Open Access
ARTICLE
Hybrid Trainable System for Writer Identification of Arabic Handwriting
Department of Information Technology, Technical Informatics College of Akre, Duhok Polytechnic University, Duhok, 42004, Iraq
* Corresponding Author: Saleem Ibraheem Saleem. Email:
(This article belongs to the Special Issue: AI, IoT, Blockchain Assisted Intelligent Solutions to Medical and Healthcare Systems)
Computers, Materials & Continua 2021, 68(3), 3353-3372. https://doi.org/10.32604/cmc.2021.016342
Received 30 December 2020; Accepted 22 February 2021; Issue published 06 May 2021
Abstract
Writer identification (WI) based on handwritten text structures is typically focused on digital characteristics, with letters/strokes representing the information acquired from the current research in the integration of individual writing habits/styles. Previous studies have indicated that a word’s attributes contribute to greater recognition than the attributes of a character or stroke. As a result of the complexity of Arabic handwriting, segmenting and separating letters and strokes from a script poses a challenge in addition to WI schemes. In this work, we propose new texture features for WI based on text. The histogram of oriented gradient (HOG) features are modified to extract good features on the basis of the histogram of the orientation for different angles of texts. The fusion of these features with the features of convolutional neural networks (CNNs) results in a good vector of powerful features. Then, we reduce the features by selecting the best ones using a genetic algorithm. The normalization method is used to normalize the features and feed them to an artificial neural network classifier. Experimental results show that the proposed augmenter enhances the results for HOG features and ResNet50, as well as the proposed model, because the amount of data is increased. Such a large data volume helps the system to retrieve extensive information about the nature of writing patterns. The affective result of the proposed model for whole paragraphs, lines, and sub words is obtained using different models and then compared with those of the CNN and ResNet50. The whole paragraphs produce the best results in all models because they contain rich information and the model can utilize numerous features for different words. The HOG and CNN features achieve 94.2% accuracy for whole paragraphs with augmentation, 83.2% of accuracy for lines, and 78% accuracy for sub words. Thus, this work provides a system that can identify writers on the basis of their handwriting and builds a powerful model that can help identify writers on the basis of their sentences, words, and sub words.Keywords
Cite This Article
Citations
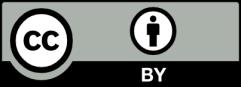
This work is licensed under a Creative Commons Attribution 4.0 International License , which permits unrestricted use, distribution, and reproduction in any medium, provided the original work is properly cited.