Open Access
ARTICLE
An Improved Machine Learning Technique with Effective Heart Disease Prediction System
1 College of Computing and Information Technology, University of Bisha, Bisha, 67714, Saudi Arabia
2 King Abdulaziz City for Science and Technology, P.O. Box 6086, Riyadh, 11442, Saudi Arabia
3 College of Computer Science and Information Systems, Najran University, Najran, 61441, Saudi Arabia
4 College of Engineering, Muzahimiyah Branch, King Saud University, Riyadh, 11451, Saudi Arabia
5 Department of Electrical Engineering, Laboratory for Analysis, Conception and Control of Systems, LR-11-ES20, National Engineering School of Tunis, Tunis El Manar University, 1002, Tunisia
* Corresponding Author: Saad Alhuwaimel. Email:
(This article belongs to the Special Issue: AI 2.0-Enabled Next Generation Intelligence of Things for Smart Enterprise Systems)
Computers, Materials & Continua 2021, 69(3), 4169-4181. https://doi.org/10.32604/cmc.2021.015984
Received 17 December 2020; Accepted 24 April 2021; Issue published 24 August 2021
Abstract
Heart disease is the leading cause of death worldwide. Predicting heart disease is challenging because it requires substantial experience and knowledge. Several research studies have found that the diagnostic accuracy of heart disease is low. The coronary heart disorder determines the state that influences the heart valves, causing heart disease. Two indications of coronary heart disorder are strep throat with a red persistent skin rash, and a sore throat covered by tonsils or strep throat. This work focuses on a hybrid machine learning algorithm that helps predict heart attacks and arterial stiffness. At first, we achieved the component perception measured by using a hybrid cuckoo search particle swarm optimization (CSPSO) algorithm. With this perception measure, characterization and accuracy were improved, while the execution time of the proposed model was decreased. The CSPSO-deep recurrent neural network algorithm resolved issues that state-of-the-art methods face. Our proposed method offers an illustrative framework that helps predict heart attacks with high accuracy. The proposed technique demonstrates the model accuracy, which reached 0.97 with the applied dataset.Keywords
Cite This Article
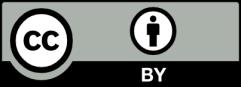