Open Access
ARTICLE
On-line Recognition of Abnormal Patterns in Bivariate Autocorrelated Process Using Random Forest
1 College of Mechanical and Electrical Engineering, Kunming University of Science & Technology, Kunming, 650500, China
2 School of Engineering, Cardiff University, Cardiff, CF24 3AA, UK
* Corresponding Author: Bo Zhu. Email:
Computers, Materials & Continua 2022, 73(1), 1707-1722. https://doi.org/10.32604/cmc.2022.027708
Received 24 January 2022; Accepted 08 March 2022; Issue published 18 May 2022
Abstract
It is not uncommon that two or more related process quality characteristics are needed to be monitored simultaneously in production process for most of time. Meanwhile, the observations obtained online are often serially autocorrelated due to high sampling frequency and process dynamics. This goes against the statistical I.I.D assumption in using the multivariate control charts, which may lead to the performance of multivariate control charts collapse soon. Meanwhile, the process control method based on pattern recognition as a non-statistical approach is not confined by this limitation, and further provide more useful information for quality practitioners to locate the assignable causes led to process abnormalities. This study proposed a pattern recognition model using Random Forest (RF) as pattern model to detect and identify the abnormalities in bivariate autocorrelated process. The simulation experiment results demonstrate that the model is superior on recognition accuracy (RA) (97.96%) to back propagation neural networks (BPNN) (95.69%), probability neural networks (PNN) (94.31%), and support vector machine (SVM) (97.16%). When experimenting with simulated dynamic process data flow, the model also achieved better average running length (ARL) and standard deviation of ARL (SRL) than those of the four comparative approaches in most cases of mean shift magnitude. Therefore, we get the conclusion that the RF model is a promising approach for detecting abnormalities in the bivariate autocorrelated process. Although bivariate autocorrelated process is focused in this study, the proposed model can be extended to multivariate autocorrelated process control.Keywords
Cite This Article
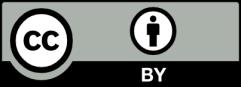