Open Access
ARTICLE
A Netnographic-Based Semantic Analysis of Tweet Contents for Stress Management
1 HAMK Smart Research Unit, Häme University of Applied Sciences, Hämeenlinna, 13100, Finland
2 Department of Computer Science, College of Computers and Information Technology, Taif University, Taif, 21944, Saudi Arabia
3 College of Computer and Information Sciences, Princess Nourah bint Abdulrahman University, Riyadh, 11671, Saudi Arabia
4 Faculty of Management and Business, Tampere University, Tampere, 33720, Finland
5 College of Computing and Information Technologies, University of Bisha, Bisha, 67714, Saudi Arabia
* Corresponding Author: Eman Alkhammash. Email:
(This article belongs to the Special Issue: Machine Learning for Data Analytics)
Computers, Materials & Continua 2022, 70(1), 1845-1856. https://doi.org/10.32604/cmc.2022.017284
Received 26 January 2021; Accepted 19 May 2021; Issue published 07 September 2021
Abstract
Social media platforms provide new value for markets and research companies. This article explores the use of social media data to enhance customer value propositions. The case study involves a company that develops wearable Internet of Things (IoT) devices and services for stress management. Netnography and semantic annotation for recognizing and categorizing the context of tweets are conducted to gain a better understanding of users’ stress management practices. The aim is to analyze the tweets about stress management practices and to identify the context from the tweets. Thereafter, we map the tweets on pleasure and arousal to elicit customer insights. We analyzed a case study of a marketing strategy on the Twitter platform. Participants in the marketing campaign shared photos and texts about their stress management practices. Machine learning techniques were used to evaluate and estimate the emotions and contexts of the tweets posted by the campaign participants. The computational semantic analysis of the tweets was compared to the text analysis of the tweets. The content analysis of only tweet images resulted in 96% accuracy in detecting tweet context, while that of the textual content of tweets yielded an accuracy of 91%. Semantic tagging by Ontotext was able to detect correct tweet context with an accuracy of 50%.Keywords
Cite This Article
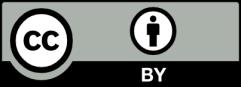