Open Access
ARTICLE
Medical Feature Selection Approach Based on Generalized Normal Distribution Algorithm
1 Faculty of Computers and Informatics, Zagazig University, Zagazig, 44519, Egypt
2 Capability Systems Centre, School of Engineering and IT, UNSW, Canberra, Australia
3 Department of Computer Science and Engineering, Soonchunhyang University, Asan, 31538, Korea
4 Department of Mathematics, Faculty of Science, Mansoura University, Mansoura, 35516, Egypt
5 Department of Computational Mathematics, Science, and Engineering (CMSE), Michigan State University, East Lansing, 48824, MI, USA
* Corresponding Author: Yunyoung Nam. Email:
Computers, Materials & Continua 2021, 69(3), 2883-2901. https://doi.org/10.32604/cmc.2021.017854
Received 12 February 2021; Accepted 16 March 2021; Issue published 24 August 2021
Abstract
This paper proposes a new pre-processing technique to separate the most effective features from those that might deteriorate the performance of the machine learning classifiers in terms of computational costs and classification accuracy because of their irrelevance, redundancy, or less information; this pre-processing process is often known as feature selection. This technique is based on adopting a new optimization algorithm known as generalized normal distribution optimization (GNDO) supported by the conversion of the normal distribution to a binary one using the arctangent transfer function to convert the continuous values into binary values. Further, a novel restarting strategy (RS) is proposed to preserve the diversity among the solutions within the population by identifying the solutions that exceed a specific distance from the best-so-far and replace them with the others created using an effective updating scheme. This strategy is integrated with GNDO to propose another binary variant having a high ability to preserve the diversity of the solutions for avoiding becoming stuck in local minima and accelerating convergence, namely improved GNDO (IGNDO). The proposed GNDO and IGNDO algorithms are extensively compared with seven state-of-the-art algorithms to verify their performance on thirteen medical instances taken from the UCI repository. IGNDO is shown to be superior in terms of fitness value and classification accuracy and competitive with the others in terms of the selected features. Since the principal goal in solving the FS problem is to find the appropriate subset of features that maximize classification accuracy, IGNDO is considered the best.Keywords
Cite This Article
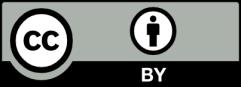