Open Access
ARTICLE
Training Multi-Layer Perceptron with Enhanced Brain Storm Optimization Metaheuristics
1 Singidunum University, Danijelova, 11000, Belgrade, Serbia
2 National Center for Robotics and IoT, Communication and Information Technology Research Institute, King Abdulaziz City for Science and Technology (KACST), Riyadh, 12371, Saudi Arabia
3 Department of Computer Science and Engineering, CHRIST (Deemed to be University), Bangalore, 560074, India
4 Prince Sattam Bin Abdulaziz University, College of Computer Engineering and Sciences, Alkharj, 11942, Saudi Arabia
* Corresponding Author: Khaled Alhazmi. Email:
(This article belongs to the Special Issue: Emerging Applications of Artificial Intelligence, Machine learning and Data Science)
Computers, Materials & Continua 2022, 70(2), 4199-4215. https://doi.org/10.32604/cmc.2022.020449
Received 24 May 2021; Accepted 07 July 2021; Issue published 27 September 2021
Abstract
In the domain of artificial neural networks, the learning process represents one of the most challenging tasks. Since the classification accuracy highly depends on the weights and biases, it is crucial to find its optimal or suboptimal values for the problem at hand. However, to a very large search space, it is very difficult to find the proper values of connection weights and biases. Employing traditional optimization algorithms for this issue leads to slow convergence and it is prone to get stuck in the local optima. Most commonly, back-propagation is used for multi-layer-perceptron training and it can lead to vanishing gradient issue. As an alternative approach, stochastic optimization algorithms, such as nature-inspired metaheuristics are more reliable for complex optimization tax, such as finding the proper values of weights and biases for neural network training. In this work, we propose an enhanced brain storm optimization-based algorithm for training neural networks. In the simulations, ten binary classification benchmark datasets with different difficulty levels are used to evaluate the efficiency of the proposed enhanced brain storm optimization algorithm. The results show that the proposed approach is very promising in this domain and it achieved better results than other state-of-the-art approaches on the majority of datasets in terms of classification accuracy and convergence speed, due to the capability of balancing the intensification and diversification and avoiding the local minima. The proposed approach obtained the best accuracy on eight out of ten observed dataset, outperforming all other algorithms by 1–2% on average. When mean accuracy is observed, the proposed algorithm dominated on nine out of ten datasets.Keywords
Cite This Article
Citations
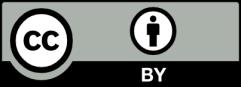