Open Access
ARTICLE
Importance of Adaptive Photometric Augmentation for Different Convolutional Neural Network
1 AI Reliability Lab, ThinkforBL Consultancy Services, Gangnam, Seoul, Korea
2 Department of Biosystems Engineering, Chungbuk National University, Cheongju-si, Chungcheongbuk-do, 28644, Korea
* Corresponding Author: Ji Hwan Park. Email:
Computers, Materials & Continua 2022, 72(3), 4433-4452. https://doi.org/10.32604/cmc.2022.026759
Received 04 January 2022; Accepted 23 February 2022; Issue published 21 April 2022
Abstract
Existing segmentation and augmentation techniques on convolutional neural network (CNN) has produced remarkable progress in object detection. However, the nominal accuracy and performance might be downturned with the photometric variation of images that are directly ignored in the training process, along with the context of the individual CNN algorithm. In this paper, we investigate the effect of a photometric variation like brightness and sharpness on different CNN. We observe that random augmentation of images weakens the performance unless the augmentation combines the weak limits of photometric variation. Our approach has been justified by the experimental result obtained from the PASCAL VOC 2007 dataset, with object detection CNN algorithms such as YOLOv3 (You Only Look Once), Faster R-CNN (Region-based CNN), and SSD (Single Shot Multibox Detector). Each CNN model shows performance loss for varying sharpness and brightness, ranging between −80% to 80%. It was further shown that compared to random augmentation, the augmented dataset with weak photometric changes delivered high performance, but the photometric augmentation range differs for each model. Concurrently, we discuss some research questions that benefit the direction of the study. The results prove the importance of adaptive augmentation for individual CNN model, subjecting towards the robustness of object detection.Keywords
Cite This Article
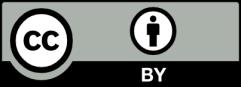