Open Access
ARTICLE
Deep Learning-Based Classification of Fruit Diseases: An Application for Precision Agriculture
1 Department of Computer Science, HITEC University, Taxila, 47040, Pakistan
2 Department of Computer Science, COMSATS University Islamabad, Wah Campus, Wah Cantt, 47080, Pakistan
3 Department of Computer Science, COMSATS University Islamabad, Attock Campus, Attock, Pakistan
4 Department of Computer Science and Engineering, Soonchunhyang University, Asan, South Korea
5 Department of Mathematics and Computer Science, Faculty of Science, Beirut Arab University, Beirut, Lebanon
* Corresponding Author: Seifedine Kadry. Email:
(This article belongs to the Special Issue: Artificial Intelligence based Smart precision agriculture with analytic pattern in sustainable environments using IoT)
Computers, Materials & Continua 2021, 66(2), 1949-1962. https://doi.org/10.32604/cmc.2020.012945
Received 18 July 2020; Accepted 05 October 2020; Issue published 26 November 2020
Abstract
Agriculture is essential for the economy and plant disease must be minimized. Early recognition of problems is important, but the manual inspection is slow, error-prone, and has high manpower and time requirements. Artificial intelligence can be used to extract fruit color, shape, or texture data, thus aiding the detection of infections. Recently, the convolutional neural network (CNN) techniques show a massive success for image classification tasks. CNN extracts more detailed features and can work efficiently with large datasets. In this work, we used a combined deep neural network and contour feature-based approach to classify fruits and their diseases. A fine-tuned, pretrained deep learning model (VGG19) was retrained using a plant dataset, from which useful features were extracted. Next, contour features were extracted using pyramid histogram of oriented gradient (PHOG) and combined with the deep features using serial based approach. During the fusion process, a few pieces of redundant information were added in the form of features. Then, a “relevance-based” optimization technique was used to select the best features from the fused vector for the final classifications. With the use of multiple classifiers, an accuracy of up to 99.6% was achieved on the proposed method, which is superior to previous techniques. Moreover, our approach is useful for 5G technology, cloud computing, and the Internet of Things (IoT).Keywords
Cite This Article
Citations
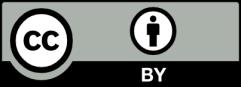