Open Access
ARTICLE
Deep Neural Networks Based Approach for Battery Life Prediction
1 School of Information Technology and Engineering, Vellore Institute of Technology, Vellore, India
2 Department of Computer Science and Engineering, Baba Ghulam Shah Badshah University, Rajouri, India
3 Industrial Engineering Department, College of Engineering, King Saud University, Riyadh, Saudi Arabia
4 Raytheon Chair for Systems Engineering, Advanced Manufacturing Institute, King Saud University, Riyadh, Saudi Arabia
* Corresponding Author: Mustufa Haider Abidi. Email:
(This article belongs to the Special Issue: Green IoT Networks using Machine Learning, Deep Learning Models)
Computers, Materials & Continua 2021, 69(2), 2599-2615. https://doi.org/10.32604/cmc.2021.016229
Received 27 December 2020; Accepted 20 February 2021; Issue published 21 July 2021
Abstract
The Internet of Things (IoT) and related applications have witnessed enormous growth since its inception. The diversity of connecting devices and relevant applications have enabled the use of IoT devices in every domain. Although the applicability of these applications are predominant, battery life remains to be a major challenge for IoT devices, wherein unreliability and shortened life would make an IoT application completely useless. In this work, an optimized deep neural networks based model is used to predict the battery life of the IoT systems. The present study uses the Chicago Park Beach dataset collected from the publicly available data repository for the experimentation of the proposed methodology. The dataset is pre-processed using the attribute mean technique eliminating the missing values and then One-Hot encoding technique is implemented to convert it to numerical format. This processed data is normalized using the Standard Scaler technique. Moth Flame Optimization (MFO) Algorithm is then implemented for selecting the optimal features in the dataset. These optimal features are finally fed into the DNN model and the results generated are evaluated against the state-of-the-art models, which justify the superiority of the proposed MFO-DNN model.Keywords
Cite This Article
Citations
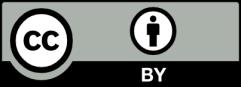
This work is licensed under a Creative Commons Attribution 4.0 International License , which permits unrestricted use, distribution, and reproduction in any medium, provided the original work is properly cited.