Open Access
ARTICLE
Machine Learning-based Optimal Framework for Internet of Things Networks
1 Department of Computer Engineering, College of Engineering, Al-Hussein Bin Talal University, Ma'an, Jordan
2 Abdul Aziz Ghurair School of Advanced Computing (ASAC), LTUC, Amman, P11118, Jordan
3 Department of Electrical Engineering, Engineering Faculty, The Hashemite University, Zarqa, 13133, Jordan
* Corresponding Author: Moath Alsafasfeh. Email:
Computers, Materials & Continua 2022, 71(3), 5355-5380. https://doi.org/10.32604/cmc.2022.024093
Received 03 October 2021; Accepted 16 November 2021; Issue published 14 January 2022
Abstract
Deep neural networks (DNN) are widely employed in a wide range of intelligent applications, including image and video recognition. However, due to the enormous amount of computations required by DNN. Therefore, performing DNN inference tasks locally is problematic for resource-constrained Internet of Things (IoT) devices. Existing cloud approaches are sensitive to problems like erratic communication delays and unreliable remote server performance. The utilization of IoT device collaboration to create distributed and scalable DNN task inference is a very promising strategy. The existing research, on the other hand, exclusively looks at the static split method in the scenario of homogeneous IoT devices. As a result, there is a pressing need to investigate how to divide DNN tasks adaptively among IoT devices with varying capabilities and resource constraints, and execute the task inference cooperatively. Two major obstacles confront the aforementioned research problems: 1) In a heterogeneous dynamic multi-device environment, it is difficult to estimate the multi-layer inference delay of DNN tasks; 2) It is difficult to intelligently adapt the collaborative inference approach in real time. As a result, a multi-layer delay prediction model with fine-grained interpretability is proposed initially. Furthermore, for DNN inference tasks, evolutionary reinforcement learning (ERL) is employed to adaptively discover the approximate best split strategy. Experiments show that, in a heterogeneous dynamic environment, the proposed framework can provide considerable DNN inference acceleration. When the number of devices is 2, 3, and 4, the delay acceleration of the proposed algorithm is 1.81 times, 1.98 times and 5.28 times that of the EE algorithm, respectively.Keywords
Cite This Article
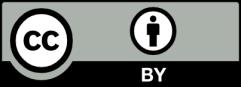