Open Access
ARTICLE
Machine Learning Empowered Security Management and Quality of Service Provision in SDN-NFV Environment
1 Department of Computer Sciences, Kinnaird College for Women, Lahore, 54000, Pakistan
2 College of Computer and Information Sciences, Jouf University, Sakaka, 72341, Saudi Arabia
3 Division of Computer Science & Information Technology, University of Education, Lahore, 54000, Pakistan
* Corresponding Author: Fahad Ahmad. Email:
Computers, Materials & Continua 2021, 66(3), 2723-2749. https://doi.org/10.32604/cmc.2021.014594
Received 01 October 2020; Accepted 18 October 2020; Issue published 28 December 2020
Abstract
With the rising demand for data access, network service providers face the challenge of growing their capital and operating costs while at the same time enhancing network capacity and meeting the increased demand for access. To increase efficacy of Software Defined Network (SDN) and Network Function Virtualization (NFV) framework, we need to eradicate network security configuration errors that may create vulnerabilities to affect overall efficiency, reduce network performance, and increase maintenance cost. The existing frameworks lack in security, and computer systems face few abnormalities, which prompts the need for different recognition and mitigation methods to keep the system in the operational state proactively. The fundamental concept behind SDN-NFV is the encroachment from specific resource execution to the programming-based structure. This research is around the combination of SDN and NFV for rational decision making to control and monitor traffic in the virtualized environment. The combination is often seen as an extra burden in terms of resources usage in a heterogeneous network environment, but as well as it provides the solution for critical problems specially regarding massive network traffic issues. The attacks have been expanding step by step; therefore, it is hard to recognize and protect by conventional methods. To overcome these issues, there must be an autonomous system to recognize and characterize the network traffic’s abnormal conduct if there is any. Only four types of assaults, including HTTP Flood, UDP Flood, Smurf Flood, and SiDDoS Flood, are considered in the identified dataset, to optimize the stability of the SDN-NFV environment and security management, through several machine learning based characterization techniques like Support Vector Machine (SVM), K-Nearest Neighbors (KNN), Logistic Regression (LR) and Isolation Forest (IF). Python is used for simulation purposes, including several valuable utilities like the mine package, the open-source Python ML libraries Scikit-learn, NumPy, SciPy, Matplotlib. Few Flood assaults and Structured Query Language (SQL) injections anomalies are validated and effectively-identified through the anticipated procedure. The classification results are promising and show that overall accuracy lies between 87% to 95% for SVM, LR, KNN, and IF classifiers in the scrutiny of traffic, whether the network traffic is normal or anomalous in the SDN-NFV environment.Keywords
Cite This Article
Citations
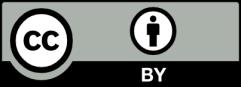
This work is licensed under a Creative Commons Attribution 4.0 International License , which permits unrestricted use, distribution, and reproduction in any medium, provided the original work is properly cited.