Open Access
ARTICLE
Model Agnostic Meta-Learning (MAML)-Based Ensemble Model for Accurate Detection of Wheat Diseases Using Vision Transformer and Graph Neural Networks
1 Department of Creative Technologies, Faculty of Computing and Artificial Intelligence, Air University, Islamabad, 44000, Pakistan
2 Department of Computer Engineering, College of Computer and Information Sciences, King Saud University, P. O. Box 51178, Riyadh, 11543, Saudi Arabia
3 Department of Computer Engineering, Bahria School of Engineering and Applied Sciences, Bahria University, Islamabad, 44000, Pakistan
4 Laboratory for Neuro & Psychophysiology, KU Leuven, Leuven, 3000, Belgium
* Corresponding Authors: Syed Muhammad Usman. Email: ; Khursheed Aurangzeb. Email:
(This article belongs to the Special Issue: Advanced Artificial Intelligence and Machine Learning Frameworks for Signal and Image Processing Applications)
Computers, Materials & Continua 2024, 79(2), 2795-2811. https://doi.org/10.32604/cmc.2024.049410
Received 06 January 2024; Accepted 29 March 2024; Issue published 15 May 2024
Abstract
Wheat is a critical crop, extensively consumed worldwide, and its production enhancement is essential to meet escalating demand. The presence of diseases like stem rust, leaf rust, yellow rust, and tan spot significantly diminishes wheat yield, making the early and precise identification of these diseases vital for effective disease management. With advancements in deep learning algorithms, researchers have proposed many methods for the automated detection of disease pathogens; however, accurately detecting multiple disease pathogens simultaneously remains a challenge. This challenge arises due to the scarcity of RGB images for multiple diseases, class imbalance in existing public datasets, and the difficulty in extracting features that discriminate between multiple classes of disease pathogens. In this research, a novel method is proposed based on Transfer Generative Adversarial Networks for augmenting existing data, thereby overcoming the problems of class imbalance and data scarcity. This study proposes a customized architecture of Vision Transformers (ViT), where the feature vector is obtained by concatenating features extracted from the custom ViT and Graph Neural Networks. This paper also proposes a Model Agnostic Meta Learning (MAML) based ensemble classifier for accurate classification. The proposed model, validated on public datasets for wheat disease pathogen classification, achieved a test accuracy of 99.20% and an F1-score of 97.95%. Compared with existing state-of-the-art methods, this proposed model outperforms in terms of accuracy, F1-score, and the number of disease pathogens detection. In future, more diseases can be included for detection along with some other modalities like pests and weed.Keywords
Cite This Article
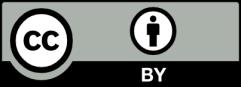