Open Access
ARTICLE
A Deep Learning-Based Continuous Blood Pressure Measurement by Dual Photoplethysmography Signals
1 Department of Electrical Engineering, National Taiwan Ocean University, Keelung, 202301, Taiwan
2 Department of Internal Medicine, National Taiwan University, Yun-Lin Branch, Dou-Liu, 640, Taiwan
3 Department of Electrical Engineering, National Formosa University, Yunlin, 632, Taiwan
* Corresponding Author: Chih-Ta Yen. Email:
Computers, Materials & Continua 2022, 70(2), 2937-2952. https://doi.org/10.32604/cmc.2022.020493
Received 26 May 2021; Accepted 27 June 2021; Issue published 27 September 2021
Abstract
This study proposed a measurement platform for continuous blood pressure estimation based on dual photoplethysmography (PPG) sensors and a deep learning (DL) that can be used for continuous and rapid measurement of blood pressure and analysis of cardiovascular-related indicators. The proposed platform measured the signal changes in PPG and converted them into physiological indicators, such as pulse transit time (PTT), pulse wave velocity (PWV), perfusion index (PI) and heart rate (HR); these indicators were then fed into the DL to calculate blood pressure. The hardware of the experiment comprised 2 PPG components (i.e., Raspberry Pi 3 Model B and analog-to-digital converter [MCP3008]), which were connected using a serial peripheral interface. The DL algorithm converted the stable dual PPG signals acquired from the strictly standardized experimental process into various physiological indicators as input parameters and finally obtained the systolic blood pressure (SBP), diastolic blood pressure (DBP) and mean arterial pressure (MAP). To increase the robustness of the DL model, this study input data of 100 Asian participants into the training database, including those with and without cardiovascular disease, each with a proportion of approximately 50%. The experimental results revealed that the mean absolute error and standard deviation of SBP was 0.17 ± 0.46 mmHg. The mean absolute error and standard deviation of DBP was 0.27 ± 0.52 mmHg. The mean absolute error and standard deviation of MAP was 0.16 ± 0.40 mmHg.Keywords
Cite This Article
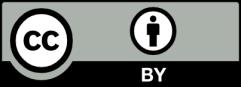