Open Access
ARTICLE
Benchmarking Performance of Document Level Classification and Topic Modeling
1 Department of Computer Science, COMSATS University Islamabad, Lahore, Pakistan
2 Department of Communication Technology and Network, Faculty of Computer Science, Universiti Putra Malaysia, Selangor, 43400, Malaysia
3 Othman Yeop Abdullah Graduate School of Business (OYAGSB), Universiti Utara Malaysia (UUM), Kuala Lumpur, 50300, Malaysia
* Corresponding Author: Muhammad Shahid Bhatti. Email:
(This article belongs to the Special Issue: Computational Models for Pro-Smart Environments in Data Science Assisted IoT Systems)
Computers, Materials & Continua 2022, 71(1), 125-141. https://doi.org/10.32604/cmc.2022.020083
Received 08 May 2021; Accepted 18 June 2021; Issue published 03 November 2021
Abstract
Text classification of low resource language is always a trivial and challenging problem. This paper discusses the process of Urdu news classification and Urdu documents similarity. Urdu is one of the most famous spoken languages in Asia. The implementation of computational methodologies for text classification has increased over time. However, Urdu language has not much experimented with research, it does not have readily available datasets, which turn out to be the primary reason behind limited research and applying the latest methodologies to the Urdu. To overcome these obstacles, a medium-sized dataset having six categories is collected from authentic Pakistani news sources. Urdu is a rich but complex language. Text processing can be challenging for Urdu due to its complex features as compared to other languages. Term frequency-inverse document frequency (TFIDF) based term weighting scheme for extracting features, chi-2 for selecting essential features, and Linear discriminant analysis (LDA) for dimensionality reduction have been used. TFIDF matrix and cosine similarity measure have been used to identify similar documents in a collection and find the semantic meaning of words in a document FastText model has been applied. The training-test split evaluation methodology is used for this experimentation, which includes 70% for training data and 30% for testing data. State-of-the-art machine learning and deep dense neural network approaches for Urdu news classification have been used. Finally, we trained Multinomial Naïve Bayes, XGBoost, Bagging, and Deep dense neural network. Bagging and deep dense neural network outperformed the other algorithms. The experimental results show that deep dense achieves 92.0% mean f1 score, and Bagging 95.0% f1 score.Keywords
Cite This Article
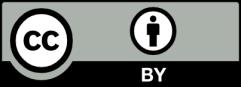