion, nutrient absorption and so on [157–159]. Multiple interactions between intestinal flora and host multiple metabolites can be characterized by metabolomics [160–162].
Metabolomics plays an essential role in toxicological research by providing a detailed analysis of altered metabolic pathways that are targeted by harmful chemicals [163–165].
Toxicity assessment, through metabolomics analysis of urine or blood samples collected to detect the physiological changes and toxic damage caused by the substance [164–166]. The main advantage of using metabolomics in toxicology is that alterations in metabolism are terminal events to genetic, transcriptomic, and proteomic levels. Thus, metabolomics facilitates the understanding of direct cellular phenotypes that are induced by toxic insults. In addition, since changes in extracellular metabolites mirror the intracellular metabolic scenario, metabolomics analysis of biological fluids obtained through non-invasive or minimally invasive techniques suffices for the evaluation of target organ toxicities [166–168]. Another advantage of metabolomics is that information on a large number of metabolites could be obtained through a single measurement, an oftentimes-basic requirement for rapidly understanding the level of drug toxicity. A large-scale screening of drugs or chemical compounds can be achieved through in vitro metabolomics approaches [169–171].
One important use of in vitro metabolomics, which employs cell lines, primary cells, co-cultures in 2D or 3D format, etc., is to predict toxicological patterns of unknown chemicals in the biological system using known reference chemicals [170,172]. Such analysis starts with the comparison of a metabolic profile of a known reference chemical with its toxicological profile in vivo. This allows for the generation of a ‘toxicity pattern,’ which defines the metabolic profile related to a distinct toxicological endpoint [168,173,174]. Afterwards, the degree of overlap is calculated by aligning the metabolic profile of the unknown chemical with the toxicity pattern. The data are used to predict the toxicological pattern of an unknown chemical.
Metabolomics plays a crucial role in disease diagnosis in recent years. By analyzing the types and quantities of metabolites within an individual, metabolomics provides a powerful tool for early disease detection, disease classification, monitoring treatment responses, and biomarker discovery [175,176]. Early disease diagnosis is a prominent application area. Metabolomics can detect changes in metabolites within the body, aiding in the identification of early signs of various conditions such as diabetes, cancer, and cardiovascular diseases [177]. Early diagnosis often offers better treatment opportunities and enhances the likelihood of successful outcomes. Furthermore, metabolomics contributes to disease classification and subtype differentiation. By studying metabolic differences among different subgroups, a better understanding of disease diversity and individual variations is achieved, supporting the field of precision medicine. During treatment, metabolomics is also utilized to monitor changes in disease progression and treatment effectiveness [177,178]. This helps physicians adjust treatment plans to ensure optimal patient outcomes. Metabolomics plays a pivotal role in biomarker discovery as well. By comparing metabolic differences between healthy individuals and those with specific diseases, metabolomics aids in identifying novel biomarkers. These biomarkers can be used for disease diagnosis, prognosis, and monitoring, providing essential information for medical decision-making [179,180].
In conclusion, the applications of metabolomics in disease diagnosis represent significant breakthroughs in the medical field. Metabolomics has the potential to improve disease management, enhance the quality of life for patients, and offer new opportunities for medical research.
An important task of metabolomics is to screen reliable biomarker metabolites to detect changes in organisms. Therefore, in metabolomics analysis, a more sensitive, fast and reliable analytical tool is needed. The treatment of samples changed with the upgrading of the instrument. With the different methods of metabolomics, the sample processing methods are different. With the development of science and technology, metabolomics as an effective analytical method will be applied to more research fields. At present, NMR and MS-based metabolomics is gradually expanding its application with the development of science and technology. In the future, in vivo analysis and on-line analysis are the research directions of metabolomics.
Despite numerous advantages of metabolomics applied in many fields of life sciences and clinical medicines, some difficulties and concerns partly hold back its full-fledged application. Firstly, in order to apply metabolomics in biomarker and drug discovery, an accurate and highly reproducible quantification of metabolites is a prime requirement. Such need is often difficult to achieve due to complexities of analytical tools. Secondly, in order to establish an optimal drug-response, the right samples and an adequate sample size should always be kept in check. Thirdly, since metabolomics data often involves a large number of variables with minimal sample size, selecting appropriate statistical tools must be ensured in order to avoid data misinterpretation. Further studies should widen the scope of this interesting method.
Acknowledgement: None.
Funding Statement: This work was supported by the Science Foundation of Heilongjiang Administration of Traditional Chinese Medicine (No. 2018-21).
Author Contributions: Study conception and design: Meng, Liu; Data collection: Xu; Analysis and interpretation of results: Liu, Yin, Yang; Draft manuscript preparation: Meng, Liu. All authors reviewed the results and approved the final version of the manuscript.
Availability of Data and Materials: Data sharing not applicable to this article as no datasets were generated or analyzed during the current study.
Ethics Approval: Not applicable.
Conflicts of Interest: The authors declare that they have no conflicts of interest to report regarding the present study.
References
1. Zhu K, Zhang J, Zhang CC, Zhao SF, Gao J, Guan JZ. Metabolomics-based analysis of plasma in postmenopausal women with normal bone mineral density, postmenopausal osteoporosis, and rheumatoid arthritis osteoporosis. All Life. 2023;16(1):2185174. doi:https://doi.org/10.1080/26895293.2023.2185174 [Google Scholar] [CrossRef]
2. Wang SS, Fang J, Li J, Wang SH, Su PY, Wan YH, et al. Identification of urine biomarkers associated with early puberty in children: an untargeted metabolomics analysis. Physiol Behav. 2023;270(15):114305. doi:https://doi.org/10.1016/j.physbeh.2023.114305 [Google Scholar] [PubMed] [CrossRef]
3. Rushing BR, Molina S, Sumner S. Metabolomics analysis reveals altered metabolic pathways and response to doxorubicin in drug-resistant triple-negative breast cancer cells. Metabolites. 2023;13(7):865. doi:https://doi.org/10.3390/metabo13070865 [Google Scholar] [PubMed] [CrossRef]
4. Samad A, Shaukat K, Ansari MUR, Nizar M, Zahra N, Naz A, et al. Role of foliar spray of plant growth regulators in improving photosynthetic pigments and metabolites in Plantago ovata (Psyllium) under salt stress-A field appraisal. BIOCELL. 2023;47(3):523–32. doi:https://doi.org/10.32604/biocell.2023.023704 [Google Scholar] [CrossRef]
5. Huang FF, Yu JJ, Lai TW, Luo LX, Zhang WZ. The combination of bioinformatics analysis and untargeted metabolomics reveals potential biomarkers and key metabolic pathways in asthma. Metabolites. 2023;13(1):25. doi:https://doi.org/10.3390/metabo13010025 [Google Scholar] [PubMed] [CrossRef]
6. Angioni S, Saponara S, Vitale SG. Metabolomics analysis in endometriosis patients: is it a step toward the future? Gynecol Endocrinol. 2023;39(1):2227276. doi:https://doi.org/10.1080/09513590.2023.2227276 [Google Scholar] [PubMed] [CrossRef]
7. Meng X, Li X, Yang LR, Yin R, Qi LH, Guo Q. Review on microbial metabolomics of probiotics and pathogens: methodologies and applications. Biocell. 2023;47(1):91–107. doi:https://doi.org/10.32604/biocell.2023.024310 [Google Scholar] [CrossRef]
8. Spinelli LV, Anzanello MJ, Santos RAD, Martins CC, Saggin JF, da Silva MAS, et al. Uncovering the phenolic diversity of Guabiju fruit: LC-MS/MS-based targeted metabolomics approach. Food Res Int. 2023;173(1):113236. doi:https://doi.org/10.1016/j.foodres.2023.113236 [Google Scholar] [PubMed] [CrossRef]
9. Wei YM, Yin XC, Zhao MJ, Zhang JX, Li TH, Zhang YY, et al. Metabolomics analysis reveals the mechanism underlying the improvement in the color and taste of yellow tea after optimized yellowing. Food Chem. 2023;428:136785. doi:https://doi.org/10.1016/j.foodchem.2023.136785 [Google Scholar] [PubMed] [CrossRef]
10. Glass KA, Germain A, Huang YV, Hanson MR. Urine metabolomics exposes anomalous recovery after maximal exertion in female ME/CFS patients. Int J Mol Sci. 2023;24(4):3685. doi:https://doi.org/10.3390/ijms24043685 [Google Scholar] [PubMed] [CrossRef]
11. Rhodes AML, Ali S, Minnion M, Lee LH, Joseph BM, Ndzo J, et al. An explorative study into the aetiology of developmental dysplasia of the hip using targeted urine metabolomics. Antioxidants. 2023;12(3):538. doi:https://doi.org/10.3390/antiox12030538 [Google Scholar] [PubMed] [CrossRef]
12. Batty CA, Cauchi M, Hunter JO, Woolner J, Baglin T, Turner C. Differences in microbial metabolites in urine headspace of subjects with immune thrombocytopenia (ITP) detected by volatile organic compound (VOC) analysis and metabolomics. Clin Chim Acta Int J Clin Chem. 2016;461:61–8. doi:https://doi.org/10.1016/j.cca.2016.07.021 [Google Scholar] [PubMed] [CrossRef]
13. Wang R, Qi SY, Zhang JD, Ren HT, Xie LG, Liu CY. Nontargeted urine metabolomics with electrospray ionization-mass spectrometry reveals the major metabolic characterization between adult males and females involved in calcium oxalate urolithiasis. Anal Lett. 2023. doi:https://doi.org/10.1080/00032719.2023.2248530 [Google Scholar] [CrossRef]
14. Yang RY, Wang Y, Yuan CH, Shen XZ, Cai M, Wang LY, et al. The combined analysis of urine and blood metabolomics profiles provides an accurate prediction of the training and competitive status of Chinese professional swimmers. Front Physiol. 2023;14:1197224. doi:https://doi.org/10.3389/fphys.2023.1197224 [Google Scholar] [PubMed] [CrossRef]
15. Bingol K, Bruschweiler R. NMR/MS translator for the enhanced simultaneous analysis of metabolomics mixtures by NMR spectroscopy and mass spectrometry: application to human urine. J Proteome Res. 2015;14(6):2642–8. doi:https://doi.org/10.1021/acs.jproteome.5b00184 [Google Scholar] [PubMed] [CrossRef]
16. Ouyang T, Ma CC, Zhao Y, Ye W, Zhao JY, Cai RZ, et al. H-1 NMR-based metabolomics of paired tissue, serum and urine samples reveals an optimized panel of biofluids metabolic biomarkers for esophageal cancer. Front Oncol. 2023;13:1082841. doi:https://doi.org/10.3389/fonc.2023.1082841 [Google Scholar] [PubMed] [CrossRef]
17. Chen L, Xiang H, Xing J, Tian JS, Qin XM, Du GH. 1H NMR based metabolomics study of bu-zhong-yi-qi-tang in the spleen-qi deficiency rat model. Yao Xue Xue Bao = Acta Pharm Sin. 2014;49(9):1320–5. Available from: http://www.ncbi.nlm.nih.gov/pubmed/25518333. [Accessed 2014]. [Google Scholar]
18. Struck-Lewicka W, Karpinska B, Rodzaj W, Nasal A, Wielgomas B, Markuszewski MJ, et al. Development of the thin film solid phase microextraction (TF-SPME) method for metabolomics profiling of steroidal hormones from urine samples using LC-QTOF/MS. Front Mol Biosci. 2023;10:1074263. doi:https://doi.org/10.3389/fmolb.2023.1074263 [Google Scholar] [PubMed] [CrossRef]
19. Qian FM, Zhao L, Zhang D, Yu MJ, Zhou W, Jin J. Serum metabolomics detected by LDI-TOF-MS can be used to distinguish between diabetic patients with and without diabetic kidney disease. FEBS Open Bio. 2023;13(10):1807–1986. doi:https://doi.org/10.1002/2211-5463.13683 [Google Scholar] [PubMed] [CrossRef]
20. Wang YD, Luo YF, Yang S, Jiang MY, Chu Y. LC-MS/MS-based serum metabolomics and transcriptome analyses for the mechanism of augmented renal clearance. Int J Mol Sci. 2023;24(13):10459. doi:https://doi.org/10.3390/ijms241310459 [Google Scholar] [PubMed] [CrossRef]
21. Zhang H, Ma XZ, Xu JF, Jin P, Yang L, Pan YM, et al. Serum metabolomics of hyperbilirubinemia and hyperuricemia in the Tibetan plateau has unique characteristics. Sci Rep. 2023;13(1):12772. doi:https://doi.org/10.1038/s41598-023-40027-6 [Google Scholar] [PubMed] [CrossRef]
22. Budczies J, Denkert C. Tissue-Based metabolomics to analyze the breast cancer metabolome. Recent Results in Cancer Res Fortschritte Krebsforschung Progres dans les Recherches sur le Cancer. 2016;207(1):157–75. doi:https://doi.org/10.1007/978-3-319-42118-6_7 [Google Scholar] [PubMed] [CrossRef]
23. Zhou JN, Pi N, Guo YQ, He XY, Wang JH, Luo R, et al. The mechanism of action of Ophiocordyceps sinensis mycelia for prevention of acute lung injury based on non-targeted serum metabolomics. PLoS One. 2023;18(6):287331. doi:https://doi.org/10.1371/journal.pone.0287331 [Google Scholar] [PubMed] [CrossRef]
24. Pedersen S, Mikkelstrup MF, Kristensen SR, Anwardeen NR, Elrayess MA, Andreassen T. Serum NMR-based metabolomics profiling identifies lipoprotein subfraction variables and amino acid reshuffling in myeloma development and progression. Int J Mol Sci. 2023;24(15):12275. doi:https://doi.org/10.3390/ijms241512275 [Google Scholar] [PubMed] [CrossRef]
25. Haddadi BS, Fang R, Girija A, Kattupalli D, Widdowson E, Beckmann M, et al. Metabolomics targets tissue-specific responses in alleviating the negative effects of salinity in tef (Eragrostis tef) during germination. Planta. 2023;258(3):67. doi:https://doi.org/10.1007/s00425-023-04224-x [Google Scholar] [PubMed] [CrossRef]
26. Li M, Geng WR, Wang Z, Wang Q, Pang L, Wang BY, et al. Analysis of the utilization value of different tissues of TaxusxMedia based on metabolomics and antioxidant activity. Bmc Plant Biol. 2023;23(1):285. doi:https://doi.org/10.1186/s12870-023-04308-6 [Google Scholar] [PubMed] [CrossRef]
27. Wang ZZ, Li FH, Ni PS, Sun L, Zhang CK, Li BM, et al. Age-related changes in adipose tissue metabolomics and inflammation, cardiolipin metabolism, and ferroptosis markers in female aged rat model. Biochem Bioph Res Commun. 2023;671:292–300. doi:https://doi.org/10.1016/j.bbrc.2023.06.027 [Google Scholar] [PubMed] [CrossRef]
28. Battini S, Faitot F, Imperiale A, Cicek AE, Heimburger C, Averous G, et al. Metabolomics approaches in pancreatic adenocarcinoma: tumor metabolism profiling predicts clinical outcome of patients. BMC Med. 2017;15(1):56. doi:https://doi.org/10.1186/s12916-017-0810-z [Google Scholar] [PubMed] [CrossRef]
29. Zhang ZH, Zhang JP, Zhao S, Peng TT, Zhang M, Xu XZ, et al. Synovial tissue metabolomics analysis of the therapeutic effects of stir-fried Xanthii Fructus on rheumatoid arthritis in rats. J Chromatogr B. 2023;1216:123594. doi:https://doi.org/10.1016/j.jchromb.2023.123594 [Google Scholar] [PubMed] [CrossRef]
30. Dailey A, Solano-Aguilar G, Urban JF, Couch RD. LC-QToF-based metabolomics identifies aberrant tissue metabolites associated with a higher-fat diet and their ‘reversion to healthy’ with dietary probiotic supplementation. Metabolites. 2023;13(3):358. doi:https://doi.org/10.3390/metabo13030358 [Google Scholar] [PubMed] [CrossRef]
31. Arnold K, Dehio P, Lotscher J, Singh KD, Garcia-Gomez D, Hess C, et al. Real-time volatile metabolomics analysis of dendritic cells. Anal Chem. 2023;95(25):9415–21. doi:https://doi.org/10.1021/acs.analchem.3c00516 [Google Scholar] [PubMed] [CrossRef]
32. Chattopadhyay R, Mitra I, Sharma S, Bose G, Ghosh S, Kalapahar S, et al. Predicting live birth rate using combined Day3-spent embryo culture medium metabolomics and cumulus cell gene expression based algorithm to optimize embryo selection in unexplained infertility. Hum Reprod. 2023;38(1):116. doi:https://doi.org/10.1093/humrep/dead093.116 [Google Scholar] [CrossRef]
33. Ibanez C, Simo C, Valdes A, Campone L, Piccinelli AL, Garcia-Canas V, et al. Metabolomics of adherent mammalian cells by capillary electrophoresis-mass spectrometry: HT-29 cells as case study. J Pharm Biomed Anal. 2015;110:83–92. doi:https://doi.org/10.1016/j.jpba.2015.03.001 [Google Scholar] [PubMed] [CrossRef]
34. Wang T, Yin SS, Gu J, Li JJ, Zhang MM, Shan JJ, et al. Study on the Intervention mechanism of cryptotanshinone on human A2780 ovarian cancer cell line using GC-MS-based cellular metabolomics. Pharmaceuticals. 2023;16(6):861. doi:https://doi.org/10.3390/ph16060861 [Google Scholar] [PubMed] [CrossRef]
35. Zha AD, Liao SM, Tan B, Liao P. Integrated lncRNA transcriptomics, proteomics, and metabolomics to identify early cellular response variation in deoxynivalenol-treated IPEC-J2 cells. Food Chem Toxicol. 2023;177:113863. doi:https://doi.org/10.1016/j.fct.2023.113863 [Google Scholar] [PubMed] [CrossRef]
36. Artymowicz M, Struck-Lewicka W, Wiczling P, Markuszewski M, Markuszewski MJ, Siluk D. Targeted quantitative metabolomics with a linear mixed-effect model for analysis of urinary nucleosides and deoxynucleosides from bladder cancer patients before and after tumor resection. Anal Bioanal Chem. 2023;415(22):5511–28. doi:https://doi.org/10.1007/s00216-023-04826-0 [Google Scholar] [PubMed] [CrossRef]
37. Chang YJ, Yoo HJ, Kim SJ, Lee KH, Lim CM, Hong SB, et al. A targeted metabolomics approach for sepsis-induced ARDS and its subphenotypes. Crit Care. 2023;27(1):263. doi:https://doi.org/10.1186/s13054-023-04552-0 [Google Scholar] [PubMed] [CrossRef]
38. Nagy K, Darko E, Szalai G, Janda T, Jokai Z, Ladanyi M, et al. UPLC-ESI-QTOF-MS assisted targeted metabolomics to study the enrichment of vinca alkaloids and related metabolites in Catharanthus roseus plants grown under controlled LED environment. J Pharmaceut Biomed. 2023;235:115611. doi:https://doi.org/10.1016/j.jpba.2023.115611 [Google Scholar] [PubMed] [CrossRef]
39. Bowen TJ, Southam AD, Hall AR, Weber RJM, Lloyd GR, Macdonald R, et al. Simultaneously discovering the fate and biochemical effects of pharmaceuticals through untargeted metabolomics. Nat Commun. 2023;14(1):4653. doi:https://doi.org/10.1038/s41467-023-40333-7 [Google Scholar] [PubMed] [CrossRef]
40. Guo TT, Deng YR, Huang X, Yan CW, Gao X, Wu Y, et al. Untargeted metabolomics reveal the metabolic profile of normal pulmonary circulation. Resp Med. 2023;217:107369. doi:https://doi.org/10.1016/j.rmed.2023.107369 [Google Scholar] [PubMed] [CrossRef]
41. Hsu FC, Palmer ND, Chen SH, Ng MCY, Goodarzi MO, Rotter JI, et al. Methods for estimating insulin resistance from untargeted metabolomics data. Metabolomics. 2023;19(8):72. doi:https://doi.org/10.1007/s11306-023-02035-5 [Google Scholar] [PubMed] [CrossRef]
42. Poorna R, Chen WW, Qiu P, Cicerone MT. Toward gene-correlated spatially resolved metabolomics with fingerprint coherent raman imaging. J Phys Chem B. 2023;127(25):5576–87. doi:https://doi.org/10.1021/acs.jpcb.3c01446 [Google Scholar] [PubMed] [CrossRef]
43. Cheng Q, Zeng LQ, Wen H, Brown SE, Wu H, Li XY, et al. Steroidal saponin profiles and their key genes for synthesis and regulation in Asparagus officinalis L. by joint analysis of metabolomics and transcriptomics. Bmc Plant Biol. 2023;23(1):207. doi:https://doi.org/10.1186/s12870-023-04222-x [Google Scholar] [PubMed] [CrossRef]
44. Peng YC, Yin DH, Li XX, Wang K, Li W, Huang YX, et al. Integration of transcriptomics and metabolomics reveals a novel gene signature guided by FN1 associated with immune response in oral squamous cell carcinoma tumorigenesis. J Cancer Res Clin. 2023;149(9):6097–113. doi:https://doi.org/10.1007/s00432-023-04572-x [Google Scholar] [PubMed] [CrossRef]
45. Ramirez-Acosta S, Huertas-Abril PV, Selma-Royo M, Prieto-Alamo MJ, Collado MC, Abril N, et al. The role of selenium in shaping mice brain metabolome and selenoproteome through the gut-brain axis by combining metabolomics, metallomics, gene expression, and amplicon sequencing. J Nutr Biochem. 2023;117:109323. doi:https://doi.org/10.1016/j.jnutbio.2023.109323 [Google Scholar] [PubMed] [CrossRef]
46. Ambati CS, Yuan F, Abu-Elheiga LA, Zhang Y, Shetty V. Identification and quantitation of malonic acid biomarkers of in-born error metabolism by targeted metabolomics. J Am Soc Mass Spectr. 2017;28(5):929–38. doi:https://doi.org/10.1007/s13361-017-1631-1 [Google Scholar] [PubMed] [CrossRef]
47. Singh U, Alsuhaymi S, Al-Nemi R, Emwas AH, Jaremko M. Compound-specific 1D H-1 NMR pulse sequence selection for metabolomics analyses. ACS Omega. 2023;8(26):23651–63. doi:https://doi.org/10.1021/acsomega.3c01688 [Google Scholar] [PubMed] [CrossRef]
48. Song XL, Feng G, Li LL, Li W, Wu ZG, Wang WJ, et al. H-NMR-based metabolomics study on the effects of raw and processed radix Wikstroemia indica on endogenous metabolites in rat plasma. Pak J Pharm Sci. 2023;36(3):801–9. doi:https://doi.org/10.36721/Pjps.2023.36.3.Reg.801-809.1 [Google Scholar] [CrossRef]
49. Chen S, Liu L, Jiang HX, Sun Q, Zhang L, Liu JQ, et al. UPLC-Q-TOF-MS/MS-based urine metabolomics studies on the toxicity and detoxication of Tripterygium wilfordii Hook. f. after roasting. J Pharmaceut Biomed. 2023;234:115573. doi:https://doi.org/10.1016/j.jpba.2023.115573 [Google Scholar] [PubMed] [CrossRef]
50. Farag MA, Baky MH, Morgan I, Khalifa MR, Rennert R, Mohamed OG, et al. Comparison of Balanites aegyptiaca parts: metabolome providing insights into plant health benefits and valorization purposes as analyzed using multiplex GC-MS, LC-MS, NMR-based metabolomics, and molecular networking. RSC Adv. 2023;13(31):21471–93. doi:https://doi.org/10.1039/D3RA03141A [Google Scholar] [PubMed] [CrossRef]
51. Jesus CSH, Bispo DSC, Oliveira MB, Mano JF, Gil AM. Unveiling lipid metabolism underlying aging and osteogenesis of mesenchymal stem cells through 1h-nmr metabolomics. Tissue Eng Pt A. 2023;29(11–12):521–2. doi:https://doi.org/10.1039/D0AN02260H [Google Scholar] [PubMed] [CrossRef]
52. Madrid-Gambin F, Oller S, Marco S, Pozo OJ, Andres-Lacueva C, Llorach R. Quantitative plasma profiling by H-1 NMR-based metabolomics: impact of sample treatment. Front Mol Biosci. 2023;10:1125582. doi:https://doi.org/10.3389/fmolb.2023.1125582 [Google Scholar] [PubMed] [CrossRef]
53. Nagarajan K, Ibrahim B, Bawadikji AA, Khaw KY, Tong WY, Leong CR, et al. Characterization of metabolites in an endophytic fungus Diaporthe fraxini via NMR-based metabolomics and cholinesterase inhibitory activity. Appl Biochem Microbiol. 2023;59(3):316–22. doi:https://doi.org/10.1134/S0003683823030134 [Google Scholar] [CrossRef]
54. Bester R, Stander Z, Mason S, Keane KM, Howatson G, Clifford T, et al. The metabolic recovery of marathon runners: an untargeted H-1-NMR metabolomics perspective. Front Physiol. 2023;14:1117687. doi:https://doi.org/10.3389/fphys.2023.1117687 [Google Scholar] [PubMed] [CrossRef]
55. Polachini GM, de Castro TB, Smarra LFS, Henrique T, de Paula CHD, Severino P, et al. Plasma metabolomics of oral squamous cell carcinomas based on NMR and MS approaches provides biomarker identification and survival prediction. Sci Rep. 2023;13(1):8588. doi:https://doi.org/10.1038/s41598-023-34808-2 [Google Scholar] [PubMed] [CrossRef]
56. Hussain M, Girelli CR, Verweire D, Oehl MC, Avendano MS, Scortichini M, et al. H-1-NMR metabolomics study after foliar and endo-therapy treatments of xylella fastidiosa subsp. pauca infected olive trees: medium time monitoring of field experiments. Plants. 2023;12(10):1946. doi:https://doi.org/10.3390/plants12101946 [Google Scholar] [PubMed] [CrossRef]
57. Wang JL, Lu YS, Ni YS, Zhu L, Fang S. Sterilization of black carrot juice by thermosonication: compound changes revealed by NMR and GC-MS metabolomics methods. J Food Process Eng. 2023;46(11):e14430. doi:https://doi.org/10.1111/jfpe.14430 [Google Scholar] [CrossRef]
58. LeGouellec A, Moyne O, Meynet E, Toussaint B, Fauvelle F. High-resolution magic angle spinning NMR-Based metabolomics revealing metabolic changes in lung of mice infected with P. aeruginosa consistent with the degree of disease severity. J Proteome Res. 2018;17(10):3409–17. doi:https://doi.org/10.1021/acs.jproteome.8b00306 [Google Scholar] [PubMed] [CrossRef]
59. Tilgner M, Vater TS, Habbel P, Cheng LL. High-resolution magic angle spinning (HRMAS) NMR methods in metabolomics. Methods Mol Biol. 2019;2037:49–67. doi:https://doi.org/10.1007/978-1-4939-9690-2 [Google Scholar] [CrossRef]
60. Chang YF, Gong WX, Zheng YH, Li JW, Zhou YZ, Qin XM, et al. Urinary metabolomics study of the effects of Scutellaria baicalensis Georgi ethanol extract on D-galactose-induced rats. Yao xue Xue Bao = Acta Pharmaceut Sin. 2016;51(1):86–92. Available from: http://www.ncbi.nlm.nih.gov/pubmed/27405167. [Accessed 2016]. [Google Scholar]
61. Derveaux E, Geubbelmans M, Criel M, Demedts I, Himpe U, Tournoy K, et al. NMR-metabolomics reveals a metabolic shift after surgical resection of non-small cell lung cancer. Cancers. 2023;15(7):2127. doi:https://doi.org/10.3390/cancers15072127 [Google Scholar] [PubMed] [CrossRef]
62. Carrola J, Bastos V, Jarak I, Oliveira-Silva R, Malheiro E, Daniel-da-Silva AL, et al. Metabolomics of silver nanoparticles toxicity in HaCaT cells: structure-activity relationships and role of ionic silver and oxidative stress. Nanotoxicology. 2016;10(8):1105–17. doi:https://doi.org/10.1080/17435390.2016.1177744 [Google Scholar] [PubMed] [CrossRef]
63. Liu CC, Wu YF, Feng GM, Gao XX, Zhou YZ, Hou WJ, et al. Plasma-metabolite-biomarkers for the therapeutic response in depressed patients by the traditional Chinese medicine formula Xiaoyaosan: a 1H NMR-based metabolomics approach. J Affect Disord. 2015;185(2):156–63. doi:https://doi.org/10.1016/j.jad.2015.05.005 [Google Scholar] [PubMed] [CrossRef]
64. Bernacchioni C, Ghini V, Cencetti F, Japtok L, Donati C, Bruni P, et al. NMR metabolomics highlights sphingosine kinase-1 as a new molecular switch in the orchestration of aberrant metabolic phenotype in cancer cells. Mol Oncol. 2017;11(5):517–33. doi:https://doi.org/10.1002/1878-0261.12048 [Google Scholar] [PubMed] [CrossRef]
65. Gooda Sahib Jambocus N, Saari N, Ismail A, Khatib A, Mahomoodally MF, Abdul Hamid A. An investigation into the antiobesity effects of Morinda citrifolia L. leaf extract in high fat diet induced obese rats using a 1H NMR metabolomics approach. J Diabetes Res. 2016;2016:2391592. doi:https://doi.org/10.1155/2016/2391592 [Google Scholar] [PubMed] [CrossRef]
66. Skorupa A, Ponski M, Ciszek M, Cichon B, Klimek M, Witek A, et al. Grading of endometrial cancer using H-1 HR-MAS NMR-based metabolomics. Sci Rep. 2021;11(1):18160. doi:https://doi.org/10.1038/s41598-021-97505-y [Google Scholar] [PubMed] [CrossRef]
67. Wong A. A roadmap to high-resolution standard microcoil MAS NMR spectroscopy for metabolomics. Nmr Biomed. 2023;36(4):e4683. doi:https://doi.org/10.1002/nbm.4683 [Google Scholar] [PubMed] [CrossRef]
68. Yoon D, Shin WC, Lee YS, Kim S, Baek NI, Lee DY. A comparative study on processed panax ginseng products using HR-MAS NMR-based metabolomics. Molecules. 2020;25(6):1390. doi:https://doi.org/10.3390/molecules25061390 [Google Scholar] [PubMed] [CrossRef]
69. Du QY, He M, Gao X, Yu X, Zhang JN, Shi J, et al. Geographical discrimination of Flos Trollii by GC-MS and UHPLC-HRMS-based untargeted metabolomics combined with chemometrics. J Pharmaceut Biomed. 2023;234(20):115550. doi:https://doi.org/10.1016/j.jpba.2023.115550 [Google Scholar] [PubMed] [CrossRef]
70. Sharma H, Ramanathan R. GC-MS-based metabolomics approach reveals metabolic variations between probiotics incorporated cow and goat milk yoghurt. Int J Dairy Technol. 2023;76(3):521–32. doi:https://doi.org/10.1111/1471-0307.12979 [Google Scholar] [CrossRef]
71. Gao Y, Zhu JF, Sun MY, Wang SM, Liu HM. Metabolomics study based on GC-MS reveals a protective function of luteolin against glutamate-induced PC12 cell injury. Biomed Chromatogr. 2023;37(2):e5537. doi:https://doi.org/10.1002/bmc.5537 [Google Scholar] [PubMed] [CrossRef]
72. Han R, Wang M, Wang L, Zhang YC, Li X, Hou YJ, et al. GC/MS-based urine metabolomics study on the ameliorative effect of Xanthoceras sorbifolia extract on Alzheimer’s disease in mice. Evid-Based Compl Alt. 2022;2022:3390034. doi:https://doi.org/10.1155/2022/3390034 [Google Scholar] [PubMed] [CrossRef]
73. Jiang B, Yang LR, Luo XP, Huang RY, Jiao WW, Zhong XX, et al. Aroma formation and dynamic changes during Sichuan black tea processing by GC-MS-based metabolomics. Fermentation. 2023;9(7):686. doi:https://doi.org/10.3390/fermentation9070686 [Google Scholar] [CrossRef]
74. Mhlongo MI, Piater LA, Dubery IA. Profiling of volatile organic compounds from four plant growth-promoting rhizobacteria by SPME-GC-MS: a metabolomics study. Metabolites. 2022;12(8):763. doi:https://doi.org/10.3390/metabo12080763 [Google Scholar] [PubMed] [CrossRef]
75. Zhu GX, Wang Y, Wang W, Shang F, Pei B, Zhao Y, et al. Untargeted GC-MS-based metabolomics for early detection of colorectal cancer. Front Oncol. 2021;11:729512. doi:https://doi.org/10.3389/fonc.2021.729512 [Google Scholar] [PubMed] [CrossRef]
76. Samokhin AS, Matyushin DD. How searching against multiple libraries can lead to biased results in GC/MS-based metabolomics. Rapid Commun Mass Spectr. 2023;37(3):e9437. doi:https://doi.org/10.1002/rcm.9437 [Google Scholar] [PubMed] [CrossRef]
77. Yang Q, Shi BH, Tian GL, Niu QQ, Tang J, Linghu DD, et al. GC-MS urinary metabolomics analysis of inherited metabolic diseases and stable metabolic biomarker screening by a comprehensive chemometric method. Microchem J. 2021;168:106350. doi:https://doi.org/10.1016/j.microc.2021.106350 [Google Scholar] [CrossRef]
78. Yin XL, Fu WJ, Chen Y, Zhou RF, Sun WQ, Ding BM, et al. GC-MS-based untargeted metabolomics reveals the key volatile organic compounds for discriminating grades of Yichang big-leaf green tea. LWT-Food Sci Technol. 2022;171:114148. doi:https://doi.org/10.1016/j.lwt.2022.114148 [Google Scholar] [CrossRef]
79. Malla MA, Dubey A, Kumar A, Yadav S, Kumari S. Modeling and optimization of chlorpyrifos and glyphosate biodegradation using RSM and ANN: elucidating their degradation pathways by GC-MS based metabolomics. Ecotox Environ Safe. 2023;252:114628. doi:https://doi.org/10.1016/j.ecoenv.2023.114628 [Google Scholar] [PubMed] [CrossRef]
80. Bu T, Zhang M, Lee SH, Cheong YE, Park Y, Kim KH, et al. GC-TOF/MS-based metabolomics for comparison of volar and non-volar skin types. Metabolites. 2022;12(8):717. doi:https://doi.org/10.3390/metabo12080717 [Google Scholar] [PubMed] [CrossRef]
81. Kumar M, Agrawal PK, Roy P, Sircar D. GC-MS-based metabolomics reveals dynamic changes in the nutritionally important metabolites in coconut meat during nut maturation. J Food Compos Anal. 2022;114:104869. doi:https://doi.org/10.1016/j.jfca.2022.104869 [Google Scholar] [CrossRef]
82. Cui CM, Zhu L, Wang Q, Liu RJ, Xie DD, Guo YJ, et al. A GC-MS-based untargeted metabolomics approach for comprehensive metabolic profiling of vancomycin-induced toxicity in mice. Heliyon. 2022;8(7):9869. doi:https://doi.org/10.1016/j.heliyon.2022.e09869 [Google Scholar] [PubMed] [CrossRef]
83. Hanafi RS, Lammerhofer M. Quality-by-design approach for development of aqueous headspace microextraction GC-MS method for targeted metabolomics of small aldehydes in plasma of cardiovascular patients. Anal Chim Acta. 2022;1221:340176. doi:https://doi.org/10.1016/j.aca.2022.340176 [Google Scholar] [PubMed] [CrossRef]
84. Sobrinho ACG, Corpes RS, Santos KIPD, Barra IMM, Miyagawa HK, Santos AS. Untargeted GC-MS Metabolomics applied to wild leaves and callus produced by plant tissue culture of Hibiscus sabdariffa L. Arab J Chem. 2022;15(9):104103. doi:https://doi.org/10.1016/j.arabjc.2022.104103 [Google Scholar] [CrossRef]
85. Chen C, Meng XX, Zhu Y, Zhang JX, Wang RL. Early Identification of serum biomarkers and pathways of sepsis through GC-MS-based metabolomics analysis. Front Biosci (Landmark Ed). 2023;28(7):145. doi:https://doi.org/10.31083/j.fbl2807145 [Google Scholar] [PubMed] [CrossRef]
86. Manhas A, Kaul V. Metabolomics of above and below ground parts of commelina benghalensis an amphicarpic weed using GC-MS. Natl Acad Sci Lett. 2023https://doi.org/10.1007/s40009-023-01339-3 [Google Scholar] [CrossRef]
87. Wen S, Sun LL, Zhang SW, Chen ZZ, Chen RH, Li ZG, et al. The formation mechanism of aroma quality of green and yellow teas based on GC-MS/MS metabolomics. Food Res Int. 2023;172:113137. doi:https://doi.org/10.1016/j.foodres.2023.113137 [Google Scholar] [PubMed] [CrossRef]
88. Han S, Micallef SA. Environmental metabolomics of the tomato plant surface provides insights on salmonella enterica colonization. Appl Environ Microbiol. 2016;82(10):3131–42. doi:https://doi.org/10.1128/AEM.00435-16 [Google Scholar] [PubMed] [CrossRef]
89. Lin FK, Long CL. GC-TOF-MS-based metabolomics correlated with bioactivity assays unveiled seasonal variations in leaf essential oils of two species in Garcinia L. Ind Crop Prod. 2023;194:116356. doi:https://doi.org/10.1016/j.indcrop.2023.116356 [Google Scholar] [CrossRef]
90. Moreira OBD, de Souza JCQ, Candido JMB, do Nascimento MP, Chellini PR, de Lemos LM, et al. Factorial design applied to LC-ESI-QTOF mass spectrometer parameters for untargeted metabolomics. Anal Methods. 2023;15(20):2512–21. doi:https://doi.org/10.1039/D3AY00094J [Google Scholar] [PubMed] [CrossRef]
91. Tang YF, Sun LX, Zhao Y, Yao JC, Feng Z, Liu Z, et al. UHPLC-ESI-QE-orbitrap-MS based metabolomics reveals the antioxidant mechanism of icaritin on mice with cerebral ischemic reperfusion. Peerj. 2023;11:e14483. doi:https://doi.org/10.7717/peerj.14483 [Google Scholar] [PubMed] [CrossRef]
92. Calderon-Santiago M, Priego-Capote F, Jurado-Gamez B, Luque de Castro MD. Optimization study for metabolomics analysis of human sweat by liquid chromatography-tandem mass spectrometry in high resolution mode. J Chromatogr A. 2014;1333:70–8. doi:https://doi.org/10.1016/j.chroma.2014.01.071 [Google Scholar] [PubMed] [CrossRef]
93. Tobolkina E, Gonzalez-Ruiz V, Meister I, de Figueiredo M, Guillarme D, Boccard J, et al. Challenges in ESI-MS-based untargeted metabolomics. Chimia. 2022;76(1–2):90–100. doi:https://doi.org/10.2533/chimia.2022.90 [Google Scholar] [PubMed] [CrossRef]
94. Youssef FS, Sobeh M, Dmirieh M, Bogari HA, Koshak AE, Wink M, et al. Metabolomics-based profiling of clerodendrum speciosum (Lamiaceae) leaves using LC/ESI/MS-MS and in vivo evaluation of its antioxidant activity using Caenorhabditis elegans model. Antioxidants. 2022;11(2):330. doi:https://doi.org/10.3390/antiox11020330 [Google Scholar] [PubMed] [CrossRef]
95. Wang HM, Yin YD, Zhu ZJ. Encoding LC-MS-based untargeted metabolomics data into images toward AI-based clinical diagnosis. Anal Chem. 2023;95(16):6533–41. doi:https://doi.org/10.1021/acs.analchem.2c05079 [Google Scholar] [PubMed] [CrossRef]
96. Chen CJ, Lee DY, Yu JX, Lin YN, Lin TM. Recent advances in LC-MS-based metabolomics for clinical biomarker discovery. Mass Spectrom Rev. 2022;42(6):2349–78. doi:https://doi.org/10.1002/mas.21785 [Google Scholar] [PubMed] [CrossRef]
97. Hamade K, Fliniaux O, Fontaine JX, Molinie R, Nnang EO, Bassard S, et al. NMR and LC-MS-based metabolomics to study osmotic stress in lignan-deficient flax. Molecules. 2021;26(3):767. doi:https://doi.org/10.3390/molecules26030767 [Google Scholar] [PubMed] [CrossRef]
98. Teul J, Deja S, Celinska-Janowicz K, Zabek A, Mlynarz P, Barc P, et al. LC-QTOF-MS and H-1 NMR metabolomics verifies potential use of greater omentum for klebsiella pneumoniae biofilm eradication in rats. Pathogens. 2020;9(5):399. doi:https://doi.org/10.3390/pathogens9050399 [Google Scholar] [PubMed] [CrossRef]
99. Celebier M, Nenni M. Recent developments in CE-MS based metabolomics. Curr Anal Chem. 2021;17(9):1229–42. doi:https://doi.org/10.2174/1573411016999200709133339 [Google Scholar] [CrossRef]
100. Naumann L, Haun A, Hochsmann A, Mohr M, Novak M, Flottmann D, et al. Augmented region of interest for untargeted metabolomics mass spectrometry (AriumMS) of multi-platform-based CE-MS and LC-MS data. Anal Bioanal Chem. 2023;415(16):3137–54. doi:https://doi.org/10.1007/s00216-023-04715-6 [Google Scholar] [PubMed] [CrossRef]
101. Hirayama A, Soga T. CE-MS in metabolomics. In: Capillary Electrophoresis-Mass Spectrometry (CE-MSPrinciples and applications. 2016. 293-314. https://doi.org/10.1002/9783527693801 [Google Scholar] [CrossRef]
102. Ortiz-Villanueva E, Benavente F, Pina B, Sanz-Nebot V, Tauler R, Jaumot J. Knowledge integration strategies for untargeted metabolomics based on MCR-ALS analysis of CE-MS and LC-MS data. Anal Chim Acta. 2017;978:10–23. doi:https://doi.org/10.1016/j.aca.2017.04.049 [Google Scholar] [PubMed] [CrossRef]
103. Garcia-Canas V, Simo C. CE-MS workflows for metabolomics: a focus on sample preparation. New Dev Mass Spectr. 2018;6:21–52. doi:https://doi.org/10.1039/9781788012737-00021 [Google Scholar] [CrossRef]
104. Wuethrich A, Quirino JP. Stacking or on-line sample concentration in CE-MS for metabolomics. New Dev Mass Spectr. 2018;6:83–112. doi:https://doi.org/10.1039/9781788012737 [Google Scholar] [CrossRef]
105. Ramautar R. Capillary electrophoresis-mass spectrometry for clinical metabolomics. Adv Clin Chem. 2016;74:1–34. doi:https://doi.org/10.1016/bs.acc.2015.12.002 [Google Scholar] [PubMed] [CrossRef]
106. Ramautar R, Somsen GW, de Jong GJ. CE-MS for metabolomics: developments and applications in the period 2014-2016. Electrophoresis. 2017;38(1):190–202. doi:https://doi.org/10.1002/elps.201600370 [Google Scholar] [PubMed] [CrossRef]
107. Sugishita T, Tokunaga M, Kami K, Terai K, Yamamoto H, Shinohara H, et al. Determination of the minimum sample amount for capillary electrophoresis-fourier transform mass spectrometry (CE-FTMS)-based metabolomics of colorectal cancer biopsies. Biomedicines. 2023;11(6):1706. doi:https://doi.org/10.3390/biomedicines11061706 [Google Scholar] [PubMed] [CrossRef]
108. Mokosch AS, Gerbig S, Grevelding CG, Haeberlein S, Spengler B. High-resolution AP-SMALDI MSI as a tool for drug imaging in. Anal Bioanal Chem. 2021;413(10):2755–66. doi:https://doi.org/10.1007/s00216-021-03230-w [Google Scholar] [PubMed] [CrossRef]
109. Müller MA, Kompauer M, Strupat K, Heiles S, Spengler B. Implementation of a high-repetition-rate laser in an AP-SMALDI MSI system for enhanced measurement performance. J Am Soc Mass Spectr. 2021;32(2):465–72. doi:https://doi.org/10.1021/jasms.0c00368 [Google Scholar] [PubMed] [CrossRef]
110. Shen Y, Wu SD, Chen Y, Li XY, Zhu Q, Nakayama K, et al. Alterations in gut microbiome and metabolomics in chronic hepatitis B infection-associated liver disease and their impact on peripheral immune response. Gut Microbes. 2023;15(1):3073–84. doi:https://doi.org/10.1080/19490976.2022.2155018 [Google Scholar] [PubMed] [CrossRef]
111. Triastuti A, Vansteelandt M, Barakat F, Amasifuen C, Jargeat P, Haddad M. Untargeted metabolomics to evaluate antifungal mechanism: a study of Cophinforma mamane and Candida albicans interaction. Nat Product Bioprosp. 2023;13:1. doi:https://doi.org/10.1007/s13659-022-00365-w [Google Scholar] [PubMed] [CrossRef]
112. Wang M, Mu CY, Li YL, Wang YX, Ma WY, Ge CH, et al. Foliar application of selenium nanoparticles alleviates cadmium toxicity in maize (Zea mays L.) seedlings: evidence on antioxidant, gene expression, and metabolomics analysis. Sci Total Environ. 2023;899(4):165521. doi:https://doi.org/10.1016/j.scitotenv.2023.165521 [Google Scholar] [PubMed] [CrossRef]
113. Henrottin J, Richez P, Gillard N. In vitro metabolomics analysis using UHPLC-HRMS/MS for vet drug registration: an efficient alternative to in vivo approaches? J Vet Pharmacol Ther. 2023;46(S1):132. doi:https://doi.org/10.1111/jvp.13376 [Google Scholar] [CrossRef]
114. Li ZQ, Gao J, Lin LJ, Zheng ZF, Yan SS, Wang WD, et al. Untargeted metabolomics analysis in drug-naive patients with severe obsessive-compulsive disorder. Front Neurosci-Switz. 2023;17:1148971. doi:https://doi.org/10.3389/fnins.2023.1148971 [Google Scholar] [PubMed] [CrossRef]
115. Malachowska B, Yang WL, Qualman A, Muro I, Boe DM, Lampe JN, et al. Transcriptomics, metabolomics, and in-silico drug predictions for liver damage in young and aged burn victims. Commun Biol. 2023;6(1):597. doi:https://doi.org/10.1038/s42003-023-04964-2 [Google Scholar] [PubMed] [CrossRef]
116. Rundblad A, Christensen JJ, Hustad KS, Bastani NE, Ottestad I, Holven KB, et al. Associations between dietary intake and glucose tolerance in clinical and metabolomics-based metabotypes. Genes Nutr. 2023;18(1):3. doi:https://doi.org/10.1186/s12263-023-00721-6 [Google Scholar] [PubMed] [CrossRef]
117. Zhu J, Cai YM, Yang LY, Li X, Luo SF, Zeng XH, et al. Differential metabolic analysis of Bletilla striata and its mutants based on widely targeted metabolomics and transcriptomics. Ind Crop Prod. 2023;204:117245. doi:https://doi.org/10.1016/j.indcrop.2023.117245 [Google Scholar] [CrossRef]
118. Zhu Z, Song ZH, Cao LT, Wang Y, Zhou WZ, Zhou P, et al. Effects of traditional Chinese medicine formula on ruminal fermentation, enzyme activities and nutrient digestibility of beef cattle. Anim Sci J = Nihon Chikusan Gakkaiho. 2018;89(4):661–71. doi:https://doi.org/10.1111/asj.12978 [Google Scholar] [PubMed] [CrossRef]
119. Wang XJ, Zhang AH, Sun H. Future perspectives of chinese medical formulae: chinmedomics as an effector. OMICS. 2012;16(7–8):414–21. doi:https://doi.org/10.1089/omi.2011.0138 [Google Scholar] [PubMed] [CrossRef]
120. Kong L, Sun Y, Sun H, Zhang AH, Zhang B, Ge N, et al. Chinmedomics strategy for elucidating the pharmacological effects and discovering bioactive compounds from keluoxin against diabetic retinopathy. Front Pharmacol. 2022;13:728256. doi:https://doi.org/10.3389/fphar.2022.728256 [Google Scholar] [PubMed] [CrossRef]
121. Wan CL, Wang XJ, Liu HD, Zhang QY, Yan GL, Li ZE, et al. Characterization of effective constituents in Acanthopanax senticosus fruit for blood deficiency syndrome based on the chinmedomics strategy. Open Chem. 2023;21(1):20220280. doi:https://doi.org/10.1515/chem-2022-0280 [Google Scholar] [CrossRef]
122. Wang ZW, Liu C, Zhang AH, Yan GL, Sun H, Han Y, et al. Discovery of Q-markers of wenxin formula based on a chinmedomics strategy. J Ethnopharmacol. 2022;298:115576. doi:https://doi.org/10.1016/j.jep.2022.115576 [Google Scholar] [PubMed] [CrossRef]
123. Wei WF, Sun H, Liu SB, Lu SW, Zhang AH, Wang WY, et al. Targets and effective constituents of zhizibaipi decoction for treating damp-heat jaundice syndrome based on chinmedomics coupled with UPLC-MS/MS. Front Pharmacol. 2022;13:857361. doi:https://doi.org/10.3389/fphar.2022.857361 [Google Scholar] [PubMed] [CrossRef]
124. Li TP, Wu FF, Zhang AH, Dong H, Ullah I, Lin H, et al. High-throughput chinmedomics strategy discovers the quality markers and mechanisms of wutou decoction therapeutic for rheumatoid arthritis. Front Pharmacol. 2022;13:854087. doi:https://doi.org/10.3389/fphar.2022.854087 [Google Scholar] [PubMed] [CrossRef]
125. Wang J, Wei FY, Wang Y, Liu QZ, He RX, Huang YX, et al. Exploring the quality markers and mechanism of Bushen Huoxue Prescription in prevention and treatment of diabetic retinopathy based on Chinmedomics strategy. J Ethnopharmacol. 2023;306:116131. doi:https://doi.org/10.1016/j.jep.2022.116131 [Google Scholar] [PubMed] [CrossRef]
126. Yan Y, Li JH, Zhang YJ, Wang H, Qin XM, Zhai KF, et al. Screening the effective components of Suanzaoren decoction on the treatment of chronic restraint stress induced anxiety-like mice by integrated chinmedomics and network pharmacology. Phytomedicine. 2023;115:154853. doi:https://doi.org/10.1016/j.phymed.2023.154853 [Google Scholar] [PubMed] [CrossRef]
127. Cheng MJ, Zheng JJ, Cui KS, Luo X, Yang T, Pan ZY, et al. Transcriptomics integrated with metabolomics provides a new strategy for mining key genes in response to low temperature stress in Helictotrichon virescens. Int J Biol Macromol. 2023;242:125070. doi:https://doi.org/10.1016/j.ijbiomac.2023.125070 [Google Scholar] [PubMed] [CrossRef]
128. Xu GX, Li LJ, Zhou J, He MQ, Lyu D, Zhao DY, et al. Integrated transcriptomics and metabolomics analyses reveal key genes and essential metabolic pathways for the acquisition of cold tolerance during dormancy in apple. Environ Exp Bot. 2023;213:105413. doi:https://doi.org/10.1016/j.envexpbot.2023.105413 [Google Scholar] [CrossRef]
129. Jiang ZZ, Zhang HL, Jiao P, Wei XT, Liu SY, Guan SY, et al. The integration of metabolomics and transcriptomics provides new insights for the identification of genes key to auxin synthesis at different growth stages of maize. Int J Mol Sci. 2022;23(21):13195. doi:https://doi.org/10.3390/ijms232113195 [Google Scholar] [PubMed] [CrossRef]
130. Wang YA, Shi JC, Liu K, Wang Y, Xu YJ, Liu YF. Metabolomics and gene expression levels reveal the positive effects of teaseed oil on lifespan and aging process in Caenorhabditis elegans. Food Sci Hum Well. 2023;12(4):1391–401. doi:https://doi.org/10.1016/j.fshw.2022.10.032 [Google Scholar] [CrossRef]
131. Seraj RGM, Tohidfar M, Irani MA, Esmaeilzadeh-Salestani K, Moradian T, Ahmadikhah A, et al. Metabolomics analysis of milk thistle lipids to identify drought-tolerant genes. Sci. 2022;12(1):12827. doi:https://doi.org/10.1038/s41598-022-16887-9 [Google Scholar] [PubMed] [CrossRef]
132. Zhang H, Liu HH, Shu L, Xu HM, Cheng Y, Mao ZT, et al. Metabolomics analysis coupled with weighted gene co-expression network analysis unravels the associations of tricarboxylic acid cycle-intermediates with edible pigments produced by monascus purpureus (Hong Qu). Foods. 2022;11(14):2168. doi:https://doi.org/10.3390/foods11142168 [Google Scholar] [PubMed] [CrossRef]
133. Caesar LK, Butun FA, Robey MT, Ayon NJ, Gupta R, Dainko D, et al. Correlative metabologenomics of 110 fungi reveals metabolite-gene cluster pairs. Nat Chem Biol. 2023;19(7):846–54. doi:https://doi.org/10.1038/s41589-023-01276-8 [Google Scholar] [PubMed] [CrossRef]
134. Maimone NM, Pozza MC, de Oliveira LFP, Rojas-Villalta D, de Lira SP, Barrientos L, et al. Metabologenomics analysis of Pseudomonas sp. So3.2b, an Antarctic strain with bioactivity against Rhizoctonia solani. Front Microbiol. 2023;14:1187321. doi:https://doi.org/10.3389/fmicb.2023.1187321 [Google Scholar] [PubMed] [CrossRef]
135. Melinda YN, Widada J, Wahyuningsih TD, Febriansah R, Damayanti E, Mustofa M. Metabologenomics approach to the discovery of novel compounds from Streptomyces sp. GMR22 as anti-SARS-CoV-2 drugs. Heliyon. 2021;7(11):e08308. doi:https://doi.org/10.1016/j.heliyon.2021.e08308 [Google Scholar] [PubMed] [CrossRef]
136. Gianazza E, Brioschi M, Iezzi A, Paglia G, Banfi C. Pharmacometabolomics for the study of lipid-lowering therapies: opportunities and challenges. Int J Mol Sci. 2023;24(4):3291. doi:https://doi.org/10.3390/ijms24043291 [Google Scholar] [PubMed] [CrossRef]
137. Li LM, Zang QC, Li XZ, Zhu Y, Wen SJ, He JM, et al. Spatiotemporal pharmacometabolomics based on ambient mass spectrometry imaging to evaluate the metabolism and hepatotoxicity of amiodarone in HepG2 spheroids. J Pharm Anal. 2023;13(5):483–93. doi:https://doi.org/10.1016/j.jpha.2023.04.007 [Google Scholar] [PubMed] [CrossRef]
138. Siani-Rose M, Cox S, Goldstein B, Abrams D, Taylor M, Kurek I. Cannabis-responsive biomarkers: a pharmacometabolomics-based application to evaluate the impact of medical cannabis treatment on children with autism spectrum disorder. Cannabis Cannabinoid. 2023;8(1):126–37. doi:https://doi.org/10.1089/can.2021.0129 [Google Scholar] [PubMed] [CrossRef]
139. Boon TK, Huri HZ, Hashim NM, Ibrahim L, Saiman MZ, Zakaria ZH, et al. Pharmacometabolomics of meformin demonstrated insulin sensitivity related pathways at peak plasma concentration on healthy volunteers—preliminary results. Metabolis. 2021;116:154536. doi:https://doi.org/10.1016/j.metabol.2020.154536 [Google Scholar] [CrossRef]
140. Wang XB, Wang ML, Chu YJ, Zhou PP, Zhang XY, Zou J, et al. Integrated pharmacokinetics and pharmacometabolomics to reveal the synergistic mechanism of a multicomponent Chinese patent medicine, Mailuo Shutong pills against thromboangiitis obliterans. Phytomedicine. 2023;112:154709. doi:https://doi.org/10.1016/j.phymed.2023.154709 [Google Scholar] [PubMed] [CrossRef]
141. Sun Y, Kim JH, Vangipuram K, Hayes DF, Smith EML, Yeomans L, et al. Pharmacometabolomics reveals a role for histidine, phenylalanine, and threonine in the development of paclitaxel-induced peripheral neuropathy. Breast Cancer Res Treat. 2018;171(3):657–66. doi:https://doi.org/10.1007/s10549-018-4862-3 [Google Scholar] [PubMed] [CrossRef]
142. Mostafa AMA, Mostafa H, Sk MASA, Hay YK. Pharmacometabolomics analysis of plasma and urine to identify clopidogrel exposure metabolic biomarkers. Eur Heart J. 2020;41(1):ehz872.084. doi:https://doi.org/10.1093/ehjci/ehz872.084 [Google Scholar] [CrossRef]
143. Wu DY, Li XY, Zhang XH, Han F, Lu X, Liu L, et al. Pharmacometabolomics identifies 3-hydroxyadipic acid, d-galactose, lysophosphatidylcholine (P-16: 0and tetradecenoyl-l-carnitine as potential predictive indicators of gemcitabine efficacy in pancreatic cancer patients. Front Oncol. 2020;9:1524. doi:https://doi.org/10.3389/fonc.2019.01524 [Google Scholar] [PubMed] [CrossRef]
144. Amaro F, Carvalho M, Bastos MD, de Pinho PG, Pinto J. Pharmacometabolomics Applied to personalized medicine in urological cancers. Pharmaceuticals. 2022;15(3):295. doi:https://doi.org/10.3390/ph15030295 [Google Scholar] [PubMed] [CrossRef]
145. Velenosi TJ, Krausz KW, Hamada K, Dorsey TH, Ambs S, Takahashi S, et al. Pharmacometabolomics reveals urinary diacetylspermine as a biomarker of doxorubicin effectiveness in triple negative breast cancer. npj Precis Oncol. 2022;6(1):70. doi:https://doi.org/10.1038/s41698-022-00313-4 [Google Scholar] [PubMed] [CrossRef]
146. Gong MJ, Lu HL, Li LX, Feng MQ, Zou ZJ. Integration of transcriptomics and metabonomics revealed the protective effects of hemp seed oil against methionine-choline-deficient diet-induced non-alcoholic steatohepatitis in mice. Food Funct. 2023;14(4):2096–111. doi:https://doi.org/10.1039/D2FO03054C [Google Scholar] [PubMed] [CrossRef]
147. Li X, Du Y, Tu ZX, Zhang CP, Wang L. Highland barley improves lipid metabolism, liver injury, antioxidant capacities and liver functions in high-fat/cholesterol diet mice based on gut microbiota and LC-MS metabonomics. Food Biosci. 2022;50:102094. doi:https://doi.org/10.1016/j.fbio.2022.102094 [Google Scholar] [CrossRef]
148. Li X, Du Y, Zhang CP, Wang L. Black rice regulates lipid metabolism, liver injury, oxidative stress and adipose accumulation in high-fat/cholesterol diet mice based on gut microbiota and untargeted metabonomics. J Nutr Biochem. 2023;117:109320. doi:https://doi.org/10.1016/j.jnutbio.2023.109320 [Google Scholar] [PubMed] [CrossRef]
149. Swann JR, Claus SP. Nutrimetabonomics: nutritional applications of metabolic profiling. Sci Prog. 2014;97(1):41–7. doi:https://doi.org/10.3184/003685014X13898807933527 [Google Scholar] [PubMed] [CrossRef]
150. Xie H, Hua ZY, Guo MY, Lin SY, Zhou YQ, Weng ZB, et al. Gut microbiota and metabonomics used to explore the mechanism of Qing’e Pills in alleviating osteoporosis. Pharm Biol. 2022;60(1):785–800. doi:https://doi.org/10.1080/13880209.2022.2056208 [Google Scholar] [PubMed] [CrossRef]
151. Xue GH, Zheng ZJ, Liang XB, Zheng YH, Wu HC. Uterine tissue metabonomics combined with 16S rRNA gene sequencing to analyze the changes of gut microbiota in mice with endometritis and the intervention effect of tau interferon. Microbiol Spectr. 2023;11(3). doi:https://doi.org/10.1128/spectrum.00409-23 [Google Scholar] [PubMed] [CrossRef]
152. Montoliu I, Cominetti O, Boulange CL, Berger B, Siddharth J, Nicholson J, et al. Modeling longitudinal metabonomics and microbiota interactions in C57BL/6 mice fed a high fat diet. Anal Chem. 2016;88(15):7617–26. doi:https://doi.org/10.1021/acs.analchem.6b01343 [Google Scholar] [PubMed] [CrossRef]
153. He MS, Yu HS, Lei P, Huang SJ, Ren JN, Fan WJ, et al. Determination of trimethylamine N-oxide and betaine in serum and food by targeted metabonomics. Molecules. 2021;26(5):1334. doi:https://doi.org/10.3390/molecules26051334 [Google Scholar] [PubMed] [CrossRef]
154. Liu SJ, Wu YN, Chan L. Application of metabonomics approach in food safety research-a review. Food Rev Int. 2020;36(6):547–58. doi:https://doi.org/10.1080/87559129.2019.1655571 [Google Scholar] [CrossRef]
155. Li YD, Xiao K, Xiao SY, Wang MM, Pei SS, Liu HL, et al. Difference in intestinal flora and characteristics of plasma metabonomics in pneumoconiosis patients. Metabolites. 2022;12(10):917. doi:https://doi.org/10.3390/metabo12100917 [Google Scholar] [PubMed] [CrossRef]
156. Wang XY, Bao LR, Jiang MY, Li D, Xu L, Bai MR. Toxic mechanism of the Mongolian medicine Hunqile-7 based on metabonomics and the metabolism of intestinal flora. Toxicol Res-UK. 2023;12(1):49–61. doi:https://doi.org/10.1093/toxres/tfac081 [Google Scholar] [PubMed] [CrossRef]
157. Wang RY, Zhao Y, Fang XH, Miao CY, Ren N, Chen Y, et al. Effect of the ketogenic diet on gut microbiome composition and metabolomics in polycystic ovarian syndrome rats induced by letrozole and a high-fat diet. Nutrition. 2023;114:112127. doi:https://doi.org/10.1016/j.nut.2023.112127 [Google Scholar] [PubMed] [CrossRef]
158. Wang YX, Zhang JY, Cao YM, Liu T, Zhang ZK, Zhang BX, et al. Coptis Chinensis-induced changes in metabolomics and gut microbiota in rats. Am J Chinese Med. 2023;51(6):1547–76. doi:https://doi.org/10.1142/S0192415X23500702 [Google Scholar] [PubMed] [CrossRef]
159. Zhang GJ, Zhang JB, Yan S, Hao M, Fei CH, Ji D, et al. Study on the plasma metabolomics of Schisandra chinensis polysaccharide against ulcerative colitis and its correlation with gut microbes metabolism. Chinese J Anal Chem. 2023;51(9):100244. doi:https://doi.org/10.1016/j.cjac.2023.100244 [Google Scholar] [CrossRef]
160. Vangeenderhuysen P, van Arnhem J, Pomian B, de Graeve M, de Commer L, Falony G, et al. Dual UHPLC-HRMS metabolomics and lipidomics and automated data processing workflow for comprehensive high-throughput gut phenotyping. Anal Chem. 2023;95(22):8461–8. doi:https://doi.org/10.1021/acs.analchem.2c05371 [Google Scholar] [PubMed] [CrossRef]
161. Wan D, Liang XJ, Yang LM, He D, Du Q, Zhang WP, et al. Integration of gut microbiota and metabolomics for the hematopoiesis of Siwu paste on anemia rats. Heliyon. 2023;9(7):e18024. doi:https://doi.org/10.1016/j.heliyon.2023.e18024 [Google Scholar] [PubMed] [CrossRef]
162. Wang H, Shen Q, Fu YX, Liu ZY, Wu T, Wang C, et al. Effects on diabetic mice of consuming lipid extracted from foxtail millet (Setaria italicagut microbiota analysis and serum metabolomics. J Agr Food Chem. 2023;71(26):10075–86. doi:https://doi.org/10.1021/acs.jafc.3c02179 [Google Scholar] [PubMed] [CrossRef]
163. Liu WT, Pan YF, Yang L, Xie Y, Chen XY, Chang J, et al. Developmental toxicity of TCBPA on the nervous and cardiovascular systems of zebrafish (Danio rerioa combination of transcriptomic and metabolomics. J Environ Sci. 2023;127:197–209. doi:https://doi.org/10.1016/j.jes.2022.04.022 [Google Scholar] [PubMed] [CrossRef]
164. Mandal P, Lanaridi O, Warth B, Ansari KM. Metabolomics as an emerging approach for deciphering the biological impact and toxicity of food contaminants: the case of mycotoxins. Crit Rev Food Sci. 2023. doi:https://doi.org/10.1080/10408398.2023.2217451 [Google Scholar] [PubMed] [CrossRef]
165. Wilhelmi P, Giri V, Zickgraf FM, Haake V, Henkes S, Driemert P, et al. A metabolomics approach to reveal the mechanism of developmental toxicity in zebrafish embryos exposed to 6-propyl-2-thiouracil. Chem-Biol Interact. 2023;382:110565. doi:https://doi.org/10.1016/j.cbi.2023.110565 [Google Scholar] [PubMed] [CrossRef]
166. Qi L, Li YP, Dong YM, Ma SL, Li G. Integrated metabolomics and transcriptomics reveal glyphosate based-herbicide induced reproductive toxicity through disturbing energy and nucleotide metabolism in mice testes. Environ Toxicol. 2023;38(8):1811–23. doi:https://doi.org/10.1002/tox.23808 [Google Scholar] [PubMed] [CrossRef]
167. Mashabela MD, Masamba P, Kappo AP. Applications of metabolomics for the elucidation of abiotic stress tolerance in plants: a special focus on osmotic stress and heavy metal toxicity. Plants. 2023;12(2):269. doi:https://doi.org/10.3390/plants12020269 [Google Scholar] [PubMed] [CrossRef]
168. Wu HF, Zhang WX, Lin H, Ye QM, Guo JY, Quan SJ. The pseudotargeted metabolomics study on the toxicity of fuzi using ultraperformance liquid chromatography tandem mass spectrometry. Evid-Based Compl Alt. 2022;2022:6539675. doi:https://doi.org/10.1155/2022/6539675 [Google Scholar] [PubMed] [CrossRef]
169. Bruhns T, Timm S, Sokolova IM. Metabolomics-based assessment of nanoparticles (nZnO) toxicity in an infaunal marine annelid, the lugworm Arenicola marina (Annelida: Sedentaria). Sci Total Environ. 2023;858:160039. doi:https://doi.org/10.1016/j.scitotenv.2022.160039 [Google Scholar] [PubMed] [CrossRef]
170. Yang YJ, Wang X, Song ZH, Zheng YF, Ji SP. Proteomics and metabolomics analysis reveals the toxicity of ZnO quantum dots on human SMMC-7721 Cells. Int J Nanomed. 2023;2023(18):277–91. doi:https://doi.org/10.2147/IJN.S389535 [Google Scholar] [PubMed] [CrossRef]
171. Yu Y, Yao CL, Zhang JQ, Bi QR, Wei WL, Li ZW, et al. Comprehensive quality evaluation of Aconiti Lateralis Radix Praeparata based on pseudotargeted metabolomics and simultaneous determination of fifteen components, and development of new processed products of black slices with less toxicity. J Pharmaceut Biomed. 2023;228:115295. doi:https://doi.org/10.1016/j.jpba.2023.115295 [Google Scholar] [PubMed] [CrossRef]
172. Hussein HA, Kassim MNI, Maulidiani M, Abas F, Abdullah MA. Cytotoxicity and H-1 NMR metabolomics analyses of microalgal extracts for synergistic application with Tamoxifen on breast cancer cells with reduced toxicity against Vero cells. Heliyon. 2022;8(3):e09192. doi:https://doi.org/10.1016/j.heliyon.2022.e09192 [Google Scholar] [PubMed] [CrossRef]
173. Jing N, Peng J, Yang X, Wang XZ, Liu Q, Wang HL, et al. Metabolomics analysis of chronic exposure to dimethylarsenic acid in mice and toxicity assessment of organic arsenic in food. ACS Omega. 2022;7(40):35774–82. doi:https://doi.org/10.1021/acsomega.2c03806 [Google Scholar] [PubMed] [CrossRef]
174. Zhang R, Zhao ZG, Li MS, Luo L, Wang SH, Guo K, et al. Metabolomics analysis reveals the response mechanism to carbonate alkalinity toxicity in the gills of Eriocheir sinensis. Comp Biochem Phys C. 2023;263:109487. doi:https://doi.org/10.1016/j.cbpc.2022.109487 [Google Scholar] [PubMed] [CrossRef]
175. Chasapi SA, Karagkouni E, Kalavrizioti D, Vamvakas S, Zompra A, Takis PG, et al. NMR-based metabolomics in differential diagnosis of chronic kidney disease (CKD) subtypes. Metabolites. 2022;12(6):490. doi:https://doi.org/10.3390/metabo12060490 [Google Scholar] [PubMed] [CrossRef]
176. Wang YY, Sun YP, Luo YM, Peng DH, Li X, Yang BY, et al. Biomarkers for the clinical diagnosis of Alzheimer’s disease: metabolomics analysis of brain tissue and blood. Front Pharmacol. 2021;12:700587. doi:https://doi.org/10.3389/fphar.2021.700587 [Google Scholar] [PubMed] [CrossRef]
177. Al-Sulaiti H, Almaliti J, Naman CB, Al Thani AA, Yassine HM. Metabolomics approaches for the diagnosis, treatment, and better disease management of viral infections. Metabolites. 2023;13(8):948. doi:https://doi.org/10.3390/metabo13080948 [Google Scholar] [PubMed] [CrossRef]
178. Zhou SF, Xu HS, Zhu JY, Fan XS, Zhang JM. Clinical efficacy and metabolomics study of Wendan decoction in the treatment of phlegm-dampness obstructive sleep apnea-hypopnea syndrome with type 2 diabetes mellitus. J Ethnopharmacol. 2023;317:116775. doi:https://doi.org/10.1016/j.jep.2023.116775 [Google Scholar] [PubMed] [CrossRef]
179. Dodangeh S, Taghizadeh H, Hosseinkhani S, Khashayar P, Pasalar P, Meybodi HRA, et al. Metabolomics signature of cardiovascular disease in patients with diabetes, a narrative review. J Diabetes Metab Dis. 2023;22:985–94. doi:https://doi.org/10.1007/s40200-023-01256-8 [Google Scholar] [PubMed] [CrossRef]
180. El-Derany MO, Hanna DMF, Youshia J, Elmowafy E, Farag MA, Azab SS. Metabolomics-directed nanotechnology in viral diseases management: COVID-19 a case study. Pharmacol Rep. 2023;75(5):1045–65. doi:https://doi.org/10.1007/s43440-023-00517-w [Google Scholar] [PubMed] [CrossRef]
Cite This Article
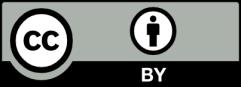