Open Access
ARTICLE
A New Optimized Wrapper Gene Selection Method for Breast Cancer Prediction
Department of Information Technology, College of Computer and Information Sciences, King Saud University, Riyadh, 11543, Saudi Arabia
* Corresponding Author: Heyam H. Al-Baity. Email:
(This article belongs to the Special Issue: AI, IoT, Blockchain Assisted Intelligent Solutions to Medical and Healthcare Systems)
Computers, Materials & Continua 2021, 67(3), 3089-3106. https://doi.org/10.32604/cmc.2021.015291
Received 14 November 2020; Accepted 02 January 2021; Issue published 01 March 2021
Abstract
Machine-learning algorithms have been widely used in breast cancer diagnosis to help pathologists and physicians in the decision-making process. However, the high dimensionality of genetic data makes the classification process a challenging task. In this paper, we propose a new optimized wrapper gene selection method that is based on a nature-inspired algorithm (simulated annealing (SA)), which will help select the most informative genes for breast cancer prediction. These optimal genes will then be used to train the classifier to improve its accuracy and efficiency. Three supervised machine-learning algorithms, namely, the support vector machine, the decision tree, and the random forest were used to create the classifier models that will help to predict breast cancer. Two different experiments were conducted using three datasets: Gene expression (GE), deoxyribonucleic acid (DNA) methylation, and a combination of the two. Six measures were used to evaluate the performance of the proposed algorithm, which include the following: Accuracy, precision, recall, specificity, area under the curve (AUC), and execution time. The effectiveness of the proposed classifiers was evaluated through comprehensive experiments. The results demonstrated that our approach outperformed the conventional classifiers as expected in terms of accuracy and execution time. High accuracy values of 99.77%, 99.45%, and 99.45% have been achieved by SA-SVM for GE, DNA methylation, and the combined datasets, respectively. The execution time of the proposed approach was significantly reduced, in comparison to that of the traditional classifiers and the best execution time has been reached by SA-SVM, which was 0.02, 0.03, and 0.02 on GE, DNA methylation, and the combined datasets respectively. In regard to precision and specificity, SA-RF obtained the best result of 100 on GE dataset. While SA-SVM attained the best recall result of 100 on GE dataset.Keywords
Cite This Article
Citations
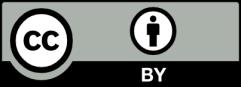
This work is licensed under a Creative Commons Attribution 4.0 International License , which permits unrestricted use, distribution, and reproduction in any medium, provided the original work is properly cited.