Open Access
ARTICLE
Classification of Positive COVID-19 CT Scans Using Deep Learning
1 Department of Computer Science, HITEC University Taxila, Pakistan
2 College of Computer Science and Engineering, University of Ha’il, Ha’il, Saudi Arabia
3 Division of Computer Science, Mathematics and Science, Collins College of Professional Studies, St.’John’s University, New York, USA
4 Department of Mathematics and Computer Science, Beirut Arab University, Lebanon
5 Department of Computer Science and Engineering, Soonchunhyang University, Asan, South Korea
6 Department of Informatics, University of Leicester, Leicester, UK
* Corresponding Author: Yunyoung Nam. Email:
(This article belongs to the Special Issue: Innovation of Blockchain Technology)
Computers, Materials & Continua 2021, 66(3), 2923-2938. https://doi.org/10.32604/cmc.2021.013191
Received 29 July 2020; Accepted 17 October 2020; Issue published 28 December 2020
Abstract
In medical imaging, computer vision researchers are faced with a variety of features for verifying the authenticity of classifiers for an accurate diagnosis. In response to the coronavirus 2019 (COVID-19) pandemic, new testing procedures, medical treatments, and vaccines are being developed rapidly. One potential diagnostic tool is a reverse-transcription polymerase chain reaction (RT-PCR). RT-PCR, typically a time-consuming process, was less sensitive to COVID-19 recognition in the disease’s early stages. Here we introduce an optimized deep learning (DL) scheme to distinguish COVID-19-infected patients from normal patients according to computed tomography (CT) scans. In the proposed method, contrast enhancement is used to improve the quality of the original images. A pretrained DenseNet-201 DL model is then trained using transfer learning. Two fully connected layers and an average pool are used for feature extraction. The extracted deep features are then optimized with a Firefly algorithm to select the most optimal learning features. Fusing the selected features is important to improving the accuracy of the approach; however, it directly affects the computational cost of the technique. In the proposed method, a new parallel high index technique is used to fuse two optimal vectors; the outcome is then passed on to an extreme learning machine for final classification. Experiments were conducted on a collected database of patients using a 70:30 training: Testing ratio. Our results indicated an average classification accuracy of 94.76% with the proposed approach. A comparison of the outcomes to several other DL models demonstrated the effectiveness of our DL method for classifying COVID-19 based on CT scans.Keywords
Cite This Article
Citations
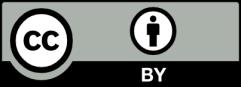