Open Access
ARTICLE
A Deep-CNN Crowd Counting Model for Enforcing Social Distancing during COVID19 Pandemic: Application to Saudi Arabia’s Public Places
1 Department of Computer Science, FCIT, King Abdulaziz University, Jeddah, Saudi Arabia
2 MIRACL-Laboratory, Sfax University, Sfax, Tunisia
3 Department of Computer Science, King Khalid University, Abha, Saudi Arabia
* Corresponding Author: Salma Kammoun Jarraya. Email:
Computers, Materials & Continua 2021, 66(2), 1315-1328. https://doi.org/10.32604/cmc.2020.013522
Received 10 August 2020; Accepted 12 September 2020; Issue published 26 November 2020
Abstract
With the emergence of the COVID19 virus in late 2019 and the declaration that the virus is a worldwide pandemic, health organizations and governments have begun to implement severe health precautions to reduce the spread of the virus and preserve human lives. The enforcement of social distancing at work environments and public areas is one of these obligatory precautions. Crowd management is one of the effective measures for social distancing. By reducing the social contacts of individuals, the spread of the disease will be immensely reduced. In this paper, a model for crowd counting in public places of high and low densities is proposed. The model works under various scene conditions and with no prior knowledge. A Deep CNN model (DCNN) is built based on convolutional neural network (CNN) structure with small kernel size and two fronts. To increase the efficiency of the model, a convolutional neural network (CNN) as the front-end and a multi-column layer with Dilated Convolution as the back-end were chosen. Also, the proposed method accepts images of arbitrary sizes/scales as inputs from different cameras. To evaluate the proposed model, a dataset was created from images of Saudi people with traditional and non-traditional Saudi outfits. The model was also trained and tested on some existing datasets. Compared to current counting methods, the results show that the proposed model has significantly improved efficiency and reduced the error rate. We achieve the lowest MAE by 67%, 32% .and 15.63% and lowest MSE by around 47%, 15% and 8.1% than M-CNN, Cascaded-MTL, and CSRNet respectively.Keywords
Cite This Article
Citations
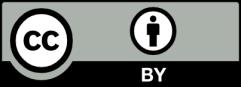