Open Access
ARTICLE
Fuzzy-Based Automatic Epileptic Seizure Detection Framework
1 Shaheed Zulfikar Ali Bhutto Institute of Science and Technology, Islamabad, Pakistan
2 Department of Computer Science & IT, University of Lakki Marwat, KPK, Pakistan
3 Stockholm University, Stockholm, Sweden
4 Department of Computer Science, School of Arts and Sciences, University of Central Asia, Kyrgyz Republic
5 Department of Software, Korea National University of Transportation, Chungju, 27469, Korea
6 Department of Biomedical Engineering, Korea National University of Transportation, Chungju, 27469, Korea
7 Department of AI Robotics Engineering, Korea National University of Transportation, Chungju, 27469, Korea
8 Department of IT & Energy Convergence (BK21 FOUR), Korea National University of Transportation, Chungju, 27469, Korea
* Corresponding Author: Jeonghwan Gwak. Email:
(This article belongs to the Special Issue: Advancements in Lightweight AI for Constrained Internet of Things Devices for Smart Cities)
Computers, Materials & Continua 2022, 70(3), 5601-5630. https://doi.org/10.32604/cmc.2022.020348
Received 20 May 2021; Accepted 16 July 2021; Issue published 11 October 2021
Abstract
Detection of epileptic seizures on the basis of Electroencephalogram (EEG) recordings is a challenging task due to the complex, non-stationary and non-linear nature of these biomedical signals. In the existing literature, a number of automatic epileptic seizure detection methods have been proposed that extract useful features from EEG segments and classify them using machine learning algorithms. Some characterizing features of epileptic and non-epileptic EEG signals overlap; therefore, it requires that analysis of signals must be performed from diverse perspectives. Few studies analyzed these signals in diverse domains to identify distinguishing characteristics of epileptic EEG signals. To pose the challenge mentioned above, in this paper, a fuzzy-based epileptic seizure detection model is proposed that incorporates a novel feature extraction and selection method along with fuzzy classifiers. The proposed work extracts pattern features along with time-domain, frequency-domain, and non-linear analysis of signals. It applies a feature selection strategy on extracted features to get more discriminating features that build fuzzy machine learning classifiers for the detection of epileptic seizures. The empirical evaluation of the proposed model was conducted on the benchmark Bonn EEG dataset. It shows significant accuracy of 98% to 100% for normal vs. ictal classification cases while for three class classification of normal vs. inter-ictal vs. ictal accuracy reaches to above 97.5%. The obtained results for ten classification cases (including normal, seizure or ictal, and seizure-free or inter-ictal classes) prove the superior performance of proposed work as compared to other state-of-the-art counterparts.Keywords
Cite This Article
Citations
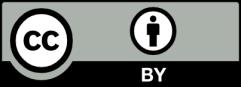