Open Access
ARTICLE
Deep Reinforcement Learning for Addressing Disruptions in Traffic Light Control
1 School of Engineering and Computer Science, University of Hertfordshire, Hatfield, AL109AB, UK
2 Department of Computing and Information Systems, Sunway University, Subang Jaya, 47500, Malaysia
3 Faculty of Computer Science and Information Technology, University of Malaya, Kuala Lumpur, 50603, Malaysia
4 National Advanced IPv6 Centre, Universiti Sains Malaysia, USM, Penang, 11800, Malaysia
* Corresponding Author: Yung-Wey Chong. Email:
(This article belongs to the Special Issue: Artificial Intelligence Enabled Intelligent Transportation Systems)
Computers, Materials & Continua 2022, 71(2), 2225-2247. https://doi.org/10.32604/cmc.2022.022952
Received 24 August 2021; Accepted 27 September 2021; Issue published 07 December 2021
Abstract
This paper investigates the use of multi-agent deep Q-network (MADQN) to address the curse of dimensionality issue occurred in the traditional multi-agent reinforcement learning (MARL) approach. The proposed MADQN is applied to traffic light controllers at multiple intersections with busy traffic and traffic disruptions, particularly rainfall. MADQN is based on deep Q-network (DQN), which is an integration of the traditional reinforcement learning (RL) and the newly emerging deep learning (DL) approaches. MADQN enables traffic light controllers to learn, exchange knowledge with neighboring agents, and select optimal joint actions in a collaborative manner. A case study based on a real traffic network is conducted as part of a sustainable urban city project in the Sunway City of Kuala Lumpur in Malaysia. Investigation is also performed using a grid traffic network (GTN) to understand that the proposed scheme is effective in a traditional traffic network. Our proposed scheme is evaluated using two simulation tools, namely Matlab and Simulation of Urban Mobility (SUMO). Our proposed scheme has shown that the cumulative delay of vehicles can be reduced by up to 30% in the simulations.Keywords
Cite This Article
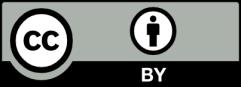